5.1.3 Discrete Probability Distributions - Finding the Mean, Variance, and Standard Deviation
TLDRThis video script delves into calculating population parameters such as mean, variance, and standard deviation from a probability distribution. It emphasizes the difference between population parameters and sample statistics, explaining that the calculated values represent theoretical expectations of the entire population. The script outlines the formulas for these calculations, highlighting an alternative variance formula for ease of use, and illustrates the process with an example involving an X-linked genetic disorder. It also touches on the concept of expected value and its relation to the law of large numbers, demonstrating how to apply these statistical measures in practical scenarios.
Takeaways
- π The lesson discusses how to find the population mean, variance, and standard deviation from a probability distribution, emphasizing the difference between population parameters and sample statistics.
- π When using a probability distribution, we are calculating population parameters, not sample statistics, which is crucial for understanding the theoretical expectations of the population.
- π² The mean (ΞΌ), variance (ΟΒ²), and standard deviation (Ο) of a population are calculated using specific formulas, where ΞΌ represents the average value expected over infinitely many trials.
- π’ To calculate the mean, multiply each value of the random variable x by its corresponding probability and sum these products to get the population mean.
- π Variance measures the spread of the data around the mean, calculated by squaring the deviation of each x value from the mean, multiplying by the probability, and summing these values.
- β An alternative formula for variance is often easier to use, especially for hand calculations or using tools like Excel, and it is equivalent to the more complex formula.
- π The standard deviation is the square root of the variance, providing a measure of dispersion in the same units as the random variable.
- π§ The concept of expected value (E[x]) is introduced as the theoretical mean value of a discrete random variable over infinitely many trials, equivalent to the population mean.
- π The law of large numbers is connected to the concept of expected value, stating that as trials are repeated, the relative frequency of an event approaches its actual probability.
- π The script provides a step-by-step guide on how to calculate the mean, variance, and standard deviation using an example of an X-linked genetic disorder inheritance among children.
- π Excel is suggested as a tool for calculations, with a demonstration of how to use formulas to calculate the mean and variance in a spreadsheet.
Q & A
What is the main focus of the video script?
-The video script focuses on explaining how to find the population mean, variance, and standard deviation given a probability distribution, and it also discusses the rationale behind the formulas used for these calculations.
Why are the mean, standard deviation, and variance calculated from a probability distribution different from those calculated from a sample?
-The mean, standard deviation, and variance calculated from a probability distribution are population parameters, not sample statistics. They describe the entire population, whereas sample statistics are estimates derived from a subset of the population.
What are the notations used for the population mean, variance, and standard deviation?
-The population mean is represented by the Greek letter mu (ΞΌ), the population variance is represented by sigma squared (Ο^2), and the population standard deviation is represented by the lowercase sigma (Ο).
How is the population mean calculated for a probability distribution?
-The population mean is calculated by multiplying each value of the random variable x by its corresponding probability and then summing all these products together.
What is the rationale behind squaring the deviation in the calculation of variance?
-Squaring the deviation ensures that all deviations from the mean are counted as positive, regardless of whether they are above or below the mean. This is necessary because variance measures the spread of the data without considering the direction of the deviation.
Why is the second formula for calculating variance recommended over the first one?
-The second formula for calculating variance is recommended because it is easier to evaluate and can be more conveniently used for hand calculations or in software like Excel, making it more accessible for practical use.
What is the relationship between the expected value and the mean of a population?
-The expected value and the mean of a population are the same thing. The expected value represents the theoretical mean value of infinitely many trials, which is the average value we would expect if we were to repeat a procedure indefinitely.
How is the law of large numbers related to the concept of expected value?
-The law of large numbers states that as a procedure is repeated, the relative frequency of an event tends to approach the actual probability. The expected value uses a similar idea, where each value of the random variable x is multiplied by its corresponding probability, and the sum of these products gives the expected value.
Can you provide an example of how to calculate the mean, variance, and standard deviation using the formulas discussed in the script?
-The script provides an example involving five males with an X-linked genetic disorder and their children. The mean is calculated by multiplying each possible number of children who inherit the disorder by its probability and summing these products. The variance is found by squaring each x value, multiplying by the probability, summing these, and then subtracting the square of the mean. The standard deviation is the square root of the variance.
What is the significance of the units in variance compared to the original random variable?
-The units of variance are the square of the units of the original random variable. This is because variance measures the spread of the data and involves squaring the deviations from the mean, which changes the units to the square of the original variable's units.
Outlines
π Understanding Population Parameters from Probability Distributions
This paragraph introduces the concept of calculating population parameters such as mean, variance, and standard deviation from a probability distribution. It clarifies that these calculations are not about sample statistics but rather about theoretical values that describe an entire population. The paragraph explains the notation used for these parameters (\( \mu \) for mean, \( \sigma^2 \) for variance, and \( \sigma \) for standard deviation) and provides the formulas for calculating them. It emphasizes that the mean is found by multiplying each value of the random variable \( x \) by its corresponding probability and summing these products. Variance is calculated by squaring the deviation of each \( x \) value from the mean, multiplying by the probability, and summing these values. The paragraph also introduces an alternative formula for variance that is easier to use in practice and concludes by defining the expected value (\( E \)) as the theoretical mean over infinitely many trials, which is equivalent to the population mean.
π§ Theoretical Basis of Expected Value and the Law of Large Numbers
The second paragraph delves into the theoretical underpinnings of the expected value, drawing a connection to the law of large numbers discussed in a previous lesson. It uses the example of flipping a fair coin to illustrate how relative frequency approaches the actual probability over a large number of trials. The expected value is then explained as the sum of each possible value of a random variable \( x \) multiplied by its corresponding probability, which represents the average outcome over many trials. The paragraph also discusses the rationale behind the formula for the mean, relating it to the sample mean from a frequency distribution and explaining how the formula can be adapted for discrete probability distributions by considering the probability of each \( x \) value as the proportion of the sample in a class.
π¨βπ¦βπ¦ Calculating Mean, Variance, and Standard Deviation for a Discrete Distribution
This paragraph presents a practical application of the formulas for calculating the mean, variance, and standard deviation using a discrete probability distribution. It describes a scenario involving five males with an X-linked genetic disorder and their children, where the random variable is the number of children who inherit the disorder. The paragraph guides through the process of finding the mean by creating a column for \( x \) times the probability and summing these values. It also demonstrates how to use Excel for calculations, highlighting the importance of double-checking results for accuracy. The mean is interpreted as the average number of children with the disorder in a large number of trials, aligning with the law of large numbers.
π Excel Techniques for Variance Calculation
The fourth paragraph continues the practical demonstration by showing how to calculate variance in Excel. It explains the process of creating an \( x^2 \) column, multiplying it by the probability of \( x \), and summing these products to get the first term of the variance formula. The paragraph then details the subtraction of the squared mean from this sum to obtain the variance. It emphasizes the importance of units in variance, noting that they are squared units of the random variable. The paragraph also includes a minor correction in the Excel demonstration, illustrating the process of verifying and adjusting calculations to ensure accuracy.
π Interpreting Results and Relating to the Law of Large Numbers
The final paragraph concludes the lesson by interpreting the calculated mean, variance, and standard deviation in the context of the genetic disorder scenario. It reiterates the mean as the expected number of children inheriting the disorder over many trials and connects this to the law of large numbers, which states that the relative frequency of an event will approach its actual probability as trials increase. The paragraph also explains how to calculate the standard deviation as the square root of the variance, providing a measure of the variation in probabilities. The summary wraps up the learning outcome with an invitation to the next video, which will discuss the range rule of thumb.
Mindmap
Keywords
π‘Population Mean
π‘Variance
π‘Standard Deviation
π‘Probability Distribution
π‘Parameters
π‘Expected Value
π‘Law of Large Numbers
π‘Discrete Random Variable
π‘Sample Mean
π‘Relative Frequency
Highlights
The video discusses learning outcome number three for lesson 5.1, focusing on finding population mean, variance, and standard deviation from a probability distribution.
Parameters like population mean, variance, and standard deviation are theoretical and describe the entire population, not just a sample.
The mean (ΞΌ), variance (ΟΒ²), and standard deviation (Ο) are represented with specific notations and are calculated using distinct formulas.
The mean is calculated by multiplying each value of the random variable x by its corresponding probability and summing these products.
Variance measures data variation and is calculated by squaring the deviation of each x value from the mean, multiplying by the probability, and summing these values.
An alternative formula for variance is often easier for hand calculations or using tools like Excel, and it's equivalent to the more complex formula.
Standard deviation is derived by taking the square root of the variance.
The expected value of a discrete random variable is represented by E(X) and is equivalent to the mean, representing the theoretical average of infinitely many trials.
The law of large numbers is related to expected value, stating that the relative frequency of an event will approach the actual probability as trials increase.
The rationale behind the formulas is explained, emphasizing the importance of understanding their derivation rather than just applying them.
A comparison is made between the sample mean formula from a frequency distribution and the population mean formula, illustrating their relationship.
An example is provided to demonstrate the calculation of mean, variance, and standard deviation using the formulas with a discrete probability distribution.
Excel is used to calculate the mean, showing the process of creating a table with x values, probabilities, and their products.
The mean of 2.5 children is explained as an average over infinitely many trials, not a literal number of children per family.
Variance is calculated in Excel by creating an x squared column, multiplying by probabilities, and then adjusting for the mean squared.
The units of variance are peculiar, being the square of the units of the random variable, which in this case are 'children squared'.
The standard deviation, 1.116 children, is a measure of the variation in the probabilities of the number of children inheriting the genetic disorder.
The video concludes with an interpretation of the calculated mean and standard deviation in the context of the genetic disorder example.
Transcripts
Browse More Related Video
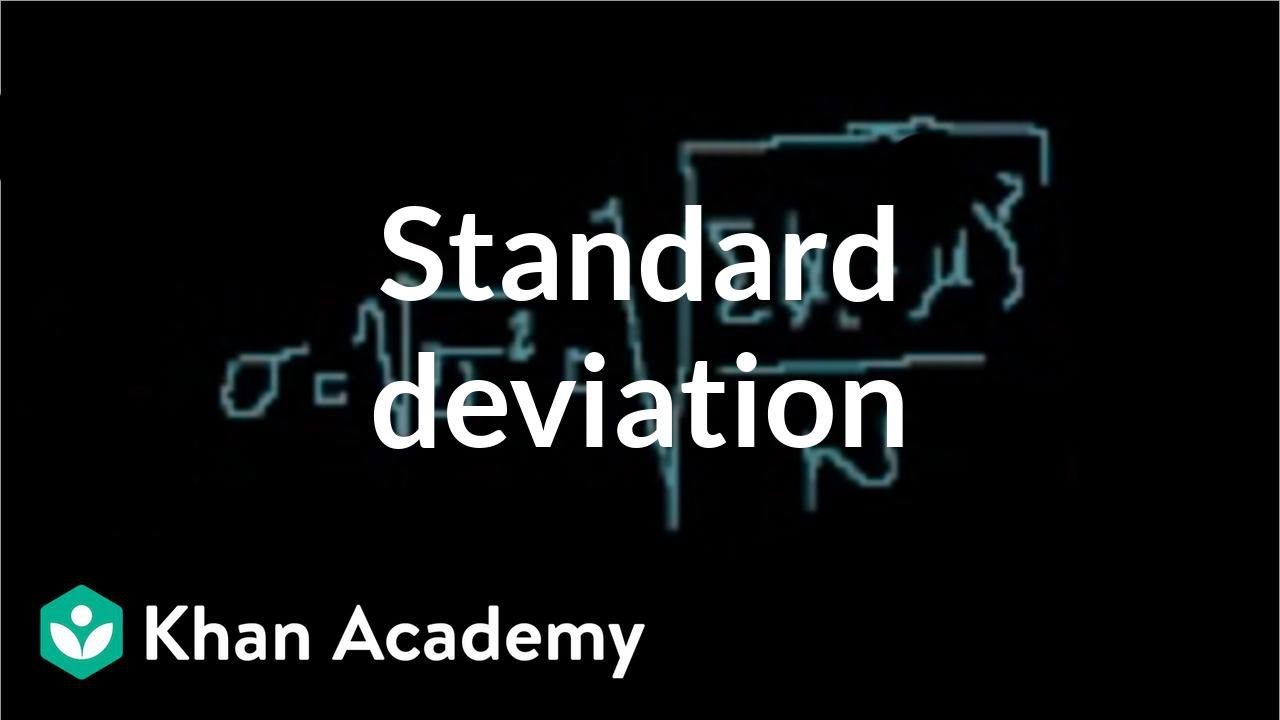
Statistics: Standard deviation | Descriptive statistics | Probability and Statistics | Khan Academy
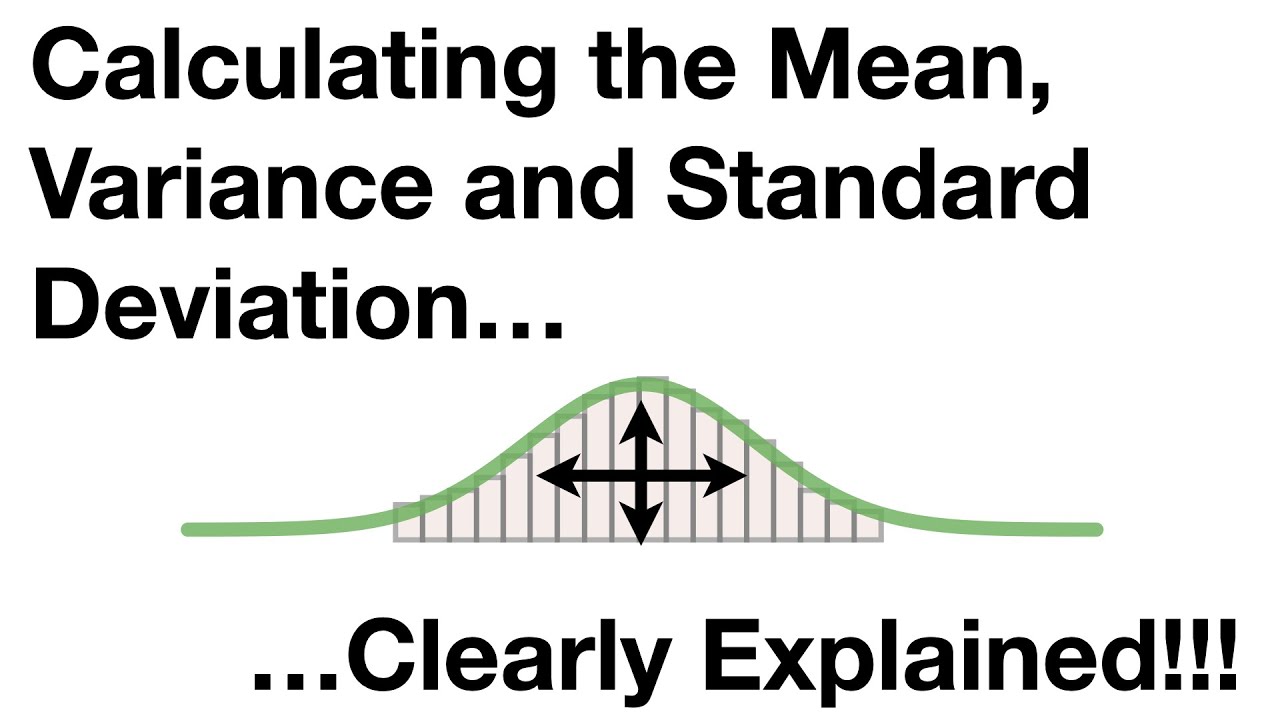
Calculating the Mean, Variance and Standard Deviation, Clearly Explained!!!
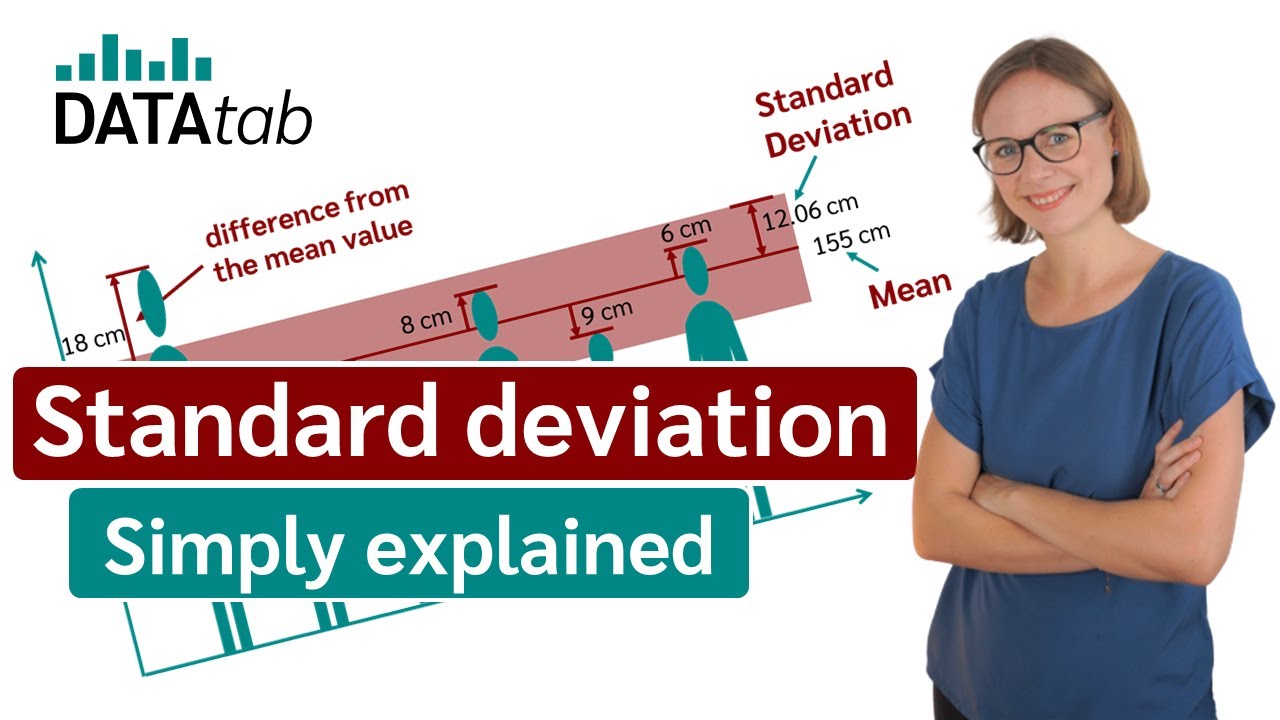
Standard deviation (simply explained)

Measures of Dispersion (Ungrouped Data) | Basic Statistics
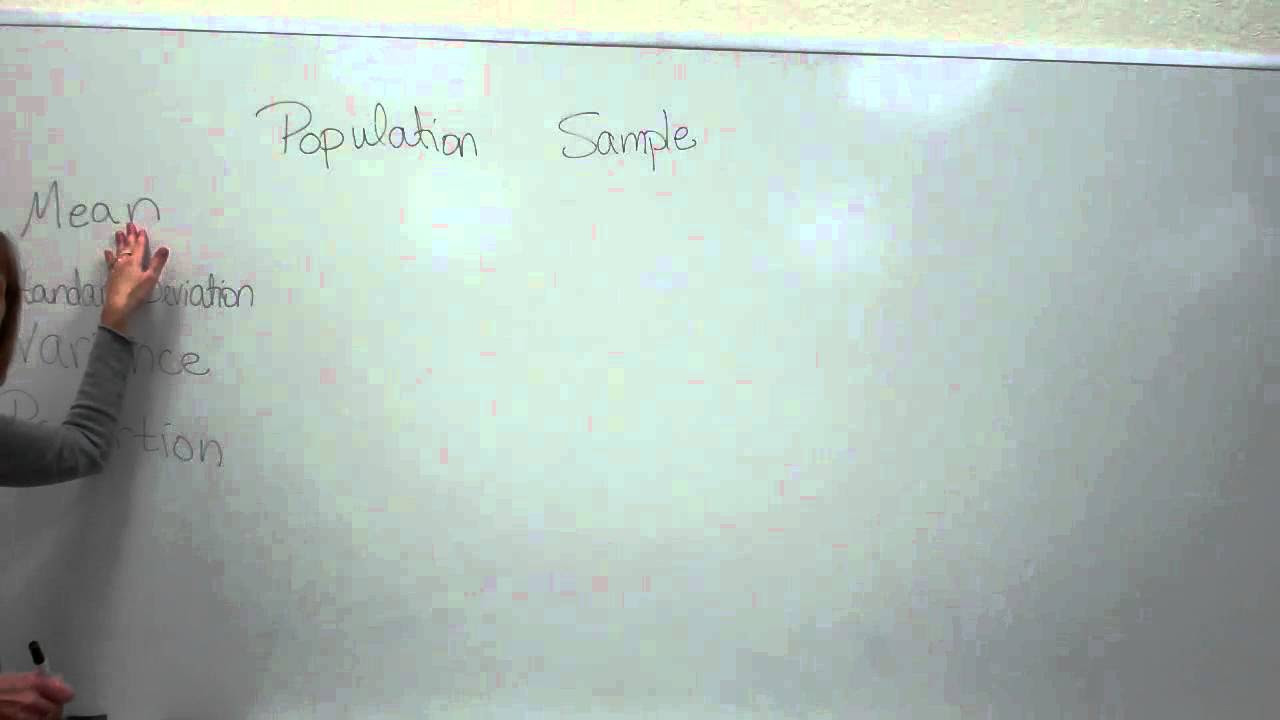
Symbols commonly used in statistics
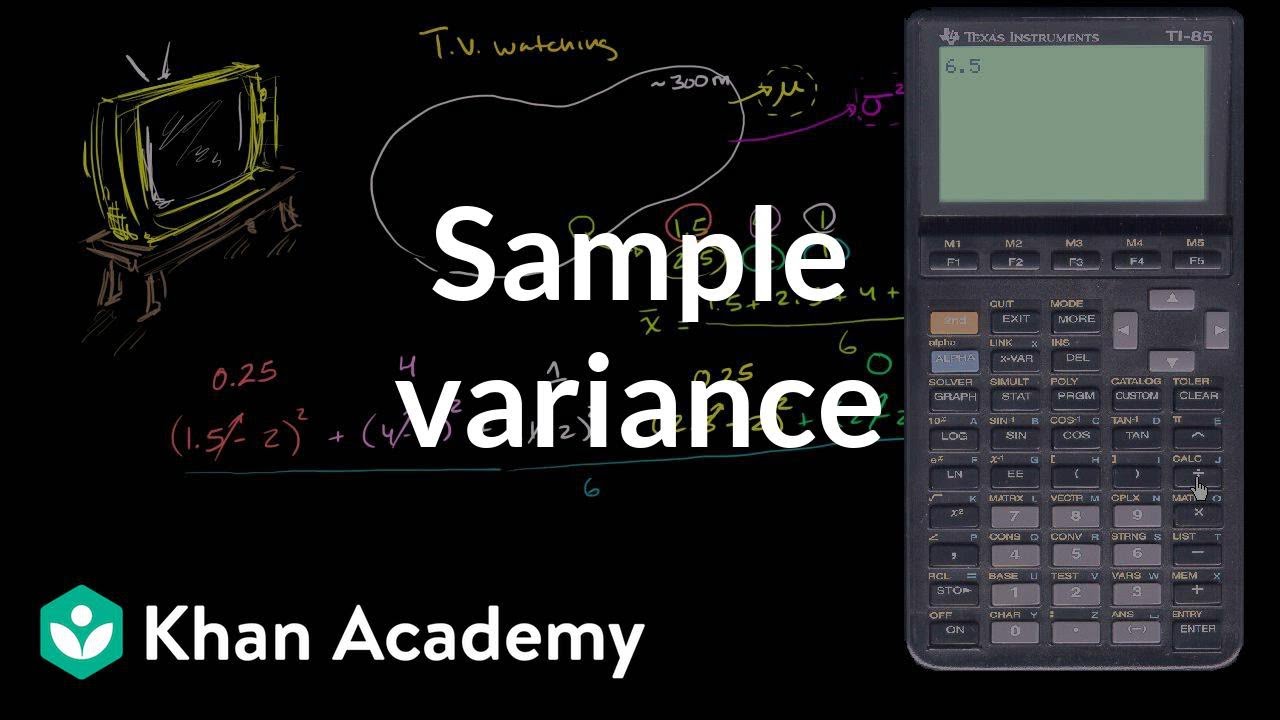
Sample variance | Descriptive statistics | Probability and Statistics | Khan Academy
5.0 / 5 (0 votes)
Thanks for rating: