COX REGRESSION and HAZARD RATIOS - easily explained with an example!
TLDRThis educational video introduces Cox regression, a statistical method for analyzing survival times and the impact of various factors on them. It explains how Cox regression, unlike the log-rank test, allows for the inclusion of explanatory variables such as gender, smoking habits, blood pressure, and genetic mutations. The video clarifies the concept of hazard rates and how covariates are used to model the hazard function. It discusses the outputs of Cox regression, focusing on coefficients and hazard ratios, and provides an example comparing a new drug's effect on survival time to a standard treatment, while adjusting for gender and age. The importance of checking assumptions before using Cox regression is also highlighted to avoid false conclusions.
Takeaways
- π The Log-Rank Test is used to compare survival times between different groups, like patients given different drugs.
- π The Log-Rank Test does not account for other factors that might affect survival, such as gender, smoking, blood pressure, or genetic mutations.
- π Cox Proportional Hazards Regression, also known as the Cox model, is used to analyze survival times while considering other explanatory variables.
- β± The hazard rate in Cox regression is the probability of an event occurring given survival up to a specific point in time, like the risk of death at a particular moment.
- π Cox regression allows for the inclusion of covariates, which are independent variables that might influence the time to event, and can be categorical, discrete, or continuous.
- π After performing Cox regression, the main outputs are coefficients and hazard ratios, which help interpret the impact of covariates on survival time.
- π A positive coefficient indicates an increased log hazard (higher risk), a negative coefficient indicates a decreased log hazard (lower risk), and a coefficient near zero suggests little effect on survival time.
- π’ Hazard ratios are obtained by exponentiating the coefficients and represent the relative change in hazard for a one-unit change in the covariate, with values greater than one indicating increased hazard and less than one indicating decreased hazard.
- π An example given in the script is comparing a new medication (Drug A) with a standard treatment (Drug B), adjusting for factors like gender and age, to see if the new drug improves survival time.
- π In the example, a hazard ratio of 0.6 for Drug A suggests a 40% lower risk of death compared to Drug B, although the significance of this finding depends on the P-value and confidence interval.
- π Cox regression has key assumptions, such as proportional hazards over time, independence of survival times between individuals, and independent censoring, which must be checked to avoid false conclusions.
Q & A
What is the primary purpose of the log-rank test?
-The log-rank test is used to determine if there is a significant difference in survival times between different groups, such as comparing the survival times of patients given different treatments.
Why is Cox proportional hazards regression model preferred over the log-rank test?
-Cox regression, also known as the proportional hazards model, is preferred because it allows for the inclusion of other explanatory variables that may affect survival time, which the log-rank test does not accommodate.
What does the hazard rate represent in the context of survival analysis?
-The hazard rate represents the probability of an event occurring, such as death, given that an individual has survived up to a specific point in time.
What are covariates in Cox regression, and how are they used?
-Covariates are independent variables that are thought to influence the time to an event. They can be categorical, discrete, or continuous and are used to model how different factors affect the hazard function.
What is the significance of coefficients in Cox regression?
-The coefficients in Cox regression indicate the log hazard ratio associated with each covariate, assuming all other covariates remain constant. They help determine the effect of each covariate on the hazard rate.
How do you interpret a positive coefficient in Cox regression?
-A positive coefficient indicates an increase in the log hazard, which means a higher risk of the event occurring.
What does a negative coefficient in Cox regression signify?
-A negative coefficient signifies a decrease in the log hazard, indicating a lower risk of the event occurring.
What is a hazard ratio, and how is it used in Cox regression?
-The hazard ratio is the exponentiated form of the coefficient and quantifies the relative change in the hazard of an event for a one-unit change in the covariate. It provides a direct interpretation of the effect of covariates on the hazard.
How does a hazard ratio greater than one impact the interpretation of Cox regression results?
-A hazard ratio greater than one indicates an increased hazard, suggesting that the risk of the event is higher for the group or condition associated with that covariate.
What does a hazard ratio less than one mean in the context of Cox regression?
-A hazard ratio less than one indicates a decreased hazard, suggesting that the risk of the event is lower for the group or condition associated with that covariate.
What are some key assumptions of Cox regression that need to be checked before using it?
-Key assumptions of Cox regression include the proportionality of hazards over time, the independence of survival times between individuals, and that censoring is independent of the survival time.
Outlines
π§ͺ Introduction to Cox Proportional Hazards Model
This paragraph introduces the Cox proportional hazards model, a statistical technique used in survival analysis to assess the impact of various factors on survival times. It explains how the model differs from the log-rank test by allowing for the inclusion of explanatory variables such as gender, smoking habits, blood pressure, genetic mutations, exercise, and diet. The paragraph also defines the hazard rate as the probability of an event occurring at a given time, given survival up to that point, using the example of death in a clinical trial. The Cox model is highlighted for its ability to incorporate covariates to understand their influence on the hazard function.
π Understanding Cox Regression Outputs: Coefficients and Hazard Ratios
This paragraph delves into the outputs of Cox regression, focusing on coefficients and hazard ratios. It explains that each covariate in the model is associated with a coefficient, which indicates the log hazard ratio and can be positive (indicating an increased risk), negative (indicating a decreased risk), or close to zero (indicating little to no effect on survival time). The concept of hazard ratios is introduced as the exponentiated form of the coefficients, which provide a direct measure of the relative change in hazard for a one-unit change in the covariate. The paragraph uses an example of a clinical trial comparing a new drug to a standard treatment, along with gender and age as covariates, to illustrate how to interpret the results, including significance levels, hazard ratios, and confidence intervals.
β οΈ Key Assumptions of Cox Regression
The final paragraph discusses the assumptions underlying the Cox regression model. It emphasizes the proportional hazards assumption, which posits that the relative risk of an event for any individual is constant over time compared to any other individual. The paragraph also mentions the assumption that the survival time of one individual is independent of another's, and that censoring is independent of the survival time. A note of caution is sounded regarding the importance of checking these assumptions before applying Cox regression to avoid drawing false conclusions.
Mindmap
Keywords
π‘Log Rank Test
π‘Cox Proportional Hazards Model
π‘Hazard Rate
π‘Covariates
π‘Coefficients
π‘Hazard Ratio
π‘Significance Threshold
π‘Confidence Interval
π‘Assumptions of Cox Regression
π‘Censoring
Highlights
The log-rank test is used to compare survival times between different groups.
Cox regression, also known as the proportional hazards model, allows for the analysis of survival times with other explanatory variables.
The hazard rate in Cox regression is the probability of an event occurring given survival up to a specific point in time.
Cox regression can include covariates such as gender, blood pressure, and age to model how different factors affect the hazard function.
Coefficients from the Cox model indicate the log hazard ratio associated with each covariate, assuming other covariates remain constant.
A positive coefficient indicates an increased hazard or higher risk, while a negative coefficient indicates a decrease.
A coefficient of approximately zero suggests no significant effect of the variable on survival time.
The hazard ratio, obtained by exponentiating the coefficient, quantifies the relative change in hazard for a one-unit change in the covariate.
A hazard ratio greater than one indicates an increased hazard, while a ratio less than one indicates a decreased hazard.
An example hazard ratio of 0.6 suggests that patients who received a new medication had a 40% lower hazard of death compared to a standard treatment.
Significance of results in Cox regression is determined using a threshold, typically 0.05, with P values indicating association strength.
In a clinical trial example, age and gender were found to be significantly associated with survival length.
The hazard ratio for a new drug compared to a standard treatment can indicate the relative risk reduction.
A confidence interval containing one suggests that there may not be a significant difference between treatments.
Statistical significance of age indicates that older patients have an increased risk of the event.
Gender's effect on survival, with a hazard ratio of approximately 0.4, suggests women have a lower risk of death compared to men.
Cox regression assumes that the hazard for any individual is proportional to the hazard for any other individual, constant over time.
The survival time of one individual should not affect another's survival time in Cox regression.
Censoring should be independent of survival time to avoid biasing Cox regression results.
Transcripts
Browse More Related Video
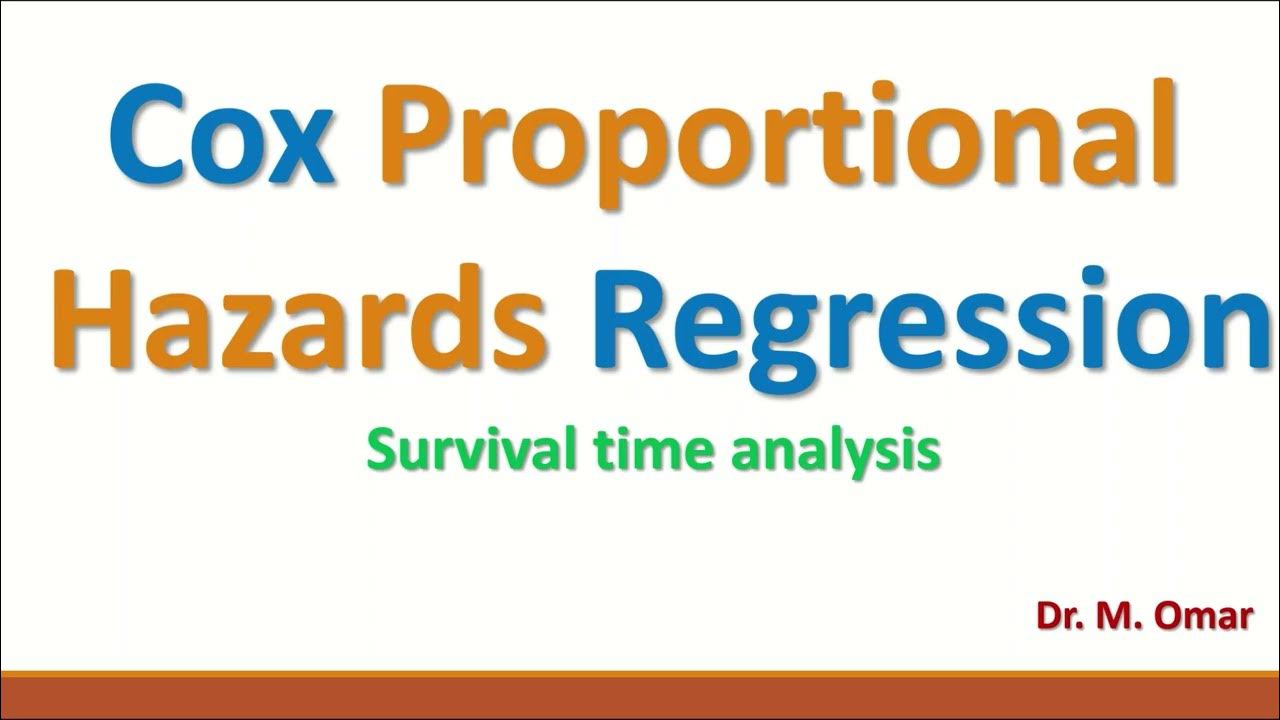
Cox Proportional Hazards Regression Survival time analysis
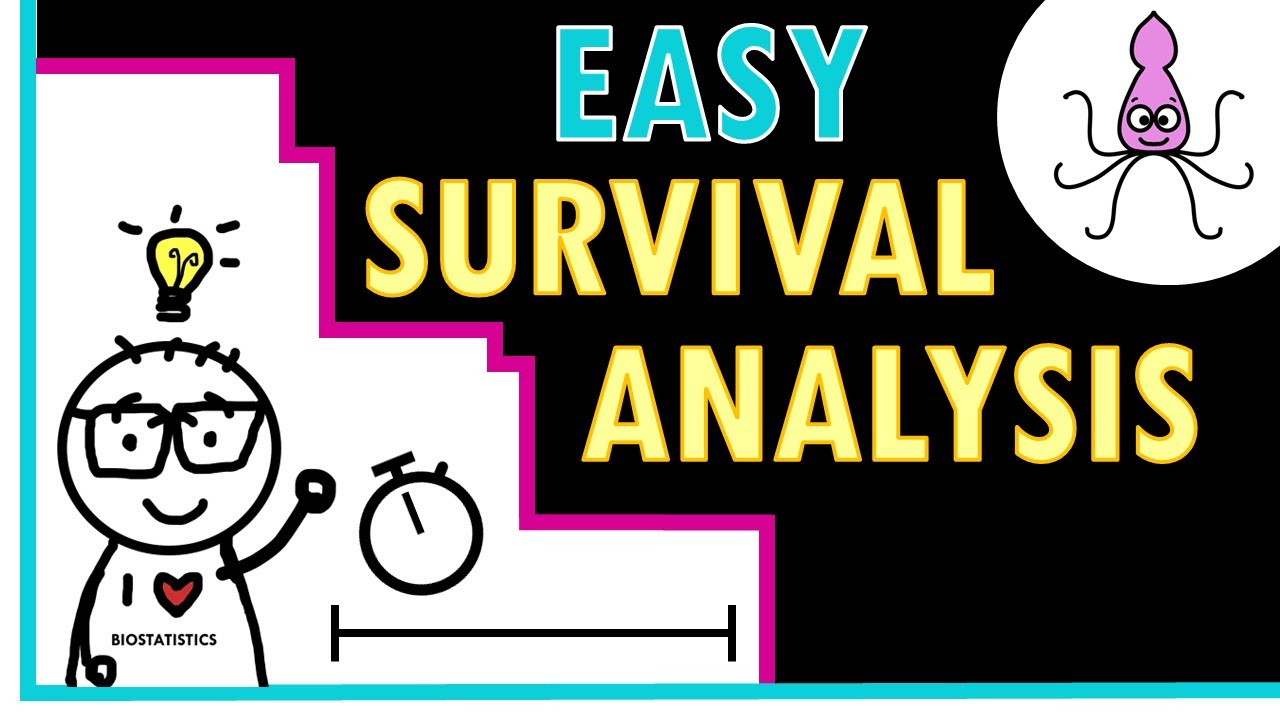
Easy survival analysis - simple introduction with an example!
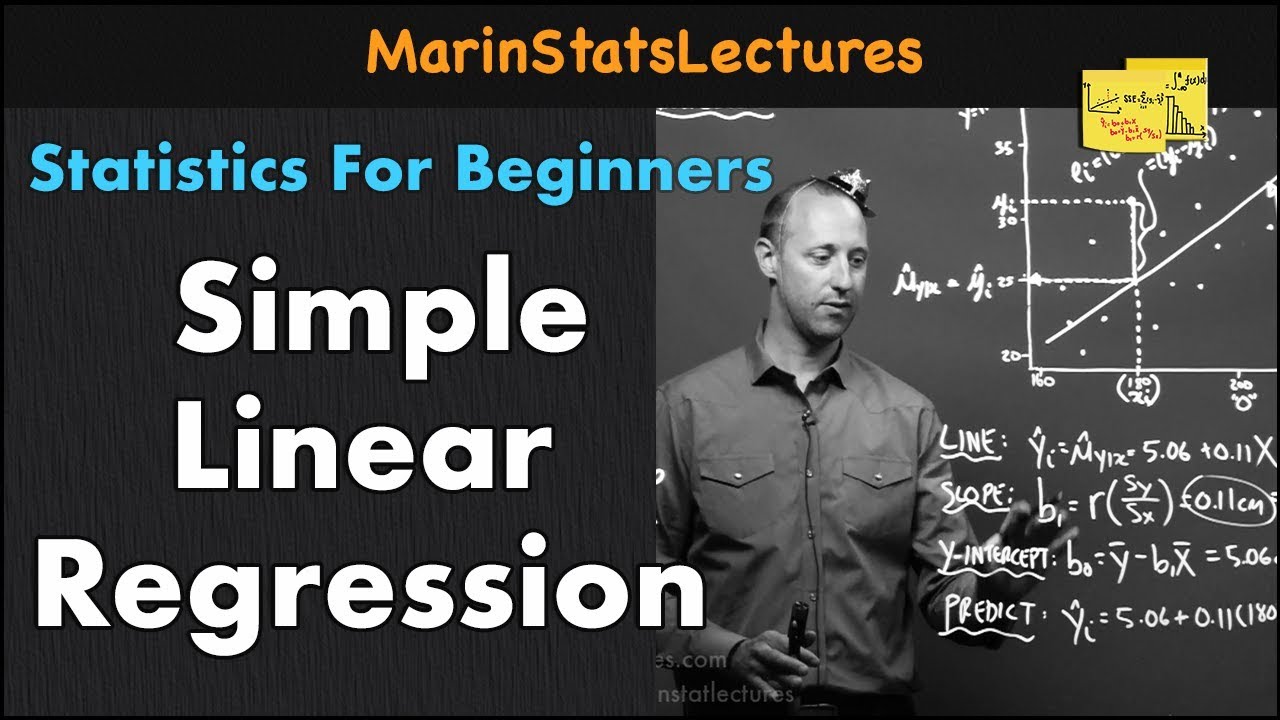
Simple Linear Regression Concept | Statistics Tutorial #32 | MarinStatsLectures
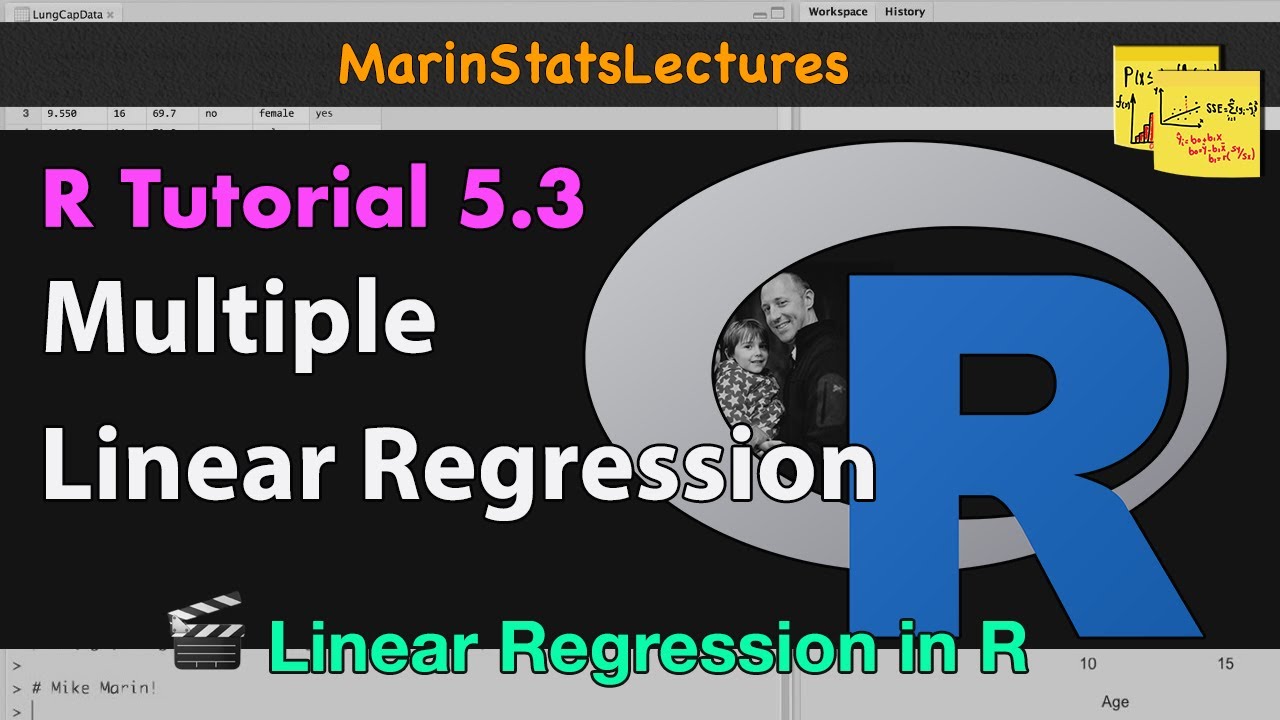
Multiple Linear Regression in R | R Tutorial 5.3 | MarinStatsLectures
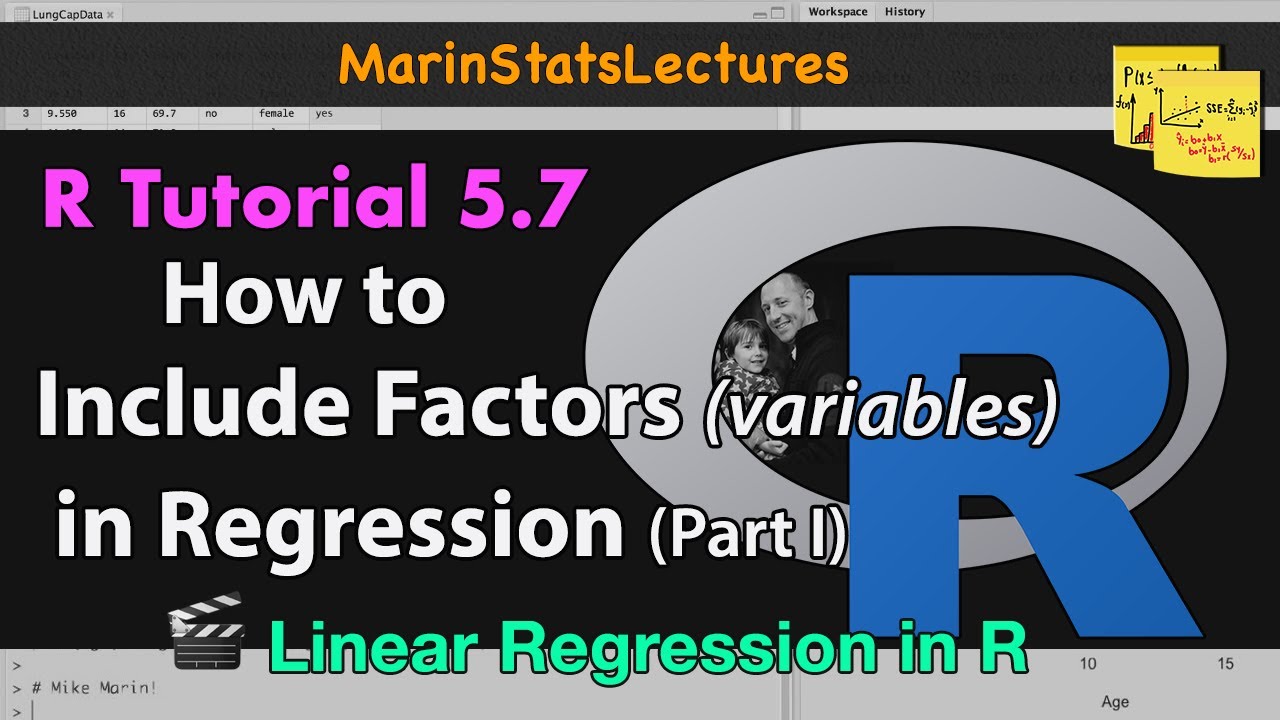
Including Variables/ Factors in Regression with R, Part I | R Tutorial 5.7 | MarinStatsLectures
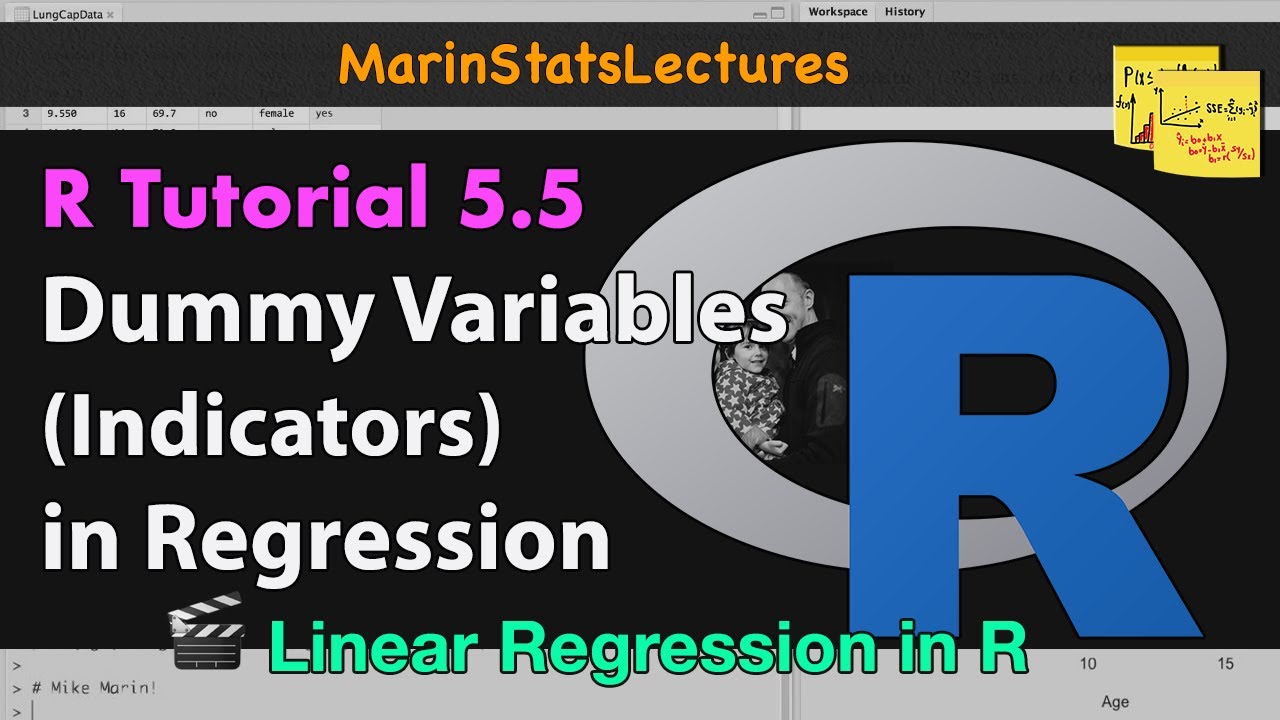
Dummy Variables or Indicator Variables in R | R Tutorial 5.5 | MarinStatsLectures
5.0 / 5 (0 votes)
Thanks for rating: