Calculus BC β 10.12 Lagrange Error Bound
TLDRIn this engaging calculus lesson, Mr. Bean introduces the concept of Lagrange Error Bound, which quantifies the discrepancy between a Taylor polynomial approximation and the actual function value. By leveraging the Taylor polynomial and the remainder term, the lecture elucidates how to estimate the maximum possible error. Through illustrative examples involving cosine and exponential functions, the video demonstrates the application of the Lagrange Error Bound in determining the precision of approximations, ultimately enhancing the understanding of series and polynomials in calculus.
Takeaways
- π The Lagrange error bound is a concept in calculus that measures the maximum possible error between a Taylor polynomial approximation and the actual function value.
- π Taylor polynomials are used to approximate functions, and understanding their construction is prerequisite to learning about error bounds.
- π The exact value of a function can be represented as the sum of a Taylor polynomial and a remainder term, which accounts for the difference between the approximation and the true value.
- π± The Lagrange error bound provides a way to calculate the maximum error of an approximation, helping to understand the accuracy of Taylor polynomials.
- π¬ Understanding and applying the Lagrange error bound involves knowing the (n+1)th derivative of the function being approximated and finding the maximum value of this derivative over a specific interval.
- π Examples used in the script include approximating functions like cosine and the exponential function, illustrating the process of calculating error bounds.
- π§ The script emphasizes the importance of absolute values in the context of error calculation, ensuring that the error is always represented as a positive quantity.
- π The remainder term in the approximation formula is crucial for understanding the concept of error in the context of Taylor polynomials.
- π² The process of finding the maximum value of the (n+1)th derivative over an interval is central to determining the Lagrange error bound.
- π The choice of the interval and the value 'c' (or 'z' in the context of the script) plays a significant role in calculating the error bound.
Q & A
What is the main topic of this calculus lesson?
-The main topic of this lesson is the Lagrange Error Bound, which is used to determine how close an approximation (like a Taylor polynomial) is to the actual function value.
What does the Lagrange Error Bound represent?
-The Lagrange Error Bound represents the maximum possible error between the approximate value given by a Taylor polynomial and the actual value of the function.
How is the remainder term in the Taylor polynomial expression defined?
-The remainder term is defined as the difference between the exact function value and the value of the Taylor polynomial approximation at the same point.
What is the formula for the Lagrange Error Bound?
-The Lagrange Error Bound is given by the formula: |R_n(x)| β€ (f^(n+1)(c) * (x - c)^(n+1)) / (n+1)!, where f^(n+1)(c) is the (n+1)th derivative of the function evaluated at some c between the interval points, and n is the degree of the Taylor polynomial.
What is the significance of the nth derivative in the Lagrange Error Bound formula?
-The nth derivative in the Lagrange Error Bound formula is significant because it helps determine the maximum possible error. It represents the behavior of the function near the interval points, and its magnitude can affect the size of the error bound.
How does the value of 'c' in the Lagrange Error Bound formula affect the error bound?
-The value of 'c' in the Lagrange Error Bound formula represents the point about which the Taylor polynomial is centered (usually 'a' in the case of a Maclaurin polynomial). The choice of 'c' can affect the magnitude of the error bound since it influences the (n+1)th derivative's value and the interval over which it is evaluated.
What is a Taylor polynomial?
-A Taylor polynomial is an approximation of a function using a polynomial constructed from the function's derivatives at a given point. It is used to estimate the function's value at other points near the point of interest.
What is the role of the factorial term in the denominator of the Lagrange Error Bound formula?
-The factorial term in the denominator of the Lagrange Error Bound formula, (n+1)!, is a scaling factor that ensures the error bound is properly adjusted for the degree of the Taylor polynomial being used. It increases rapidly with 'n', which helps to control the size of the error bound as the degree of the polynomial increases.
How does the Lagrange Error Bound help in practical applications?
-The Lagrange Error Bound provides a way to estimate the reliability of a Taylor polynomial approximation. It helps in determining how close the approximation is likely to be to the actual function value, which is crucial for ensuring the accuracy of calculations in various applications.
What is the process for finding the smallest order of a Taylor polynomial given a Lagrange Error Bound?
-To find the smallest order of a Taylor polynomial given a Lagrange Error Bound, you start with the lowest order and calculate the error bound for each successive order until the error bound is less than the specified limit (usually 1). The last order for which the error bound is still greater than the limit is not the correct order; you need to use the next higher order.
How can the Lagrange Error Bound be used to improve the accuracy of a Taylor polynomial approximation?
-By using the Lagrange Error Bound, you can determine the necessary degree of the Taylor polynomial to achieve a desired level of accuracy. If the error bound is larger than acceptable, you can increase the degree of the polynomial to reduce the error and improve the approximation's accuracy.
Outlines
π Introduction to Lagrange Error Bound
This paragraph introduces the concept of the Lagrange Error Bound in the context of calculus. It explains that the error bound is a measure of how far an approximation, such as a Taylor polynomial, is from the actual function value. The discussion begins with a review of Taylor polynomials and their role as approximations of functions. The focus then shifts to the error between the approximation and the true function value, aiming to determine the maximum possible error. The explanation is set to simplify the concept by relating it to previously covered topics, setting the stage for a deeper dive into the Lagrange Error Bound.
π’ Applying Lagrange Error Bound to Cosine Approximation
In this paragraph, the application of the Lagrange Error Bound is demonstrated using the approximation of the cosine function at x=0.2. The speaker calculates the approximation using the fourth-degree Maclaurin polynomial and then determines the error bound. The process involves finding the maximum value of the fifth derivative of the function over the interval from 0 to 0.2 and applying it to the Lagrange Error Bound formula. The result is a bound of 2.667 x 10^(-6), indicating that the approximation is extremely close to the actual value of cosine at x=0.2.
π Example: Error Bound for e^x at x=1
This section presents an example of calculating the Lagrange Error Bound for the function e^x at x=1 using a third-degree Taylor polynomial. The speaker first shows the approximation of e^x by plugging in x=1 into the Taylor polynomial, resulting in an approximation of 2.6667. The error bound is then calculated by considering the maximum value of the fourth derivative of e^x (which is e^x) over the interval from 0 to 1. The bound is found to be e^1 * (1^4 / 4!), which simplifies to 1/4!. The actual error is then compared to the error bound, showing that the actual error is smaller than the bound, confirming the effectiveness of the Lagrange Error Bound in estimating the maximum possible error.
π Determining the Smallest Order for a Given Error Bound
The final paragraph discusses a reverse application of the Lagrange Error Bound, where the goal is to find the smallest order of a Taylor polynomial that results in an error less than a given threshold. Using the function e^(x-1) as an example, the speaker outlines the process of determining the order of the Taylor polynomial by testing different values of n and calculating the corresponding error bounds. The process involves finding the value of n for which the error bound first drops below the given threshold (in this case, less than 1). The example concludes with the determination that a fifth-degree Taylor polynomial is the smallest order that satisfies the error bound condition.
Mindmap
Keywords
π‘Lagrange Error Bound
π‘Taylor Polynomial
π‘Derivative
π‘Maclaurin Polynomial
π‘Approximation
π‘Error Analysis
π‘nth Degree
π‘Remainder
π‘Interval
π‘Factorial
Highlights
Introduction to Lagrange Error Bound in the context of calculus.
Connection between Lagrange Error Bound and previously discussed alternating series error bound.
Explanation of Taylor polynomials as approximations of functions and the concept of error between approximation and actual values.
Definition of the remainder term in the context of Taylor polynomials.
Discussion on the absolute value of the error and its representation as the difference between the actual function and the Taylor polynomial.
Introduction to the concept of finding the maximum possible error using Lagrange Error Bound.
Explanation of the n plus one derivative and its role in determining the maximum error.
Use of a variable 'z' to represent a value between 'c' and 'x' for finding the maximum of the n plus one derivative.
Derivation of the Lagrange Error Bound formula and its components.
Example of approximating cosine of 0.2 using a fourth-degree Maclaurin polynomial.
Calculation of the Lagrange Error Bound for approximating cosine of 0.2 and its significance.
Explanation of the maximum value of a sine or cosine function being one, simplifying the error bound calculation.
Application of the Lagrange Error Bound to approximate e to the power of 1 using a third-degree Taylor polynomial.
Demonstration of how the actual error can be smaller than the error bound, providing a safe estimate of the approximation's accuracy.
Procedure for determining the smallest order of a Taylor polynomial given a Lagrange Error Bound less than one.
Use of a table of values to find the smallest order of a Taylor polynomial that meets the error bound requirement.
Conclusion on the practical application of Lagrange Error Bound in understanding the accuracy of Taylor polynomial approximations.
Transcripts
Browse More Related Video
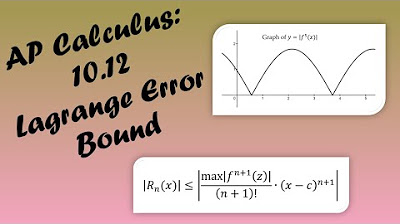
AP Calculus BC Lesson 10.12
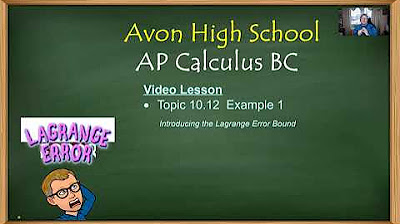
Avon High School - AP Calculus BC - Topic 10.12 - Example 1

Avon High School - AP Calculus BC - Topic 10.12 - Example 3
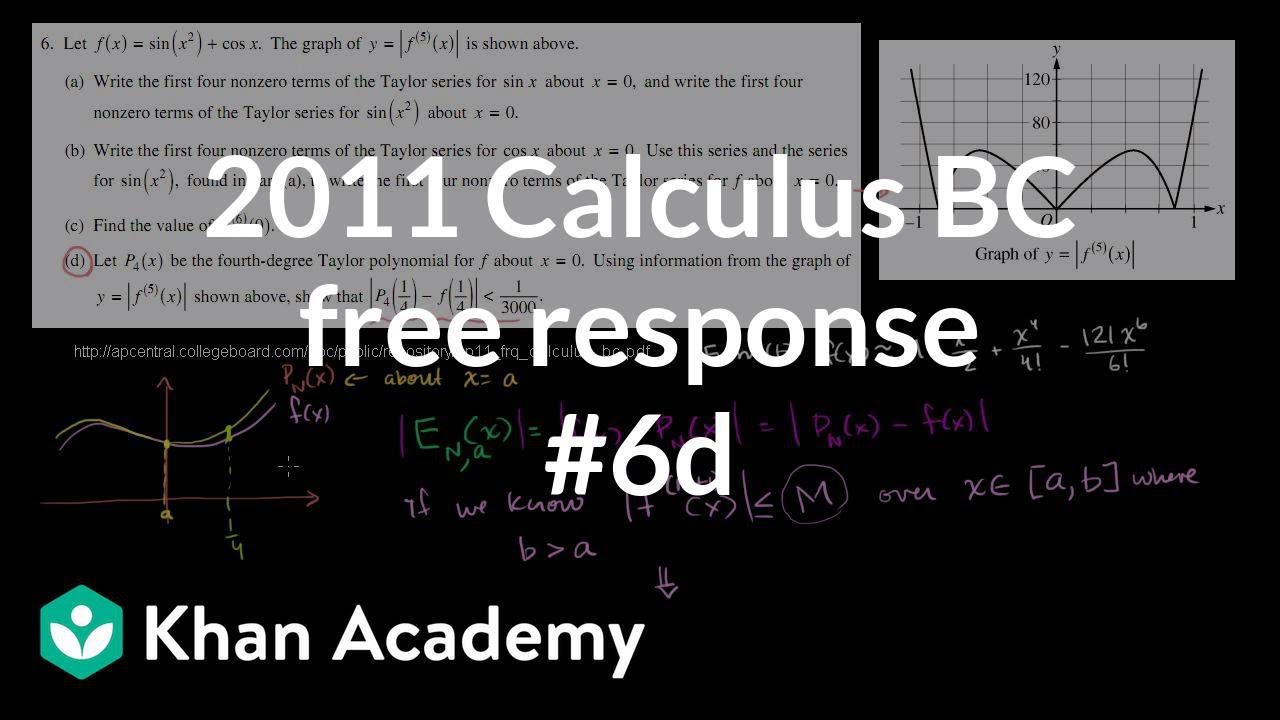
2011 Calculus BC free response #6d | AP Calculus BC | Khan Academy
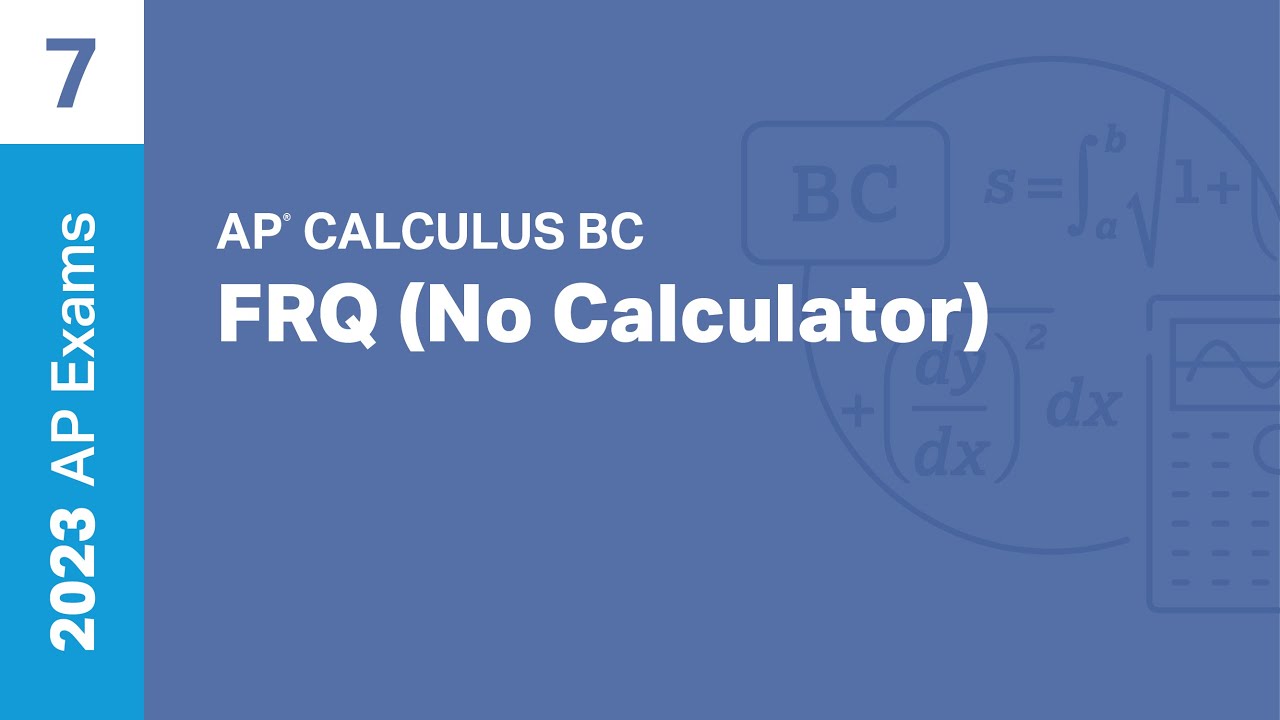
7 | FRQ (No Calculator) | Practice Sessions | AP Calculus BC

Avon High School - AP Calculus BC - Topic 10.12 - Example 2
5.0 / 5 (0 votes)
Thanks for rating: