What is Homoscedasticity and Heteroscedasticity and how to check it using SPSS?
TLDRThis video delves into the concept of homoscedasticity, a crucial assumption in regression analysis. It explains that homoscedasticity refers to the equal distribution of residual values, contrasting it with heteroscedasticity where residuals cluster or spread unevenly. The presenter uses a sales example to illustrate both scenarios, guiding viewers through a step-by-step regression analysis and charting process. By comparing standardized predicted values with standardized residual values, the video effectively demonstrates how to identify homoscedasticity and its importance in ensuring the validity of regression models.
Takeaways
- π Homoscedasticity is an assumption in regression analysis that refers to the equal distribution of residual values.
- π Residual values are the error terms, which are the differences between observed and predicted values of the dependent variable.
- π The video demonstrates how to perform regression analysis and visualize the distribution of residual values using a sales example.
- π To check for homoscedasticity, one should plot the standardized predicted values (z-pred) on the x-axis and standardized residual values (z-resid) on the y-axis.
- π In a homoscedastic condition, the residual values are uniformly distributed without forming any clusters.
- π« Heterosedasticity occurs when residual values cluster at some values and spread apart at others, indicating non-equal distribution.
- π The video contrasts homoscedasticity with a heterosedasticity example, showing a triangular shape of the residual distribution from left to right.
- π Homoscedasticity is preferred in regression analysis as it aligns with the assumption of equal variance of errors.
- π The script emphasizes the importance of checking for homoscedasticity to ensure the validity of regression analysis results.
- π Understanding the distribution of residuals is crucial for diagnosing potential issues in regression models and interpreting the results accurately.
Q & A
What is homoscedasticity?
-Homoscedasticity is an assumption in regression analysis that refers to the residuals (error terms) of the dependent variable being equally distributed, rather than clustering together at some values or spreading apart at others.
What are residual values in the context of regression analysis?
-Residual values are the differences between the observed values and the predicted values of the dependent variable in a regression analysis.
What is the opposite of homoscedasticity?
-The opposite of homoscedasticity is heterosedasticity, where the residual values do not have an equal distribution but tend to cluster at some values and spread apart at others.
Why is it important to check for homoscedasticity in regression analysis?
-Checking for homoscedasticity is important because it is an assumption of regression analysis that ensures the validity of the model. If the assumption is violated, the standard errors of the regression coefficients may be inaccurate, leading to misleading inferences.
How can you visually assess homoscedasticity using a chart?
-You can visually assess homoscedasticity by plotting the standardized predicted values on the x-axis and the standardized residual values on the y-axis. If the residuals are uniformly distributed without forming clusters, it indicates homoscedasticity.
What does a triangular shape in the residual distribution chart suggest about the homoscedasticity of the model?
-A triangular shape in the residual distribution chart, where values cluster on the left and spread out as you move to the right, suggests heterosedasticity, indicating that the model does not have homoscedasticity.
In the provided script, what variables are used in the sales example for regression analysis?
-In the sales example provided in the script, the independent variable is 'experience' and the dependent variable is 'sales'.
How can you generate a chart for residual variable distribution in a regression analysis?
-To generate a chart for residual variable distribution, you can use statistical software to perform regression analysis, then select 'plots' and choose 'zpred' as the x-axis and 'zresid' as the y-axis, which represent standardized predicted and residual values, respectively.
What does the script suggest about the relationship between the standardized predicted values and standardized residual values in a homoscedastic model?
-The script suggests that in a homoscedastic model, the standardized residual values are uniformly distributed across the standardized predicted values, indicating no pattern or clustering in the residuals.
What is the purpose of standardizing predicted and residual values in regression analysis?
-Standardizing predicted and residual values in regression analysis helps to normalize the data, making it easier to compare and visualize the distribution of residuals across different ranges of predicted values.
How does the script summarize the conditions for homoscedasticity and heterosedasticity?
-The script summarizes that in the case of homoscedasticity, residual values are equally distributed, whereas in heterosedasticity, the residual values are not equally distributed and tend to cluster or spread out in a pattern.
Outlines
π Understanding Homoscedasticity and Heteroscedasticity in Regression Analysis
This paragraph introduces the concept of homoscedasticity, an important assumption in regression analysis. Homoscedasticity refers to the equal distribution of residual values, which are the differences between observed and predicted values of the dependent variable. The speaker explains that if residuals are uniformly distributed without forming clusters, this indicates homoscedasticity. Conversely, if residuals cluster at certain values and spread apart at others, this is known as heteroscedasticity. The paragraph uses a sales example to illustrate these concepts, where experience is the independent variable and sales are the dependent variable. The speaker guides through the process of conducting regression analysis and plotting the distribution of residuals to visually assess homoscedasticity. The paragraph concludes with a visual representation of homoscedasticity, where the residuals are uniformly distributed across the chart.
π Comparing Homoscedasticity and Heteroscedasticity with Sales Data
Building upon the previous explanation, this paragraph further explores the concepts of homoscedasticity and heteroscedasticity using another sales example. The speaker describes the process of conducting regression analysis for a different product's sales data, again using experience as the independent variable. The aim is to observe the distribution of residuals to determine if the dependent variable exhibits homoscedasticity or heteroscedasticity. The speaker instructs on how to plot the residuals against standardized predicted values to visually assess the distribution. The paragraph concludes with the observation of a triangular-shaped distribution of residuals, indicating heteroscedasticity, where the residuals cluster on the left side and scatter as they move to the right, contrasting with the uniform distribution seen in homoscedasticity.
Mindmap
Keywords
π‘Homoscedasticity
π‘Residual Values
π‘Regression Analysis
π‘Dependent Variable
π‘Independent Variable
π‘Heteroscedasticity
π‘Standardized Predicted Variable
π‘Standardized Residual Variable
π‘Distribution
π‘Z-Score
Highlights
Homoscedasticity is an important assumption in regression analysis.
Residual values are the error terms, representing the difference between observed and predicted values.
Homoscedasticity refers to the equal distribution of residual values.
Heteroscedasticity is when residual values cluster at some values and spread apart at others.
In regression analysis, it's important to check for homoscedasticity or heteroscedasticity in the dependent variable.
An example is provided using sales data, with experience as the independent variable and sales as the dependent variable.
To analyze regression and draw the residual variable distribution chart, specific steps in a statistical software are outlined.
Standardized predicted values (z-pred) and standardized residual values (z-resid) are used for the analysis.
Homoscedasticity is indicated by a uniform distribution of standardized residual values.
Heteroscedasticity is shown when the distribution of residuals takes a triangular shape, clustering on the left and scattering to the right.
The video demonstrates how to identify and differentiate between homoscedasticity and heteroscedasticity through visual inspection of the residual plot.
The assumption of homoscedasticity in regression analysis is crucial for the validity of the model.
The video provides a clear distinction between the two conditions through visual examples.
Understanding the distribution of residuals is key to assessing the quality of a regression model.
The video emphasizes the importance of equal distribution in homoscedasticity for reliable regression analysis.
Heteroscedasticity can lead to underestimation or overestimation of the true variability in the data.
The video concludes with a summary reinforcing the definitions and implications of homoscedasticity and heteroscedasticity.
Transcripts
Browse More Related Video
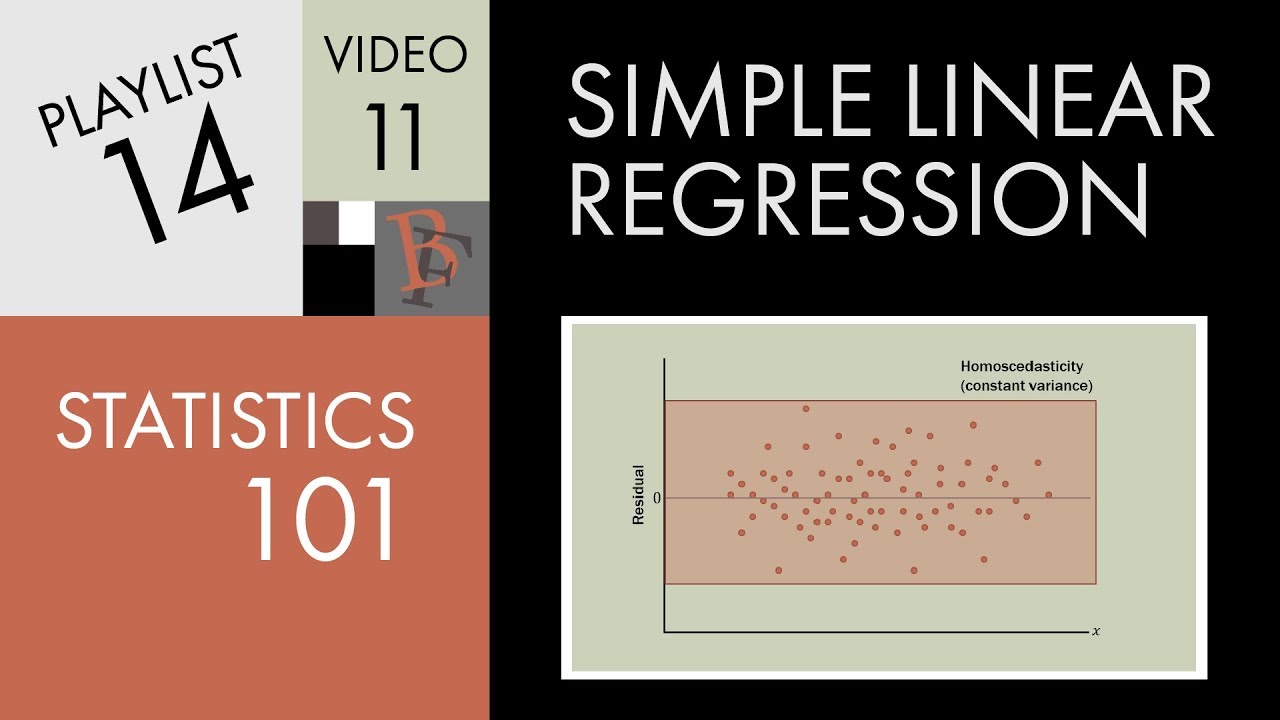
Statistics 101: Linear Regression, Residual Analysis

10.2.6 Regression - Residual Plots and Their Interpretation
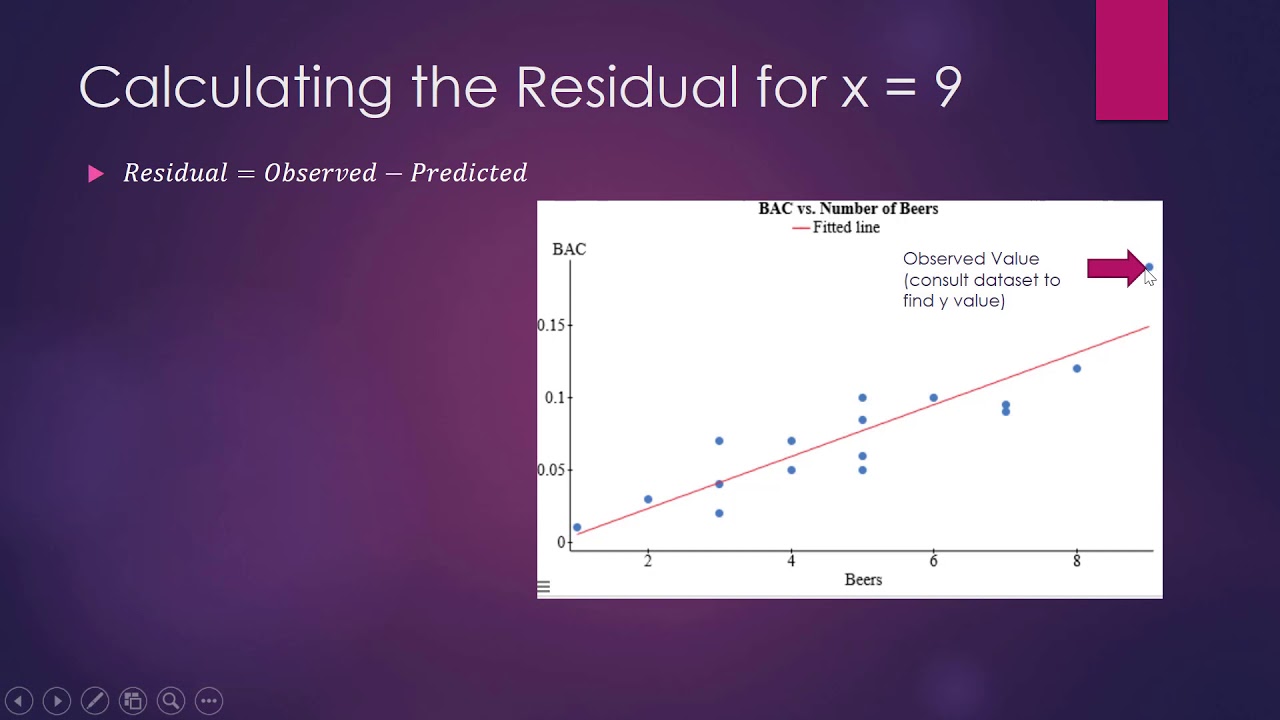
How to Calculate the Residual
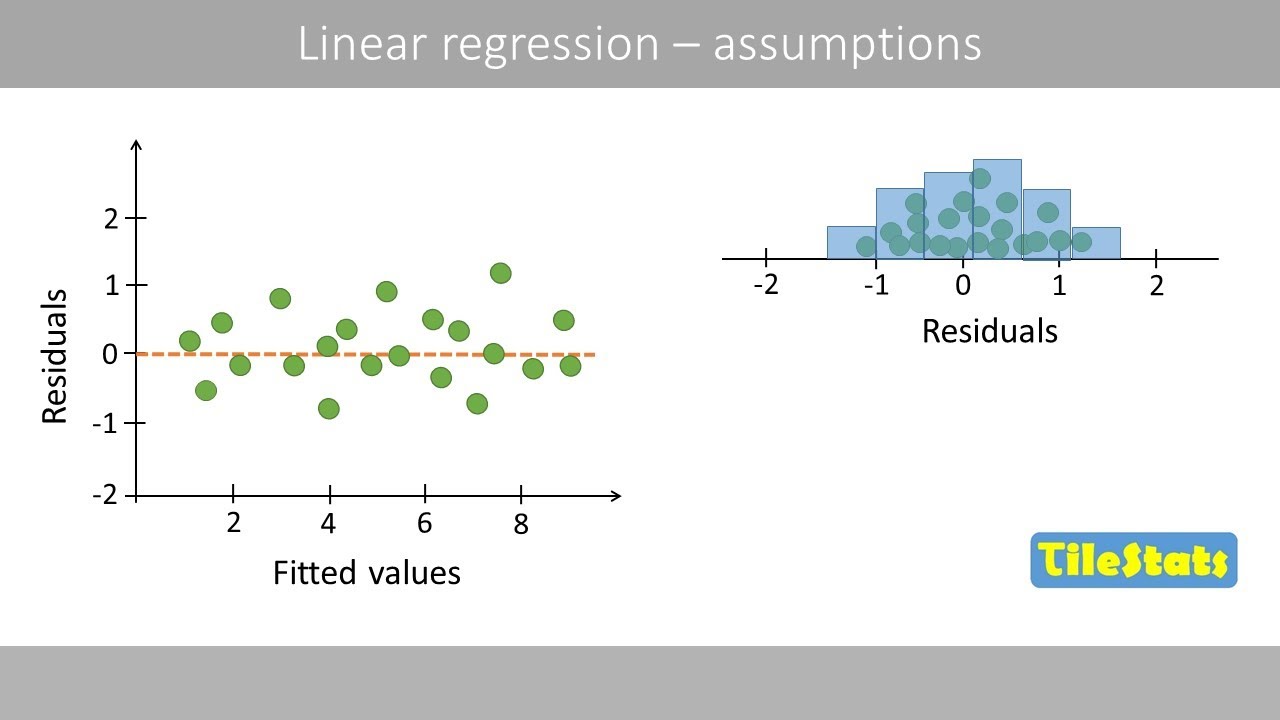
Assumptions in Linear Regression - explained | residual analysis

Calculating Residuals & Making Residual Plots on TI-84 Plus

Residual plots | Exploring bivariate numerical data | AP Statistics | Khan Academy
5.0 / 5 (0 votes)
Thanks for rating: