Cohenβs d Effect Size for t Tests (10-7)
TLDRThe video script emphasizes the importance of understanding statistical significance beyond the traditional alpha level of .05 and p-values. It highlights the necessity of reporting effect sizes and confidence intervals to provide a more comprehensive evaluation of research findings. Effect size, measured through Cohen's D, offers a standardized way to assess the practical impact of a treatment or difference. The script also clarifies the difference between statistical significance and effect size, using an example to illustrate how similar effect sizes can lead to different p-values due to sample size and study power. It further explains Cohen's conventions for interpreting effect sizes and the importance of considering effect size in research design and reporting.
Takeaways
- π’ There is no inherent magic in setting alpha at .05; it's a conventional threshold for statistical significance.
- π A p-value alone is insufficient to determine the practical significance of an effect; it only tells us the probability of observing the data assuming the null hypothesis is true.
- π It's important to report effect sizes and confidence intervals alongside findings to provide a more complete picture of the impact of a study's results.
- π Effect size is a standardized measure that quantifies the magnitude of an effect, helping to assess the practical significance of a treatment or intervention.
- π Cohen's D is a commonly used measure of effect size for T tests, providing a standardized way to compare the magnitude of differences.
- π Statistical significance indicates whether observed differences are likely due to chance, while effect size measures the practical importance of those differences.
- π Effect size can reveal consistent findings across studies, even when p-values differ due to variations in sample size or study power.
- π Jacob Cohen established conventions for interpreting effect sizes as small, medium, or large, which are based on the degree of overlap between two distributions.
- π¬ Cohen's conventions for effect sizes are arbitrary but useful for providing a framework to understand the practical significance of research findings.
- π‘ Effect size can guide power analysis, helping researchers determine the appropriate sample size needed to detect an effect of a given magnitude.
- π Reporting effect size is recommended by the APA and is crucial for interpreting the results of studies, especially when sample sizes are small or results are non-significant.
Q & A
What are the key takeaways from the discussion about statistical significance?
-The key takeaways are: (1) there is nothing magical about alpha equals .05, (2) a p-value alone does not provide enough information, and (3) it is important to report effect sizes and confidence intervals alongside findings.
What is an effect size and why is it important?
-An effect size is a standardized measure of the magnitude of an effect, which allows for an objective evaluation of the size of the effect. It is important because it helps answer the question of whether a treatment has practical usefulness and how large the effect is.
Why is Cohen's D a commonly used measure of effect size for T tests?
-Cohen's D is commonly used because it provides a standardized measure that quantifies the magnitude of the difference between two groups, making it easier to compare the effect sizes across different studies.
How does statistical significance differ from effect size?
-Statistical significance tells us whether the differences between means were not due to chance, while effect size measures the magnitude of the effect. Significance is about the probability of observing the results under the null hypothesis, whereas effect size is about the practical importance of the observed differences.
What is an example given to illustrate the difference between statistical significance and effect size?
-The example involves Smith and Jones conducting studies on two leadership styles. Smith finds a significant result with a T value of 2.21 and a p-value less than .05, while Jones, with a smaller sample size, does not replicate the significance with a T value of 1.06 and a p-value greater than .30. However, both studies have similar effect sizes (Cohen's D of 0.49 and 0.47), indicating that the magnitude of the effect was consistent despite the difference in statistical significance.
What are the conventions for interpreting Cohen's D effect size as small, medium, or large?
-Jacob Cohen provides the following conventions: a small effect size is around 0.2, a medium effect size is around 0.5, and a large effect size is around 0.8. These are based on the probabilities of the overlap between two distributions.
Why might the conventions for interpreting effect sizes be considered arbitrary?
-Jacob Cohen himself acknowledged that all conventions are arbitrary. However, he argued that they should not be unreasonable and should be useful, which in the case of Cohen's D, they are based on probabilities and the overlap of distributions.
What is the purpose of a power analysis in research?
-A power analysis helps determine the number of participants needed in an experiment to detect an effect of a certain size. It ensures that the study is adequately powered to find any real differences that exist and to avoid wasting resources with too few participants.
Why is it recommended to report effect size even for statistically non-significant findings?
-Reporting effect size is recommended because it provides insight into the magnitude of the effect, which can be important even if the results are not statistically significant. This is especially true for small sample sizes where non-significance does not necessarily mean the absence of an effect.
How does sample size influence the relationship between effect size and statistical significance?
-Sample size can greatly influence statistical significance. A larger sample size can lead to statistically significant results even for small effect sizes, while a smaller sample size may result in non-significant findings even if the effect size is large. Reporting effect size helps interpret the results in light of the sample size.
Outlines
π Understanding Statistical Significance and Effect Size
The discussion emphasizes the limitations of relying solely on p-values and the importance of reporting effect sizes and confidence intervals. It clarifies that a p-value does not inherently measure the size or practical significance of an effect. The concept of effect size is introduced as a standardized measure, with Cohen's D being a common metric for T tests. The script contrasts statistical significance, which indicates whether observed differences could be due to chance, with effect size, which quantifies the magnitude of the effect. An example illustrates how two studies with different p-values can have similar effect sizes, highlighting the fallacy of equating non-significance with the absence of an effect. Cohen's conventions for interpreting effect sizes as small, medium, or large are explained, with an emphasis on their arbitrary yet useful nature based on probabilities and distribution overlap.
π Effect Size Conventions and Their Practical Implications
This paragraph delves into the specifics of Cohen's effect size conventions, explaining how different effect sizes correspond to the degree of overlap between two distributions. It provides concrete percentages to illustrate the practical differences between small, medium, and large effect sizes, using relatable examples such as height differences among age groups and IQ differences among occupational levels. The paragraph also introduces expanded categories for very small, very large, and huge effect sizes. The importance of effect size in determining the necessary sample size for a study is highlighted through power analysis, which helps in avoiding underpowered studies and wasted resources. The paragraph emphasizes the direct interpretability of effect sizes and their utility in research, regardless of statistical significance.
π The Importance of Reporting Effect Size in Research
The final paragraph underscores the necessity of reporting effect sizes in research, as recommended by the APA, even for non-significant findings. It argues that effect size provides crucial information about the practical significance of results, which can be obscured by statistical significance alone. The paragraph discusses how effect size can clarify the impact of treatments or interventions, especially in cases of small sample sizes where non-significance might not equate to ineffectiveness. It also points out the influence of sample size on statistical significance and how effect size helps interpret results in context. The summary concludes by advocating for the inclusion of effect size in all research reports to provide a more comprehensive understanding of study outcomes.
Mindmap
Keywords
π‘Statistical Significance
π‘P-Value
π‘Effect Size
π‘Cohen's D
π‘Sample Size
π‘Power Analysis
π‘Null Hypothesis
π‘Confidence Intervals
π‘APA Recommendations
π‘Practical Significance
Highlights
There is nothing magical about alpha equals .05, challenging the traditional threshold for statistical significance.
A p-value alone does not provide sufficient information about the results of a study.
Effect sizes and confidence intervals should be reported alongside findings for a more comprehensive understanding.
Effect size measures the practical significance of an effect, answering the question of its real-world impact.
Cohen's D is the most commonly used measure of effect size for T tests, providing a standardized measure.
Statistical significance and effect size are different; significance indicates whether differences are due to chance.
Making decisions based solely on p-values can be misleading, as illustrated by the example of Smith and Jones' studies.
Even when p-values differ, the effect size can show that the same effect was found, as seen in the comparison of Smith's and Jones's studies.
Jacob Cohen provides conventions for interpreting effect sizes as small, medium, or large, based on probabilities and distribution overlap.
Effect sizes can be categorized as 'very small,' 'small,' 'medium,' 'large,' 'very large,' and 'huge,' expanding on Cohen's original scale.
Knowing the effect size allows for power analysis, helping to determine the necessary sample size for detecting an effect.
The American Psychological Association (APA) recommends including a measure of effect size in all published statistical reports.
Effect size reporting is crucial even when the sample size is small and results are non-significant, as it can indicate real-world impact.
Statistical significance is a function of sample size, and effect size helps interpret the significance in the context of sample size.
Effect size reporting is essential to understand the true impact of findings, regardless of statistical significance.
Effect size can be posited directly for power analysis, without needing to be calculated from previous data.
Transcripts
Browse More Related Video

What Is And How To Calculate Cohen's d?

Introduction to Effect Size
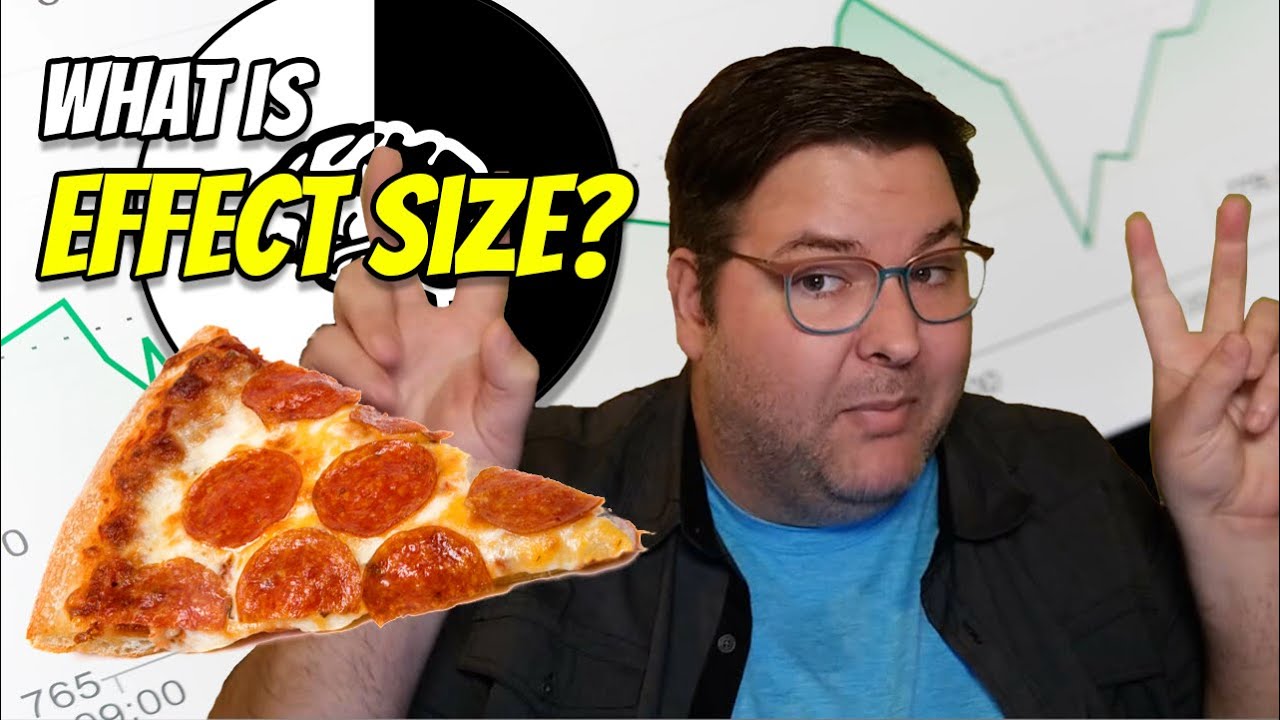
What is Effect Size? Statistical significance vs. Practical significance
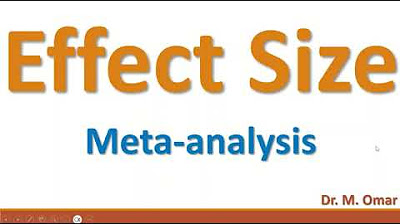
Effect size in meta analysis

How to find Cohen's D to determine the Effect Size Using Pooled Standard Deviation
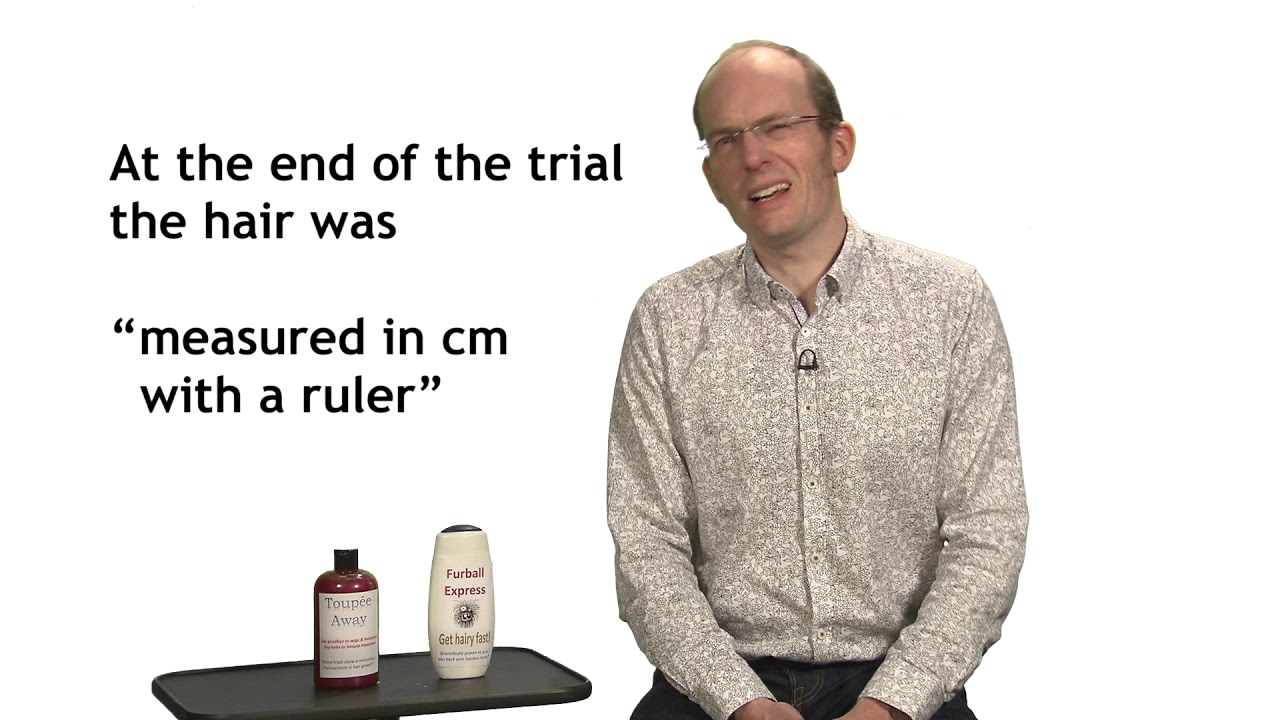
What do my results mean Effect size is not the same as statistical significance. With Tom Reader.
5.0 / 5 (0 votes)
Thanks for rating: