Wilcoxon Signed Rank Test | Statistics Tutorial #22 | MarinStatsLectures
TLDRThis video script introduces the Wilcoxon Signed-Rank Test, a nonparametric method for comparing paired numeric values, such as pre- and post-treatment measurements. It serves as an alternative to the paired t-test, suitable for small sample sizes and situations where normality cannot be assumed. The script explains the concept of nonparametric tests, starting with the simple Sign Test and progressing to the more comprehensive Wilcoxon test, which considers both the sign and magnitude of differences. It emphasizes the importance of understanding the underlying concepts rather than manual calculations, highlighting the trade-offs between nonparametric and parametric approaches.
Takeaways
- π The Wilcoxon Signed-Rank Test is a nonparametric alternative to the paired t-test, used for comparing two related groups on a numeric variable.
- π It is particularly useful for small sample sizes or when the data does not meet the assumptions of normality required for parametric tests.
- π The test focuses on the median change rather than the mean change, which is a key difference from parametric tests.
- π Nonparametric tests like the Wilcoxon Signed-Rank Test have lower statistical power compared to parametric tests, meaning they are less likely to detect an effect when one exists.
- π€ The concept of 'power' in statistical tests refers to the probability that the test will correctly reject a false null hypothesis.
- π Nonparametric tests make fewer assumptions about the data, which can be an advantage when dealing with data that does not meet the criteria for parametric analysis.
- π The script introduces the Sign Test as a simpler nonparametric method, which only considers the direction of change (increase or decrease), not the magnitude.
- π The Wilcoxon Signed-Rank Test improves upon the Sign Test by incorporating both the direction and magnitude of changes, through ranking the absolute differences.
- π’ The process of ranking involves ordering the absolute differences from smallest to largest and assigning a rank to each difference.
- π― The test statistic is calculated by comparing the sum of ranks for decreases versus increases, with the expectation that they should be roughly equal under the null hypothesis.
- π The p-value derived from the test indicates the probability of observing the sample results, or something more extreme, assuming the null hypothesis is true.
- π« The script emphasizes that the manual calculation of ranks and test statistics is not the focus, but rather understanding the conceptual approach of nonparametric testing.
Q & A
What is the Wilcoxon signed-rank test?
-The Wilcoxon signed-rank test is a nonparametric statistical test used to compare two related samples, or repeated measurements on a single sample, to assess whether their population mean ranks differ. It is an alternative to the paired sample t-test when the data cannot be assumed to be normally distributed.
Why might we use a nonparametric test like the Wilcoxon signed-rank test instead of a parametric test?
-We might use a nonparametric test when the sample size is small and a parametric approach is not feasible, or when we want to test the hypothesis about the median change rather than the mean. Nonparametric tests make fewer assumptions about the data, such as normality, and are more robust to outliers.
What is the main difference between parametric and nonparametric approaches in statistical testing?
-Parametric approaches make specific assumptions about the data distribution and often require larger sample sizes. Nonparametric approaches, on the other hand, make fewer assumptions and do not require the data to follow a specific distribution, making them suitable for smaller sample sizes and more robust against outliers.
What is the concept of the sign test in nonparametric statistics?
-The sign test is a simple nonparametric test that determines whether the median of a population is different from a specified value. It only considers the direction (sign) of the difference between paired observations, ignoring the magnitude of the changes.
Why is the sign test considered too simple to be useful in some cases?
-The sign test is considered too simple because it only looks at whether there was an increase or decrease in the data, without considering the magnitude of the change. This can lead to misleading results, as it treats large and small changes equally.
How does the Wilcoxon signed-rank test address the limitation of the sign test?
-The Wilcoxon signed-rank test addresses the limitation of the sign test by incorporating the magnitude of the changes in addition to their signs. It ranks the absolute differences in size and then sums the ranks for decreases and increases separately, providing a more nuanced comparison.
What is the purpose of ranking the absolute differences in the Wilcoxon signed-rank test?
-Ranking the absolute differences allows the test to take into account the magnitude of the changes, rather than just their direction. This helps to differentiate between small and large changes, providing a more accurate reflection of the data.
How does the Wilcoxon signed-rank test calculate the p-value?
-The p-value in the Wilcoxon signed-rank test is calculated based on the probability of observing the sample data's sum of ranks for decreases or something more extreme, assuming the null hypothesis is true. Smaller p-values indicate stronger evidence against the null hypothesis.
What is the consequence of rejecting the null hypothesis in the Wilcoxon signed-rank test?
-Rejecting the null hypothesis in the Wilcoxon signed-rank test suggests that there is a statistically significant difference in the medians of the two related samples, indicating that the treatment or condition being tested has had an effect.
How can the Wilcoxon signed-rank test be used in practice?
-The Wilcoxon signed-rank test can be used in practice to analyze data from experiments where the same subjects are measured before and after a treatment or intervention, such as in medical trials, to determine the effectiveness of a treatment without assuming normal distribution of the data.
Outlines
π Introduction to the Wilcoxon Signed-Rank Test
This paragraph introduces the Wilcoxon signed-rank test, a nonparametric alternative to the paired t-test, suitable for comparing paired numeric values. It highlights the test's utility in scenarios with small sample sizes or when the focus is on the median change rather than the mean. The paragraph contrasts parametric and nonparametric approaches, noting that nonparametric methods, while less powerful, make fewer assumptions about data distribution. It also briefly mentions bootstrapping as an alternative for small sample sizes or when avoiding normality assumptions is desired. The sign test is introduced as a foundational nonparametric approach, testing the null hypothesis that the median difference is zero against the alternative that it is not, specifically less than zero in this context.
π Understanding the Sign Test and Its Limitations
This paragraph delves deeper into the sign test, explaining its simplicity and limitations. It describes how the test only considers the direction of change (increase or decrease) without accounting for the magnitude. An example is provided to illustrate the test's process, where the number of individuals experiencing a decrease or increase in systolic blood pressure after treatment is counted. The expected 50-50 split under the null hypothesis is discussed, along with the calculation of the p-value using a binomial distribution. The paragraph emphasizes the test's inability to differentiate between small and large changes, using an exaggerated example to highlight this issue.
π Enhancing Nonparametric Analysis with the Wilcoxon Signed-Rank Test
Building upon the sign test, this paragraph introduces the Wilcoxon signed-rank test, which incorporates the magnitude of changes by ranking them. It explains the process of ranking absolute changes from smallest to largest and then summing the ranks for decreases and increases separately. The expectation under the null hypothesis is that the ranks should be evenly distributed between increases and decreases. The paragraph provides an example with tied ranks, explaining how to average them, and emphasizes the importance of understanding the conceptual approach rather than focusing on mechanical calculations.
π Applying the Wilcoxon Signed-Rank Test to Data
This paragraph demonstrates the application of the Wilcoxon signed-rank test to a larger dataset, showing the process of assigning ranks to the absolute differences and calculating the sum of ranks for both decreases and increases. It discusses the expected sums under the null hypothesis and compares them to the observed sums in the sample data. The calculation of the p-value to determine the probability of observing the sample results or something more extreme if the null hypothesis were true is explained. The paragraph concludes with the interpretation of the p-value, leading to the rejection of the null hypothesis in the given example, and reinforces the importance of understanding the nonparametric approach's underlying concepts.
Mindmap
Keywords
π‘Wilcoxon Signed-Rank Test
π‘Nonparametric
π‘Paired or Dependent Samples
π‘Median Change
π‘Power
π‘Bootstrapping
π‘Sign Test
π‘Magnitude of Change
π‘Ranks
π‘P-Value
Highlights
Introduction of the Wilcoxon signed-rank test as a nonparametric alternative to the paired t-test.
Comparison of two groups on a numeric value that are paired or dependent.
Use of the Wilcoxon signed-rank test for small sample sizes or when a parametric approach is not feasible.
Testing the hypothesis about the median change rather than the mean.
Differences between parametric and nonparametric approaches in terms of power and assumptions.
Advantages of nonparametric approaches, such as fewer assumptions and no requirement for large sample sizes or normality.
Introduction of bootstrapping or resampling as alternative approaches for small sample sizes or reduced assumptions.
Explanation of the sign test as a simple form of a nonparametric approach and its limitations.
The sign test's focus on the direction of change (increase or decrease) without considering the magnitude.
Calculation of p-value for the sign test using a binomial distribution.
The Wilcoxon signed-rank test's incorporation of both the sign and magnitude of changes.
Ranking the absolute changes to account for the magnitude in the Wilcoxon signed-rank test.
Procedure for assigning average ranks to tied observations in the ranking process.
Concept of calculating expected sums of ranks under the null hypothesis for both increases and decreases.
Comparison of observed sums of ranks to expected values to determine the p-value.
Rejection of the null hypothesis based on a small p-value, indicating evidence for the alternative hypothesis.
Advantages of nonparametric tests in terms of reduced sensitivity to outliers.
Trade-offs of using ranks in nonparametric tests, including the loss of some information about the magnitude of changes.
Emphasis on understanding the conceptual framework of nonparametric tests rather than manual calculation details.
Transcripts
Browse More Related Video

Paired t Test | Statistics Tutorial #21| MarinStatsLectures
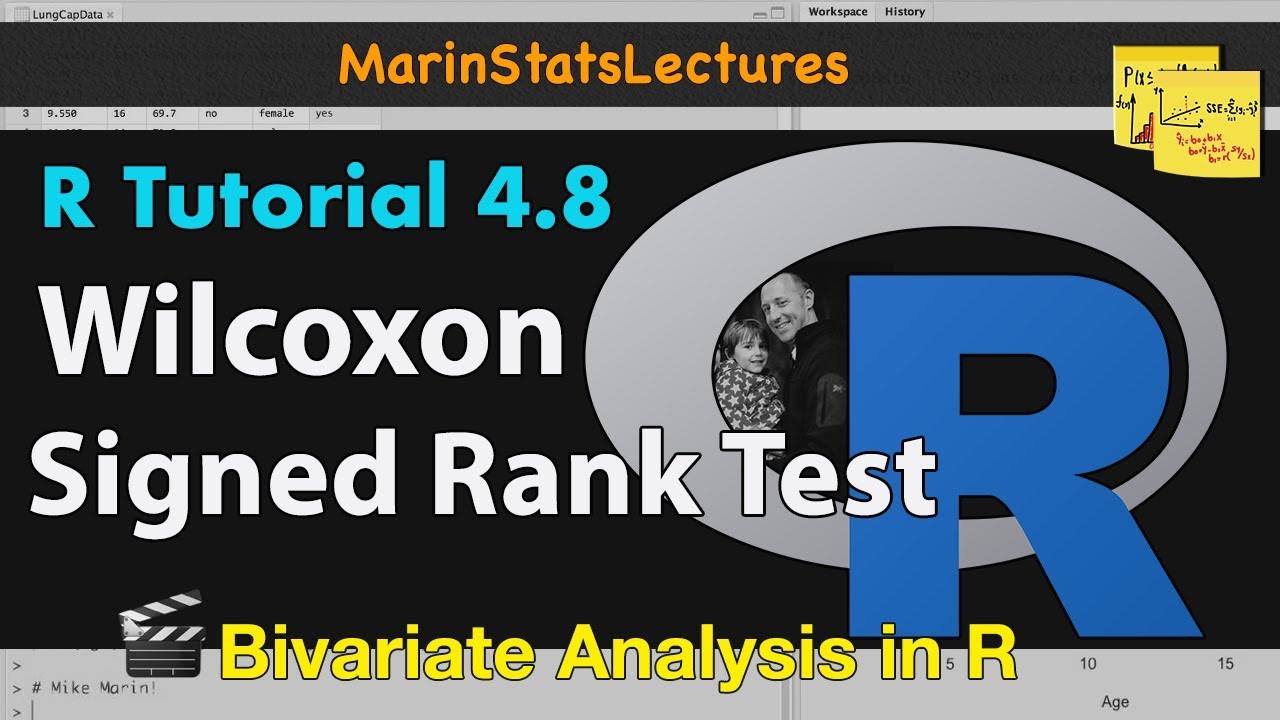
Wilcoxon Signed Rank Test in R with Example | R Tutorial 4.8 | MarinStatsLectures
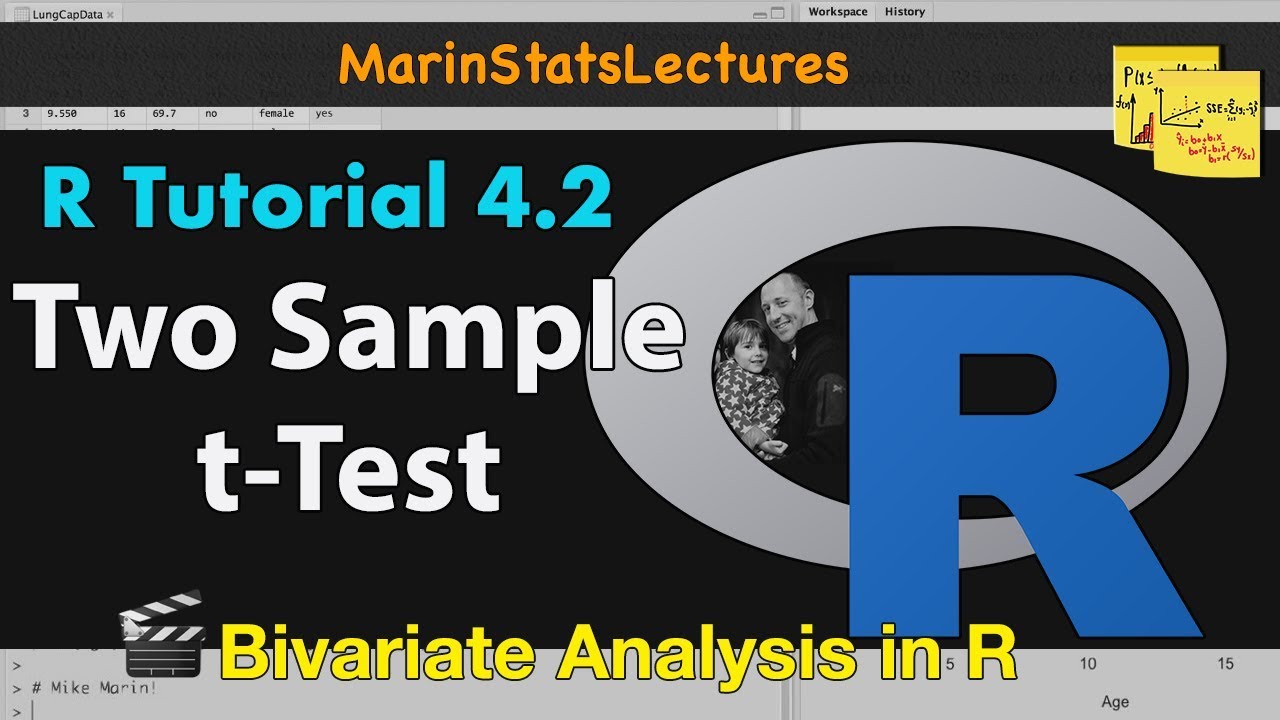
Two-Sample t Test in R (Independent Groups) with Example | R Tutorial 4.2 | MarinStatsLectures

Paired t-Test in R with Examples | R Tutorial 4.7 | MarinStatsLectures
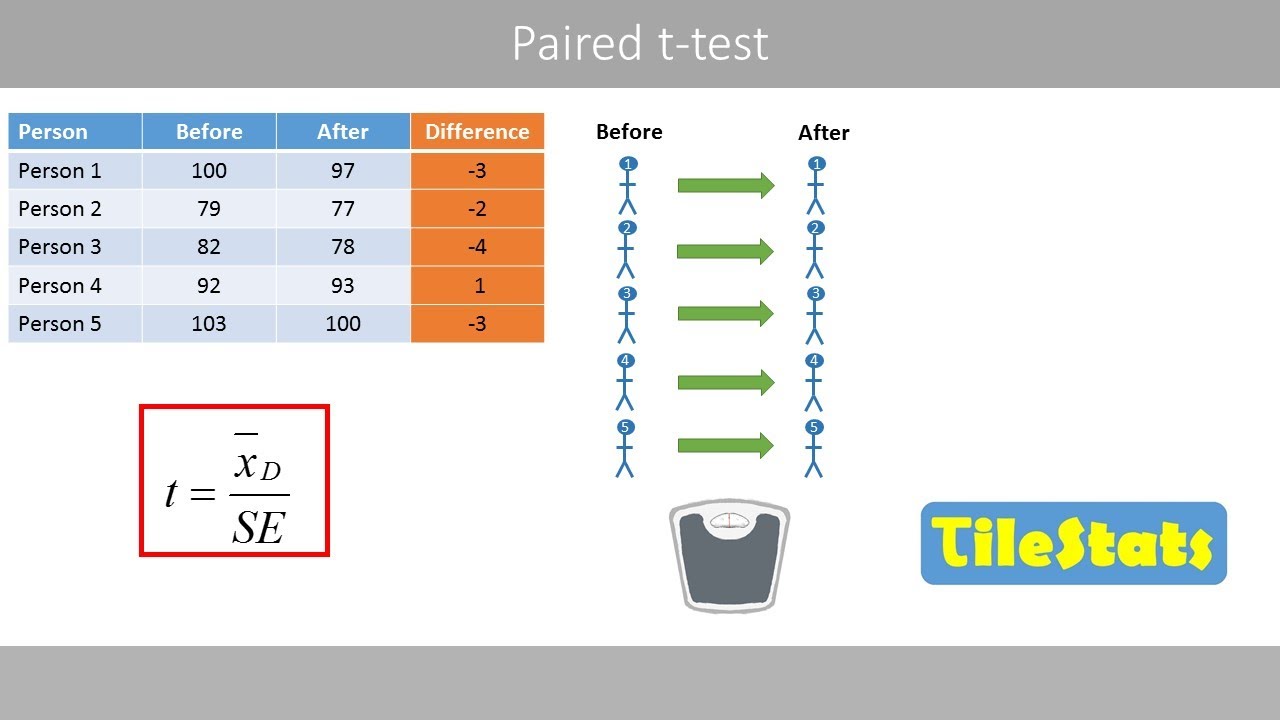
The paired t-test | explained with a simple example
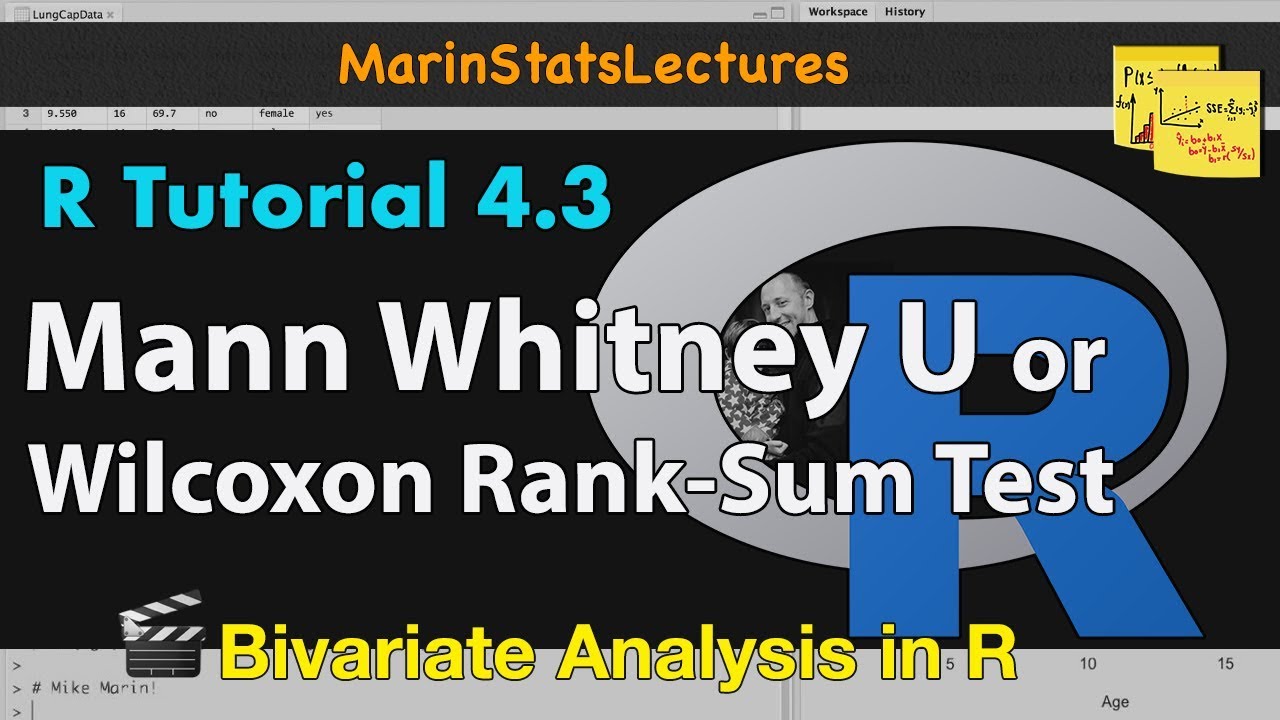
Mann Whitney U / Wilcoxon Rank-Sum Test in R | R Tutorial 4.3 | MarinStatsLectures
5.0 / 5 (0 votes)
Thanks for rating: