Factors Affecting Power - Effect size, Variability, Sample Size (Module 1 8 7)
TLDRThis script discusses the factors influencing the power of a hypothesis test, focusing on the importance of the alternative hypothesis being true. It explains that power is maximized when there is a significant effect size, as larger effects are easier to detect. The script also highlights the role of population variability and sample size in determining power, using formulas and diagrams to illustrate how less variability and larger sample sizes can lead to more accurate hypothesis testing and a reduced likelihood of missing true effects.
Takeaways
- 🔍 The power of a hypothesis test refers to the probability of correctly rejecting a false null hypothesis when the alternative hypothesis is true.
- 🎯 Power is undefined if there isn't a real effect, as there's no correct way to reject a null hypothesis if it's actually true.
- 📊 The size of the effect is a crucial factor affecting the power of a test; larger effects are easier to detect and thus increase power.
- 📉 The test statistic Z is influenced by the effect size, which is the difference between the means under the alternative and null hypotheses.
- 📈 Increasing the effect size can shift the sampling distribution, reducing the chances of missing a true effect, thereby increasing power.
- 🧩 Variability in the population also affects power; less variability leads to tighter sampling distributions and higher power.
- 📊 Sigma sub x-bar, the standard error of the sampling distributions, is composed of the population standard deviation divided by the square root of the sample size.
- 🔍 If the population is less variable, sample means are closer to the population mean, making it harder to get a sample mean far from the null hypothesis mean.
- 📈 Increasing the sample size draws in the sampling distributions, making it more likely that sample means from the alternative hypothesis will exceed the critical value and increase power.
- 🔢 Reducing population variability or increasing sample size are ways to increase power, with sample size being more controllable and thus a primary method to enhance power.
- ∞ With infinitely large samples, the sample mean is expected to be very close to the population mean, making it nearly impossible to confuse a sample mean from the alternative hypothesis with a chance occurrence under the null hypothesis.
Q & A
What is the concept of 'power' in the context of hypothesis testing?
-In hypothesis testing, 'power' refers to the probability that a test will correctly reject a false null hypothesis when the alternative hypothesis is true. It's a measure of the test's ability to detect an effect when there is one.
Why is power undefined if there isn't a real effect?
-Power is undefined without a real effect because there's no correct way to reject a false null hypothesis if the null hypothesis is actually true. The concept of power is tied to the existence of an effect that the test is designed to detect.
What is the most intuitive factor affecting the power of a hypothesis test?
-The most intuitive factor affecting the power of a hypothesis test is the size of the effect. A larger effect is easier to detect, thus increasing the power of the test.
How does the size of the effect influence the power of a hypothesis test?
-The size of the effect influences the power by affecting the numerator of the test statistic (Z sub X bar), which is the difference between the sample mean under the alternative hypothesis and the mean under the null hypothesis. A larger effect size leads to a larger test statistic, increasing the power of the test.
What is the relationship between the variability in the population and the power of a hypothesis test?
-The variability in the population affects the power of a hypothesis test through the standard error (sigma sub x-bar), which is the denominator of the test statistic. Less variability in the population leads to a smaller standard error, which in turn increases the power of the test.
How does the sample size impact the power of a hypothesis test?
-The sample size impacts the power of a hypothesis test by affecting the standard error of the sampling distribution. A larger sample size reduces the standard error, which increases the test statistic's value and thus the power of the test.
What is the role of the sample mean in hypothesis testing?
-The sample mean plays a crucial role in hypothesis testing as it estimates the population mean under the alternative hypothesis. It is used to determine whether the observed effect is likely due to chance or represents a real effect.
What does the term 'beta region' refer to in the context of hypothesis testing?
-The 'beta region' in hypothesis testing refers to the probability of a Type II error, which is the likelihood of failing to reject a false null hypothesis when the alternative hypothesis is true.
How does increasing the effect size change the power of a hypothesis test?
-Increasing the effect size shifts the sampling distribution under the alternative hypothesis further away from the mean under the null hypothesis, reducing the beta region and increasing the power of the test to detect the effect.
What is the impact of reducing population variability on the power of a hypothesis test?
-Reducing population variability narrows the sampling distribution, making it more likely that sample means will be close to the population mean under the alternative hypothesis. This increases the power of the test because it becomes less likely to confuse a sample mean from the alternative population with one from the null population.
Can you always increase the power of a hypothesis test by increasing the sample size?
-Yes, increasing the sample size is one of the main ways to increase the power of a hypothesis test. A larger sample size reduces the standard error, leading to a larger test statistic and a higher likelihood of correctly rejecting a false null hypothesis.
Outlines
🔍 Understanding Hypothesis Test Power
The first paragraph discusses the concept of power in hypothesis testing, emphasizing that power is about the ability to correctly reject a false null hypothesis when the alternative hypothesis is true. It highlights the importance of effect size in determining power, illustrating how larger effects are easier to detect. The paragraph also explains the role of the sample mean and population mean under the null and alternative hypotheses in calculating the test statistic (Z score). Increasing the effect size, as shown in the script, increases the likelihood of correctly rejecting the null hypothesis, thereby increasing power. However, even with large effects, there's a chance of missing the effect due to sampling error.
📊 Variability and Sample Size in Power Analysis
The second paragraph delves into additional factors that affect the power of a hypothesis test, namely the variability within the population and the sample size. It explains that less variability in the population leads to sampling distributions that are more tightly clustered around the population mean, making it easier to detect a true effect. The paragraph also discusses how increasing the sample size narrows the sampling distribution, which increases the likelihood that the sample mean will be close to the population mean under the alternative hypothesis. This, in turn, increases the power of the test. The summary underscores that while reducing population variability is challenging, increasing sample size is a practical way to boost power.
Mindmap
Keywords
💡Hypothesis Test
💡Power
💡Alternative Hypothesis
💡Effect Size
💡Z-score
💡Standard Error
💡Sample Size
💡Population Variability
💡Beta (β)
💡Type I and Type II Errors
Highlights
The power of a hypothesis test is affected by the size of the effect and the alternative hypothesis being true.
Power is undefined if there isn't a real effect, meaning no way to correctly reject a false null hypothesis if the null hypothesis is true.
Effect size is intuitively the most impactful factor on power, as larger effects are easier to detect.
The formula for the size of the effect is X bar minus mu sub X bar, where X bar estimates the mean under the alternative hypothesis.
Increasing the effect size shifts the distribution and reduces miss rates, thus increasing power.
Even with large effect sizes, there is still a chance of missing the effect due to sampling error.
Population variability is another factor affecting power, with less variability leading to sample means closer to the population mean.
The standard error of the sampling distributions, sigma sub x-bar, is derived from the population standard deviation and sample size.
Less variability in the population results in sampling distributions that are less variable and more likely to exceed the critical value.
Increasing sample size is a primary method to increase power, as larger samples provide more consistent estimates of the population mean.
With large sample sizes, the sample mean is likely to be closer to the population mean, making it harder to confuse with a chance occurrence.
Reducing population variability is difficult, but better measurement systems can help.
The consistency of a statistic increases with larger sample sizes, making it more reliable.
In large samples, the sample mean is expected to be very close to the true mean, reducing the likelihood of Type I and Type II errors.
The relationship between sample size, effect size, and population variability is crucial for understanding the power of a hypothesis test.
Power analysis is essential for designing experiments and interpreting the results in the context of the population.
Understanding the factors that affect power helps in making informed decisions about sample size and expected outcomes.
Transcripts
Browse More Related Video
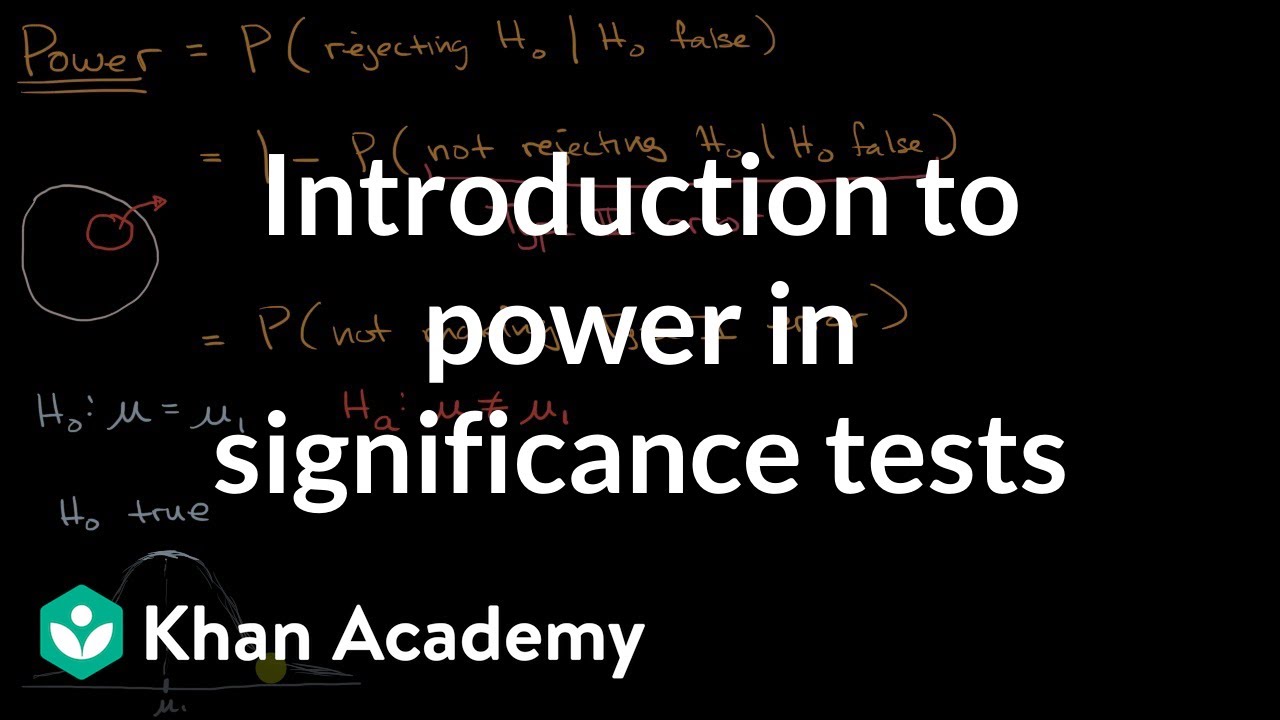
Introduction to power in significance tests | AP Statistics | Khan Academy
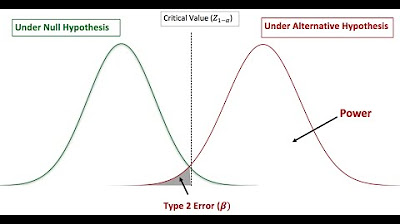
Power and Sample Size Calculation
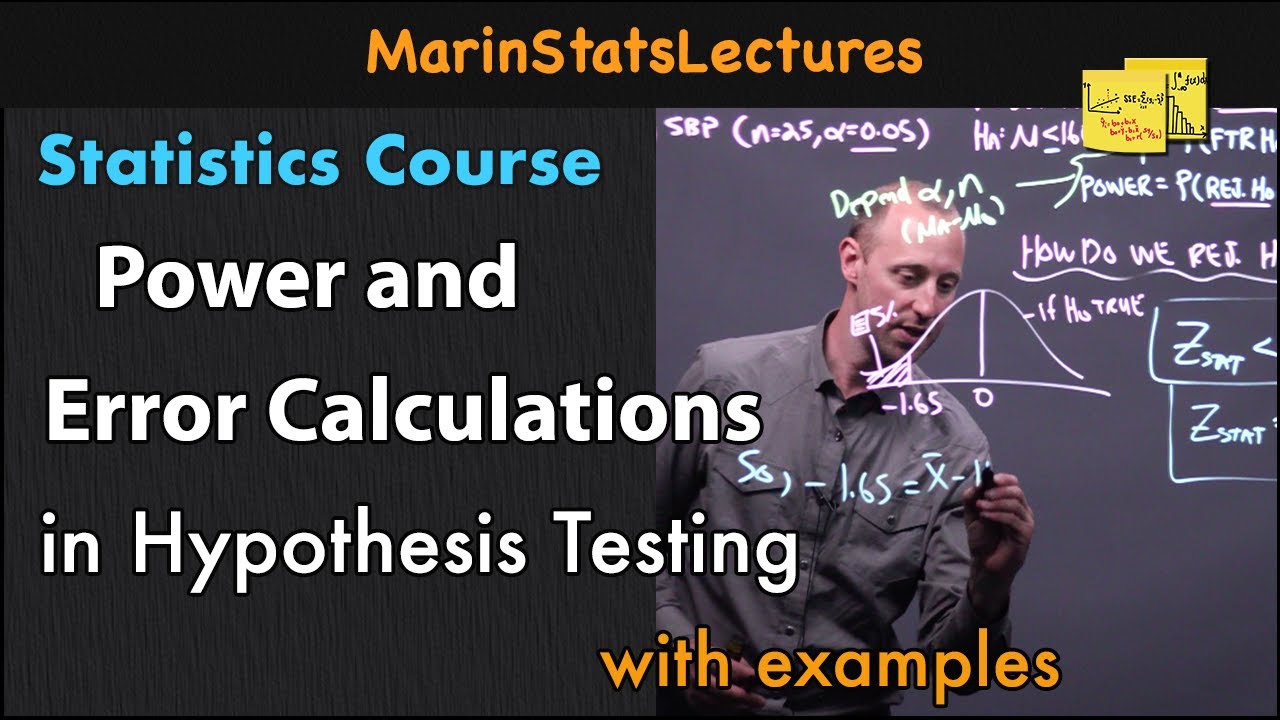
Power Calculations in Hypothesis Testing | Statistics Tutorial #17 | MarinStatsLectures
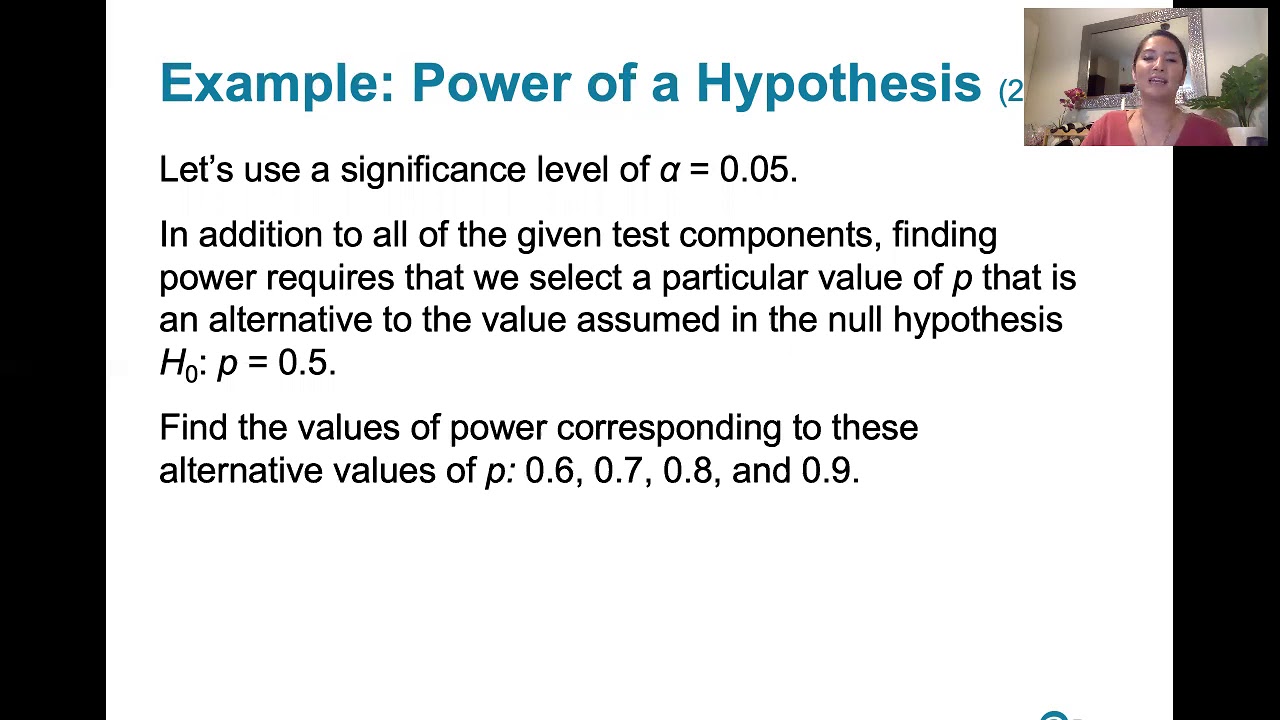
8.1.6 Basics of Hypothesis Testing - Power of a Hypothesis Test
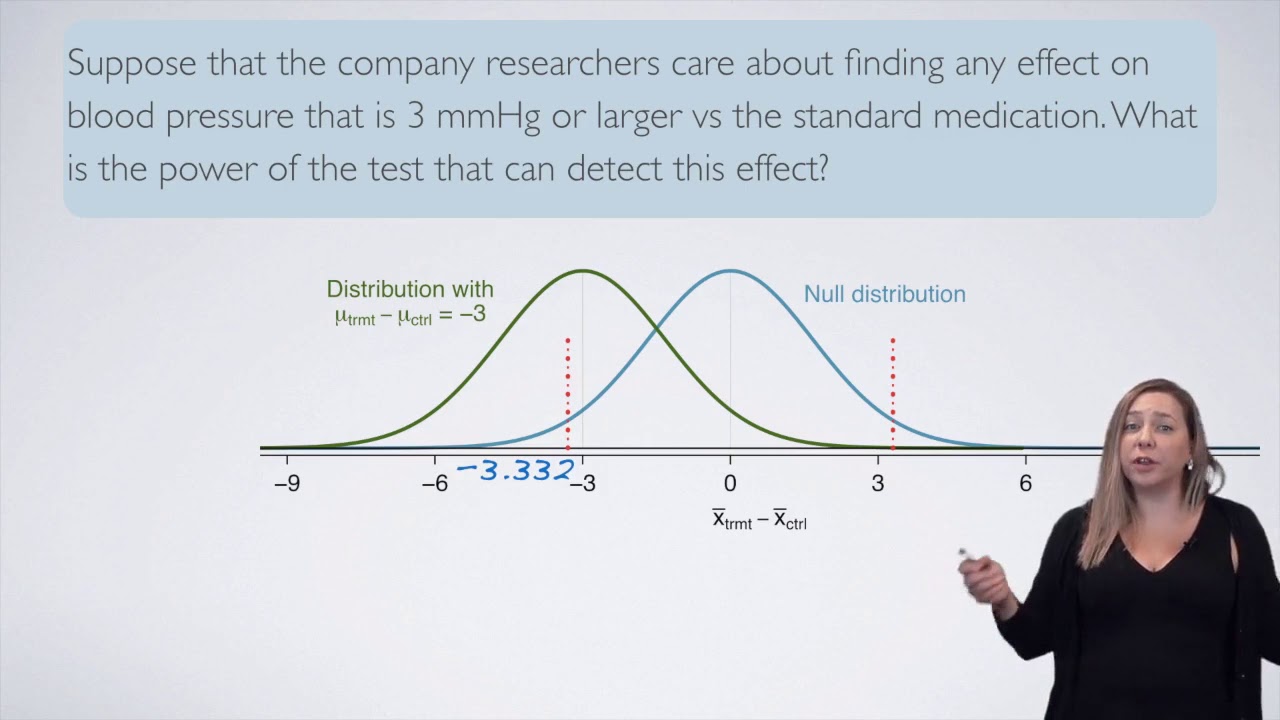
5 4 Power Calculations for difference of two means
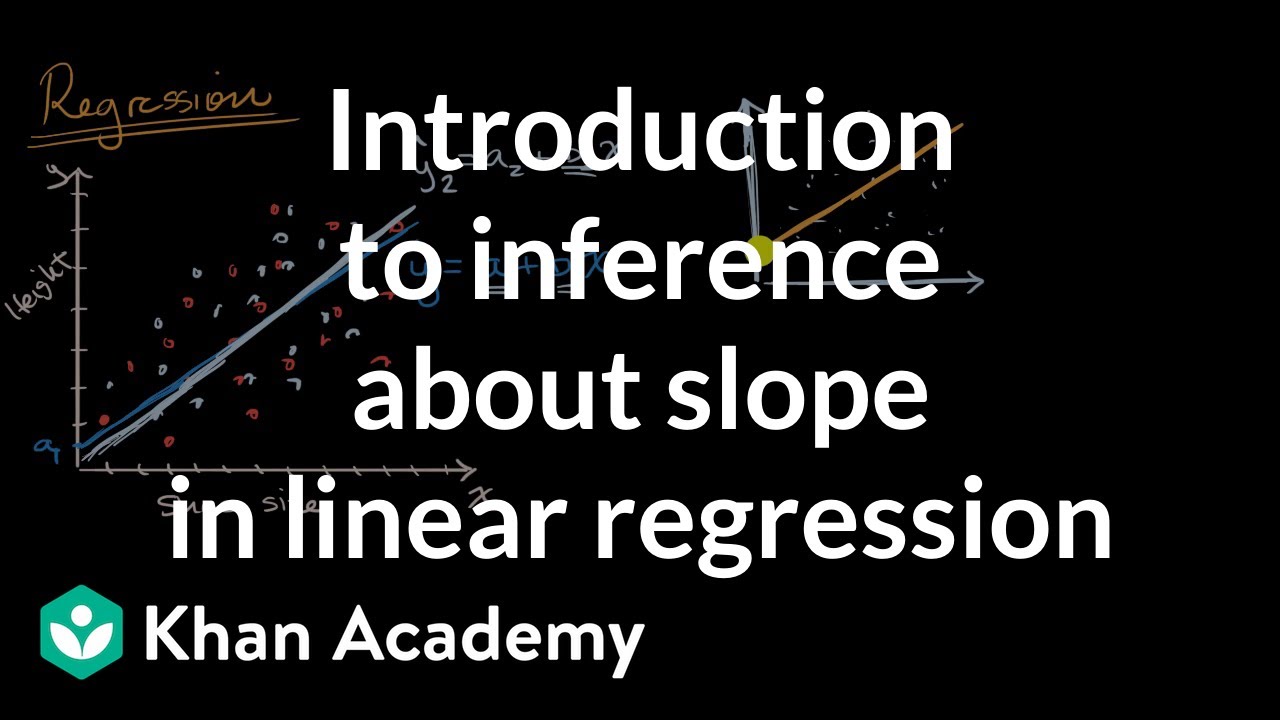
Introduction to inference about slope in linear regression | AP Statistics | Khan Academy
5.0 / 5 (0 votes)
Thanks for rating: