Simple explanation of A/B Testing
TLDRThe video script discusses A/B testing, a technique used to determine the effectiveness of two different versions of a webpage or product feature. It uses the example of AtliQ Technologies, a software company, to illustrate how moving a contact button's position can impact sales leads. The script emphasizes the importance of random distribution of traffic to avoid sampling bias and the need for sufficient samples to ensure statistical significance. It also mentions the widespread use of A/B testing in industries, including Amazon's use of it for product recommendations and tab design, and its application in social media ad campaigns. The simplicity and power of A/B testing make it a popular choice over more complex multi-version testing.
Takeaways
- π A/B testing is a technique used to determine which of two options performs better.
- π― AtliQ Technologies used A/B testing to decide whether moving a contact button to the middle of their website would generate more sales leads.
- π The company ran parallel versions of their website for 10 days, dividing traffic equally between the two versions to gather data.
- π After the test period, version B with the contact button in the middle generated more sales leads, indicating its effectiveness.
- βοΈ It's important to consider potential factors like sampling bias and ensure a random distribution of users across different versions.
- π A sufficient sample size is crucial for the validity of A/B testing; a short experiment with few users may not yield reliable results.
- π A/B testing is widely used across various industries, including website design, data science, and machine learning.
- π οΈ Amazon uses A/B testing to refine their product category tabs and other aspects of their user interface.
- π€ In machine learning, A/B testing helps evaluate the performance of new models before full deployment.
- π A/B testing can also be applied to advertising campaigns, such as social media ads, to determine the most effective strategies.
- π While A/B/C or A/B/C/D testing is possible, A/B testing is preferred for its simplicity and ease of interpretation.
Q & A
What is A/B testing and why is it used?
-A/B testing is a technique used to compare two versions of a webpage, product, or marketing campaign to determine which one performs better. It's used to make data-driven decisions by measuring the performance of different variations against each other.
How does AtliQ Technologies use A/B testing to improve their website?
-AtliQ Technologies uses A/B testing to determine the effectiveness of moving their contact button from the top right to the middle of the webpage. They run two versions of the website in parallel, with equal traffic, and compare the number of sales leads generated to decide which version is better.
What are the key factors to consider when conducting A/B testing?
-When conducting A/B testing, it's important to consider factors such as sampling bias, random distribution of traffic, sufficient sample size, and statistical significance to ensure the results are reliable and not due to random chance.
What is sampling bias in the context of A/B testing?
-Sampling bias occurs when the users visiting one version of the test are from a specific area or demographic that may skew the results. To avoid this, the traffic should be randomly distributed so that participants in both versions A and B are representative of the overall audience.
Why is it important to have a sufficient sample size in A/B testing?
-A sufficient sample size ensures that the results of the A/B test are statistically significant and not influenced by random fluctuations. A small sample size might lead to conclusions that are not representative of the entire user base.
How does A/B testing relate to data science and machine learning?
-A/B testing is heavily used in data science and machine learning to validate and improve models and algorithms. For example, it can be used to test the effectiveness of a new machine learning model for product recommendations before fully deploying it.
Can A/B testing be expanded beyond just two versions?
-Yes, A/B testing can be expanded to include more than two versions, known as A/B/C or A/B/C/D testing. However, two-version testing is more popular because it simplifies the experimental design and makes it easier to interpret the results.
What is an example of A/B testing in e-commerce?
-Amazon uses A/B testing to design their product category tabs. They test different layouts and features to determine which version leads to better user engagement and sales.
How is A/B testing applied in social media ad campaigns?
-In social media ad campaigns, A/B testing can be used to compare different ad layouts, demographics, and budgets to determine which campaign performs better. The insights gained can then be used to allocate advertising funds more effectively.
What does statistical significance mean in A/B testing?
-Statistical significance means that the results of the A/B test are unlikely to have occurred by chance. It's a measure that helps determine if the difference in performance between the two versions is real and not just a random occurrence.
How does A/B testing help in decision-making for businesses?
-A/B testing provides businesses with empirical evidence to make informed decisions. By comparing different versions, businesses can identify the most effective strategies, layouts, or messaging that resonate with their audience, leading to improved performance and outcomes.
Outlines
π§ A/B Testing: The Technique for Decision-Making
This paragraph introduces A/B testing as a method to determine the better-performing option between two variants. It uses AtliQ Technologies as a case study, where the company tests the placement of a contact button on their website to generate more sales leads. The paragraph explains the process of running two parallel versions of the website for a set period, dividing the traffic equally between them, and comparing the results to conclude which version is superior. It also touches on the importance of avoiding sampling bias and ensuring a sufficient sample size for reliable conclusions. The paragraph concludes by mentioning that A/B testing is widely used in various industries, including website layouts, data science, and machine learning, with a specific example of Amazon's product recommendation system.
π Applying A/B Testing in Marketing Campaigns
The second paragraph discusses the application of A/B testing in marketing, particularly in social media ad campaigns. It provides an example of allocating a budget to test different ad layouts and demographic configurations to determine the most effective campaign. The paragraph emphasizes the practicality of A/B testing in making informed decisions on where to invest advertising funds based on the performance of the tested campaigns. It concludes by highlighting the power and utility of A/B testing in optimizing marketing strategies and invites viewers to share their questions in the comments section.
Mindmap
Keywords
π‘A/B Testing
π‘Sampling Bias
π‘Statistical Significance
π‘Hypothesis Testing
π‘Sales Leads
π‘Web Layout Changes
π‘Data Science
π‘Machine Learning
π‘Product Recommendations
π‘Social Media Ad Campaigns
π‘Conversion Rates
Highlights
A/B testing is a technique used to determine which of two options performs better.
AtliQ Technologies, a software company, uses A/B testing to optimize their website's contact button placement.
The business manager at AtliQ suggests moving the contact button to the middle of the website to potentially increase sales leads.
A/B testing involves creating an alternate version of a website or product and running both versions in parallel to compare performance.
Traffic is divided equally between the two versions of the website to ensure a fair comparison.
Sales leads generated by each version are compared over a set period to determine which is more effective.
Sampling bias must be considered to ensure that the user demographics visiting each version are representative and not skewed.
Adequate samples are crucial for the validity of A/B testing; not enough samples can lead to unreliable conclusions.
Statistical significance is an important factor in A/B testing to ensure that conclusions are not based on random chance.
A/B testing is widely used in website design and layout changes, such as Amazon's product category tabs.
Data science and machine learning heavily rely on A/B testing for product recommendations and algorithm deployment.
A/B testing is also applicable in advertising campaigns, such as Facebook ad campaigns, to determine the most effective strategy.
The simplicity of A/B testing, compared to A/C or A/B/C/D testing, makes it a popular choice for comparing two options.
A/B testing helps businesses make data-driven decisions rather than relying solely on intuition.
The process of A/B testing involves hypothesis testing to validate the results and ensure they are not due to random variation.
A/B testing is a powerful technique that is used throughout the industry for various applications, from website design to social media campaigns.
The video provides a link to a research paper demonstrating Amazon's use of A/B testing in designing their product category tabs.
The video aims to educate viewers on the importance and application of A/B testing in a clear and engaging manner.
Transcripts
Browse More Related Video
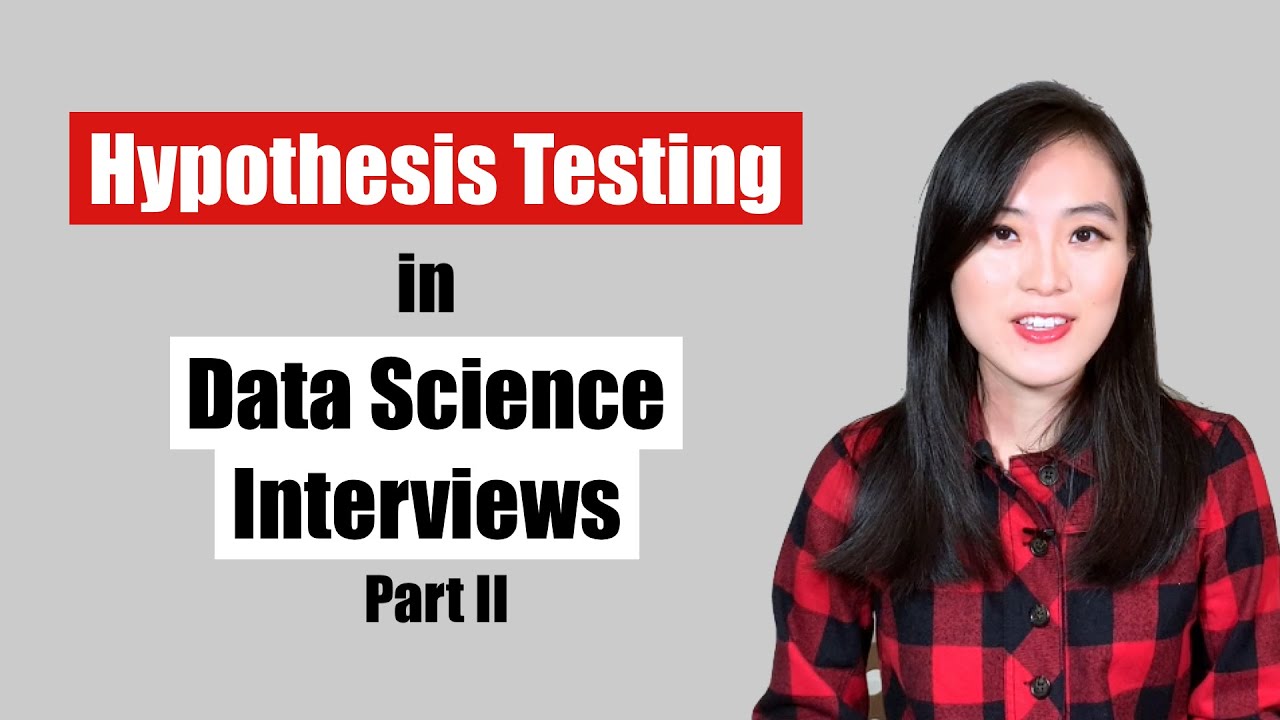
A/B Testing Analysis Made Easy: How to Use Hypothesis Testing for Data Science Interviews!
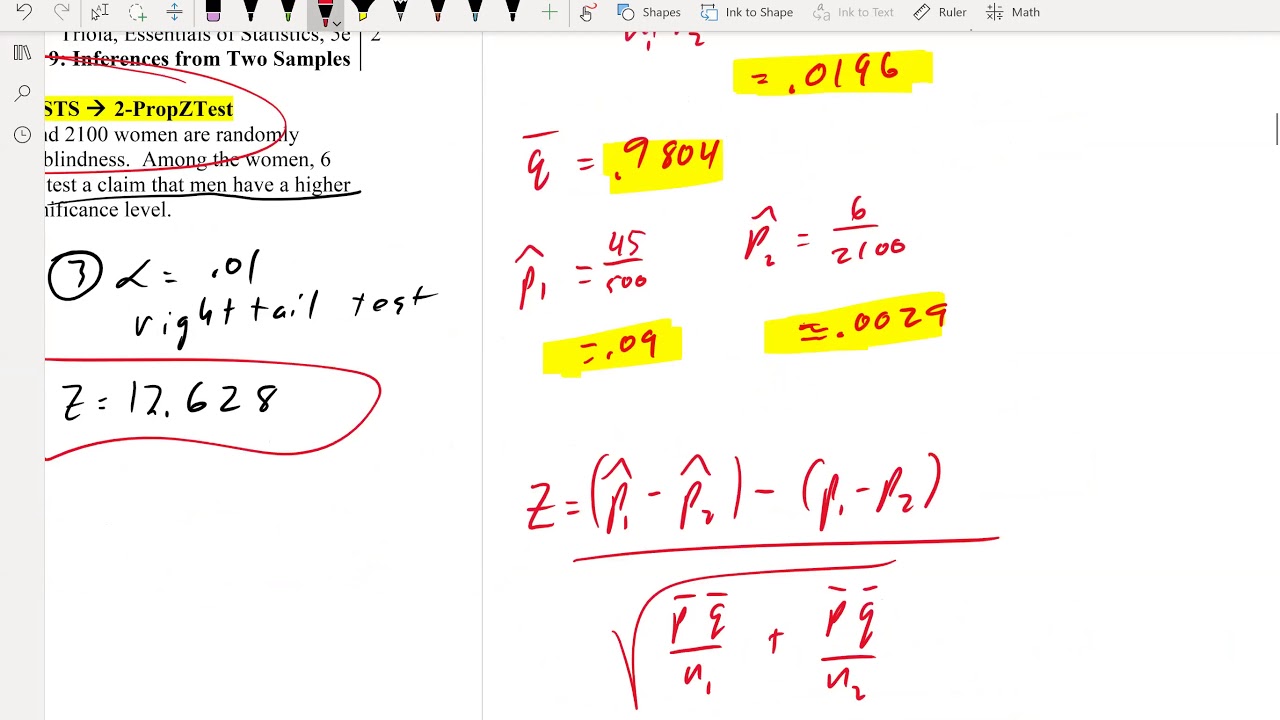
Math 119 Chapter 9 part 1
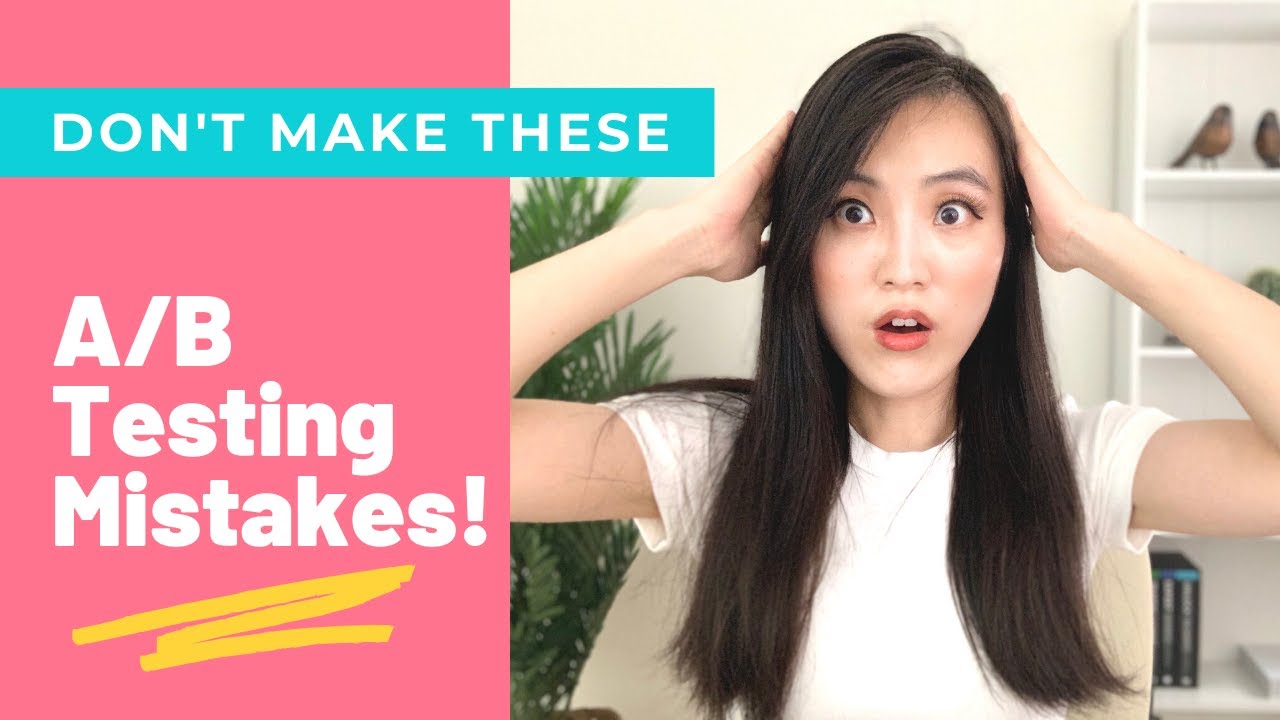
A/B Testing Mistakes to Avoid in Your Data Science Interview: Tips and Tricks!
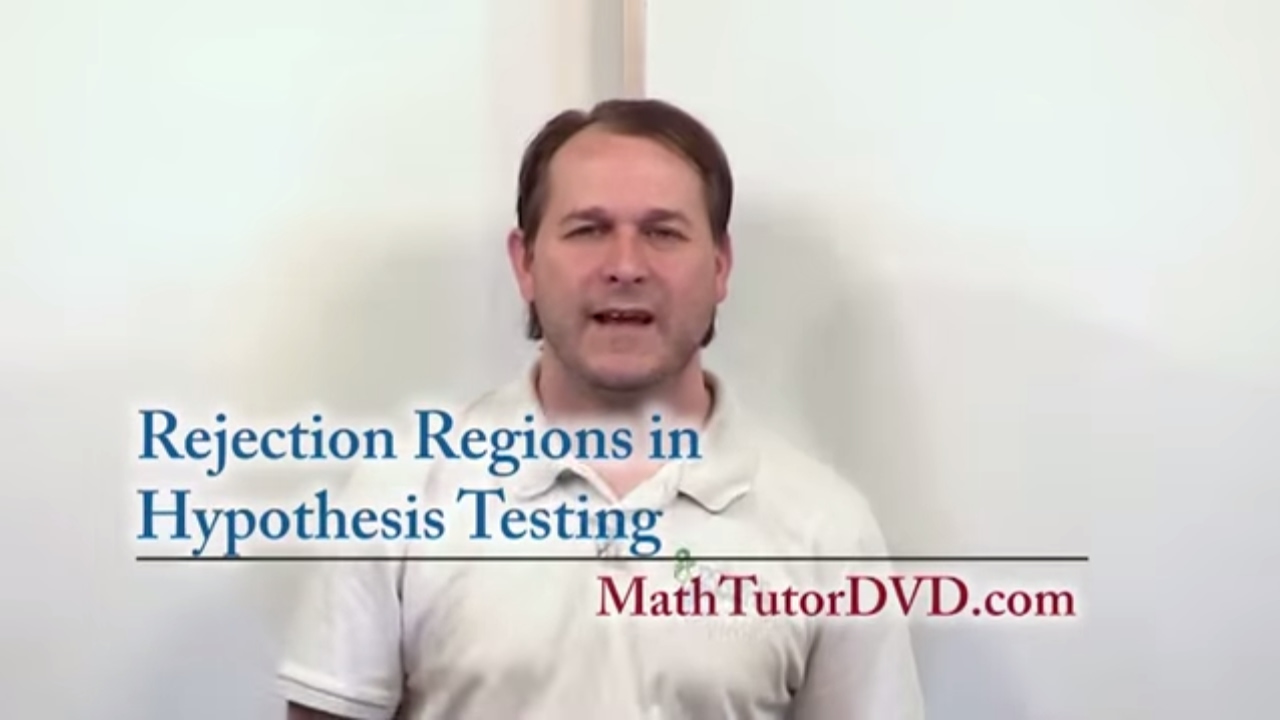
Lesson 10 - Rejection Regions In Hypothesis Testing (Statistics Tutor)
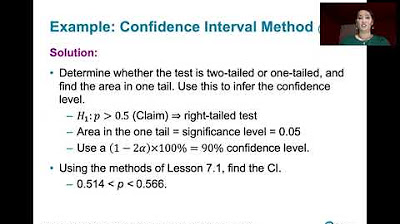
8.2.2 Testing a Claim About A Proportion - Confidence Interval Method, Comparison to Other Methods
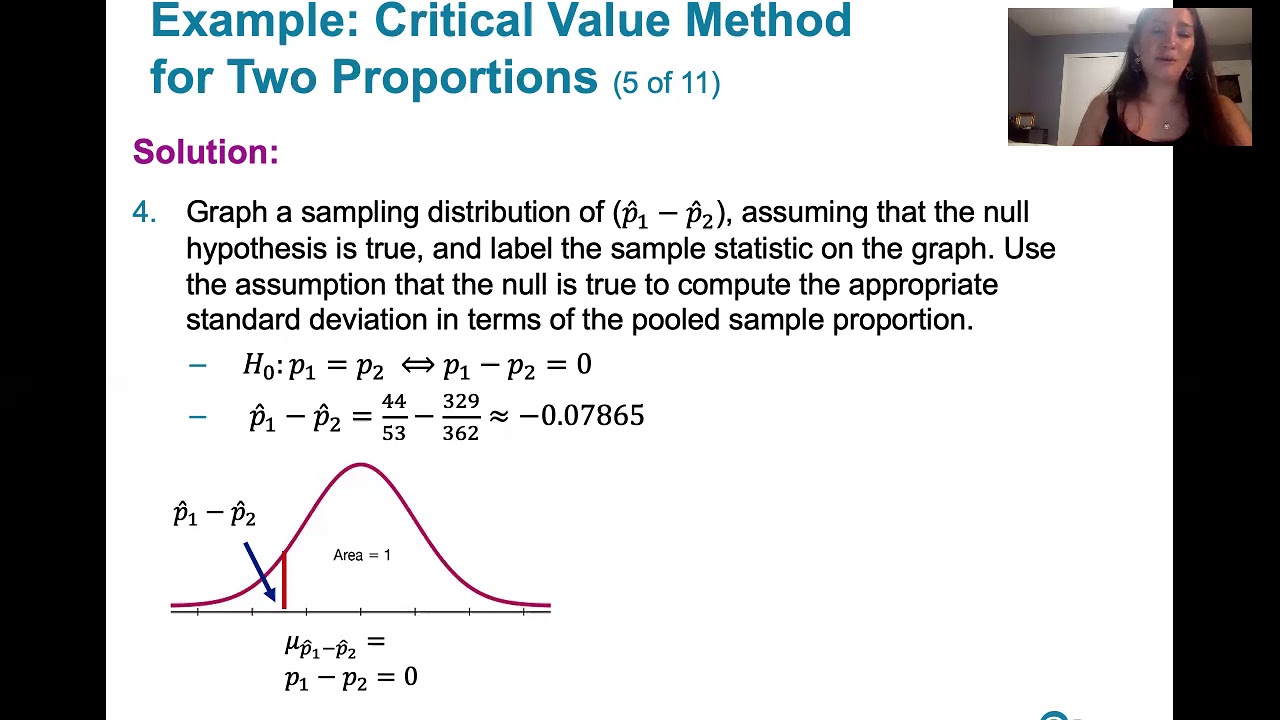
9.1.3 Two Proportions - Example, Critical Value Method, Normal Approximation
5.0 / 5 (0 votes)
Thanks for rating: