Bayes in Science and Everyday Life: Crash Course Statistics #25
TLDRThis video explains Bayesian inference, a statistical method of using prior beliefs and new evidence to update probabilities and make decisions. Examples show how Bayes' theorem is applied in business, science, and daily life to determine the likelihood of events and test hypotheses. The math of Bayes with continuous variables is more complex but allows richer updating of beliefs. Examples demonstrate Bayesian A/B testing for marketing emails and evaluating memory differences in dissociative identity disorder patients. Overall, Bayesian methods leverage both data and expertise to produce actionable results in translation, recommendations, medicine, and more.
Takeaways
- ๐ Bayesian methods help update beliefs by combining prior knowledge with new evidence
- ๐ Businesses use Bayesian analysis to make decisions, like testing which emails get more clicks
- ๐ With continuous Bayesian analysis we update probability distributions instead of discrete probabilities
- ๐ค Bayesian analysis quantified evidence that people with Dissociative Identity Disorder may have distinct memory between personalities
- ๐ฎ Bayes' theorem works the same way for continuous variables, but the math gets more complicated
- ๐ Businesses create probability distributions for metrics like click rates based on past data and experience
- โ๏ธ Bayes factors quantify the update from prior belief to posterior - in the DID study this was 4,000!
- ๐ฏ Bayesian methods are used behind the scenes for translation, recommendations, medicine and more
- โ๏ธ Before seeing data, all probabilities for the coin flip were seen as equally likely
- ๐ก The Bayesian calculation matched our intuition - extreme probabilities became less likely after seeing data
Q & A
What is Bayesian hypothesis testing?
-Bayesian hypothesis testing, also called Bayesian inference, is a method of using evidence and prior beliefs to update beliefs about the world. It allows us to quantify and update beliefs involving continuous variables.
How did computational limitations impact the use of Bayesian methods in the 20th century?
-Lack of computational power limited scientists' and statisticians' ability to use Bayesian methods for much of the 20th century. The calculations involved were too complex to do by hand.
What is a Bayes factor?
-A Bayes factor is the ratio of the probabilities of the data under two different models or hypotheses. It quantifies how much the evidence supports one model over another.
How did the business example update beliefs about email click rates?
-The business example used Bayesian inference to update prior beliefs about email click rates with new data from an A/B test. This allowed them to quantify the probability that adding a picture increases click rate.
How did researchers use Bayes to study memory in Dissociative Identity Disorder?
-Researchers used Bayesian methods to quantify how much the data supported the hypothesis that DID patients, people who did not see the materials, and people pretending not to see the materials were three distinct groups in terms of memory.
What are some examples of Bayesian methods being used today?
-Bayesian methods are used today for machine translation between languages, product recommendations, personalized medicine, and artificial intelligence applications like speech recognition.
What is a posterior distribution?
-A posterior distribution represents your updated beliefs after seeing new evidence and incorporating your prior beliefs. It combines the prior distribution and likelihood.
How did Bayes help update beliefs about the coin flip example?
-Bayes' theorem updated the prior uniform distribution using the likelihood of the data (1 out of 5 tails). This ruled out probabilities very close 0 and 1 as unlikely and made probabilities around 0.1-0.5 more likely.
What are some benefits of Bayesian analyses?
-Bayesian analyses allow you to incorporate expert opinion and prior knowledge alongside data. This can lead to better, more efficient decision making with limited data.
How was Bayes' theorem adapted for continuous variables?
-Bayes' theorem for continuous variables uses probability distributions rather than simple probabilities. The math is more complex but the general framework of updating prior beliefs with evidence is the same.
Outlines
๐ฅ Introducing Bayesian Statistics
Adriene Hill introduces Bayesian statistics and hypothesis testing. She explains how we use evidence and experience to update our beliefs about the world. Bayesian methods help companies make business decisions and help researchers quantify results.
๐ Using Bayes with Continuous Variables
Bayes' Theorem can be used with continuous variables, not just discrete ones. The math is more complicated but computers now make it possible. An example is given testing if a coin is biased based on flipping it 5 times and getting 1 tail.
๐ง Business Use Case: Email Click Rates
A beauty blogger example shows Bayesian inference in business decisions. Testing if adding a picture to emails increases click rate. Prior belief of 30% click rate is updated after sending 2 email types. Simulations determine if picture email is better.
๐ฌ Scientific Use Case: Memory in DID Patients
A study on Dissociative Identity Disorder patients learns materials in one personality, then switches to test memory retention. Bayes Factor analysis shows the DID group is quite distinct from groups that did or pretended not to see materials.
๐ค Bayes in Everyday Life
Bayesian methods used in language translation, purchase recommendations, medicine reactions, and artificial intelligence. Bayesian inference increasing in popularity across scientific fields.
Mindmap
Keywords
๐กBayesian Inference
๐กposterior beliefs
๐กlikelihood
๐กprior probability
๐กcontinuous variable
๐กposterior distribution
๐กA/B testing
๐กBayes Factor
๐กNull Hypothesis Significance Testing
๐กposterior odds
Highlights
Bayesian methods have helped companies make marketing decisions and researchers quantify results in scientific studies
Bayes' Theorem can update beliefs involving continuous variables, not just discrete ones
The Bayesian calculation matches intuition - probabilities of tails close to 0 or 1 became less likely after seeing 1 out of 5 tails
Businesses like Bayesian inference because it allows incorporating previous knowledge and expert opinion into calculations
A beauty blogger uses Bayesian A/B testing to determine if including a picture of her poodle Ginger increases email click rate
The posterior distributions indicate the group with dog pictures likely has a higher click rate
Simulating samples from the posterior distributions quantifies how likely the picture group's click rate is higher
Bayesian analysis enabled incorporating prior knowledge about typical email click rate
A psychology study on Dissociative Identity Disorder used Bayes Factors to quantify evidence that patient groups behaved differently
The large Bayes Factor means updated beliefs will likely reflect the groups being distinct regardless of prior beliefs
This provides evidence DID patients may not just pretend to not remember things from other personalities
Bayesian methods are used in language translation, purchase recommendations, medicine personalization, and AI
Bayes helps Siri understand you likely said "Turn on the lights" not "Learn all the sites"
Scientists and statisticians were limited in using Bayes historically by lack of computing power
Researchers wanted to know if DID patients, people who didn't see materials, and pretenders had different test accuracy
Transcripts
Browse More Related Video
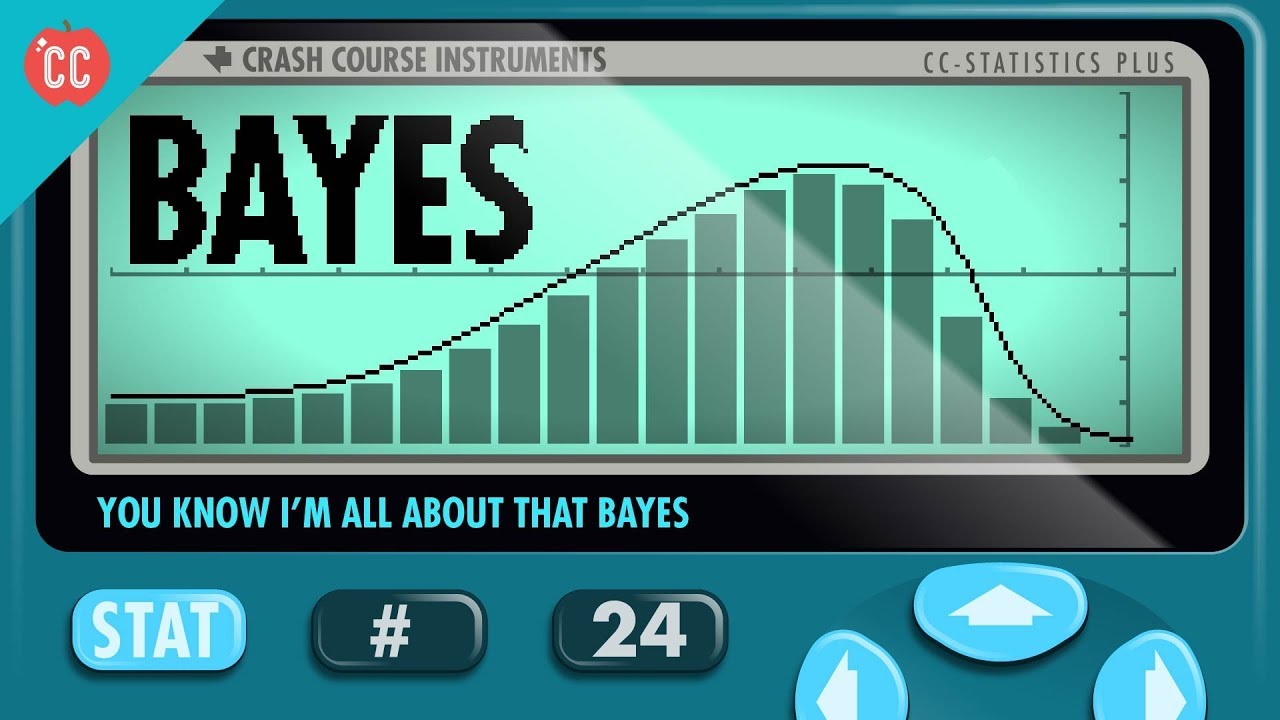
You Know I'm All About that Bayes: Crash Course Statistics #24
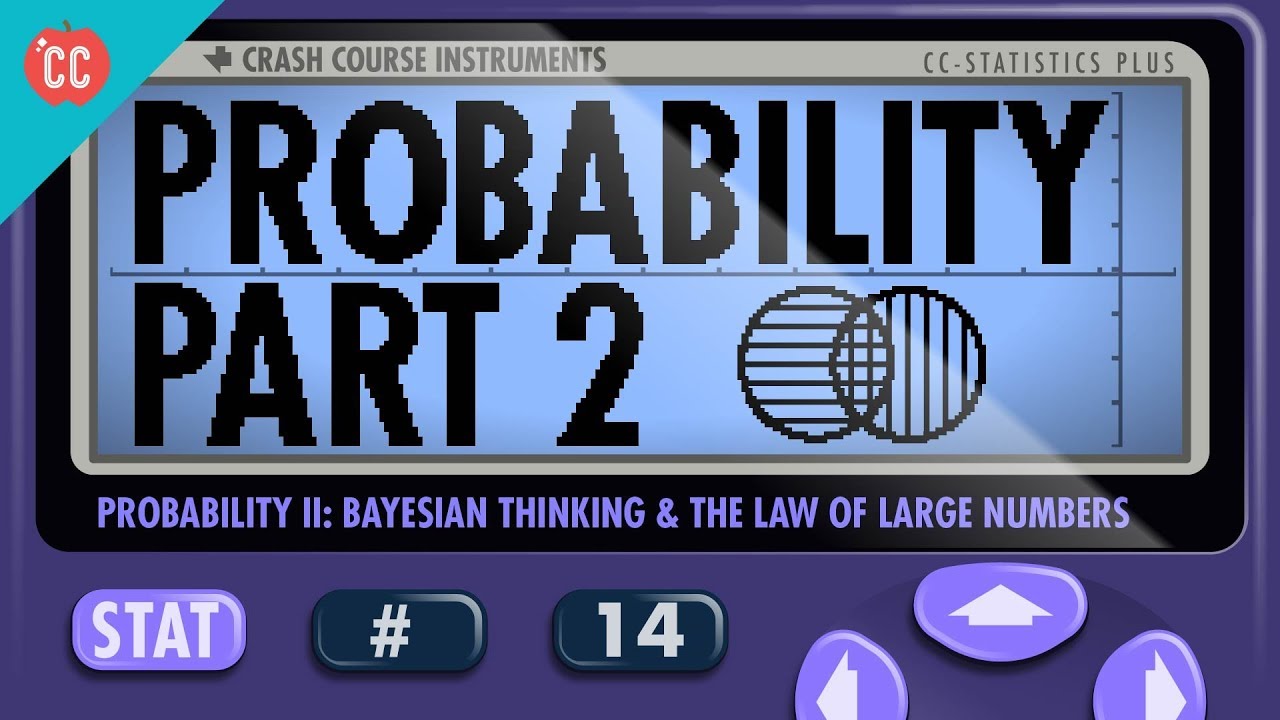
Probability Part 2: Updating Your Beliefs with Bayes: Crash Course Statistics #14
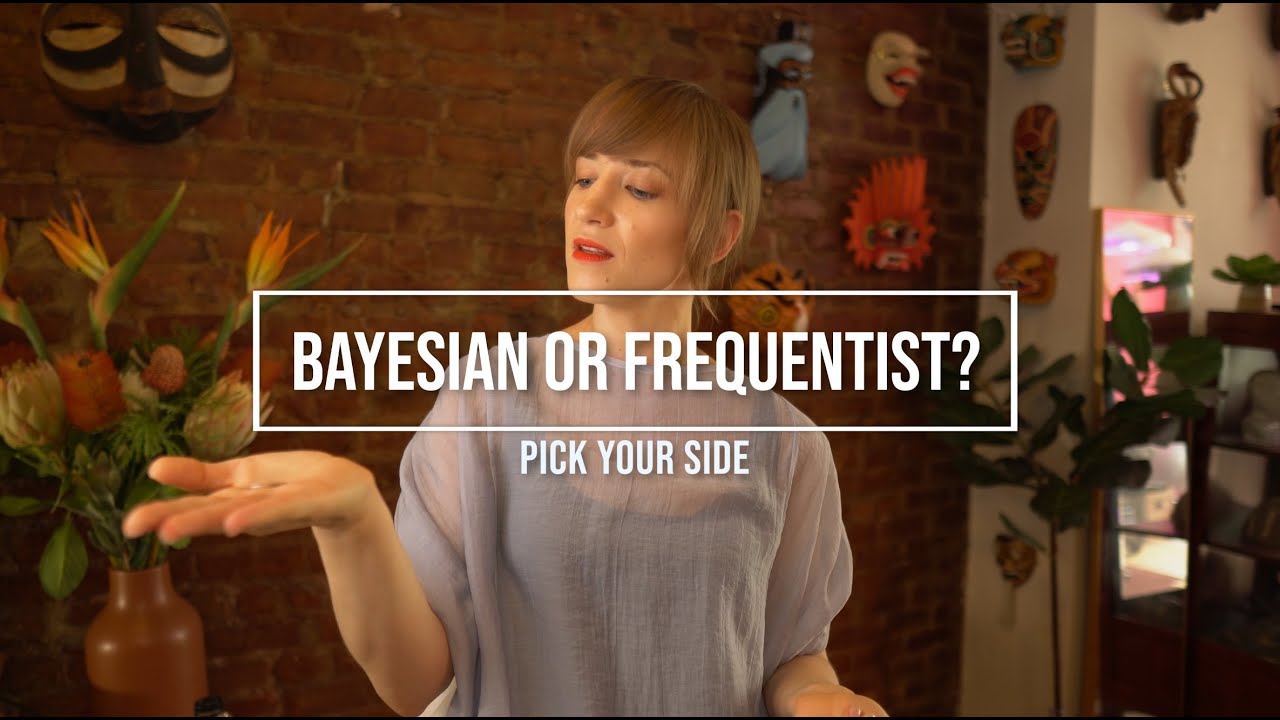
Are you Bayesian or Frequentist?
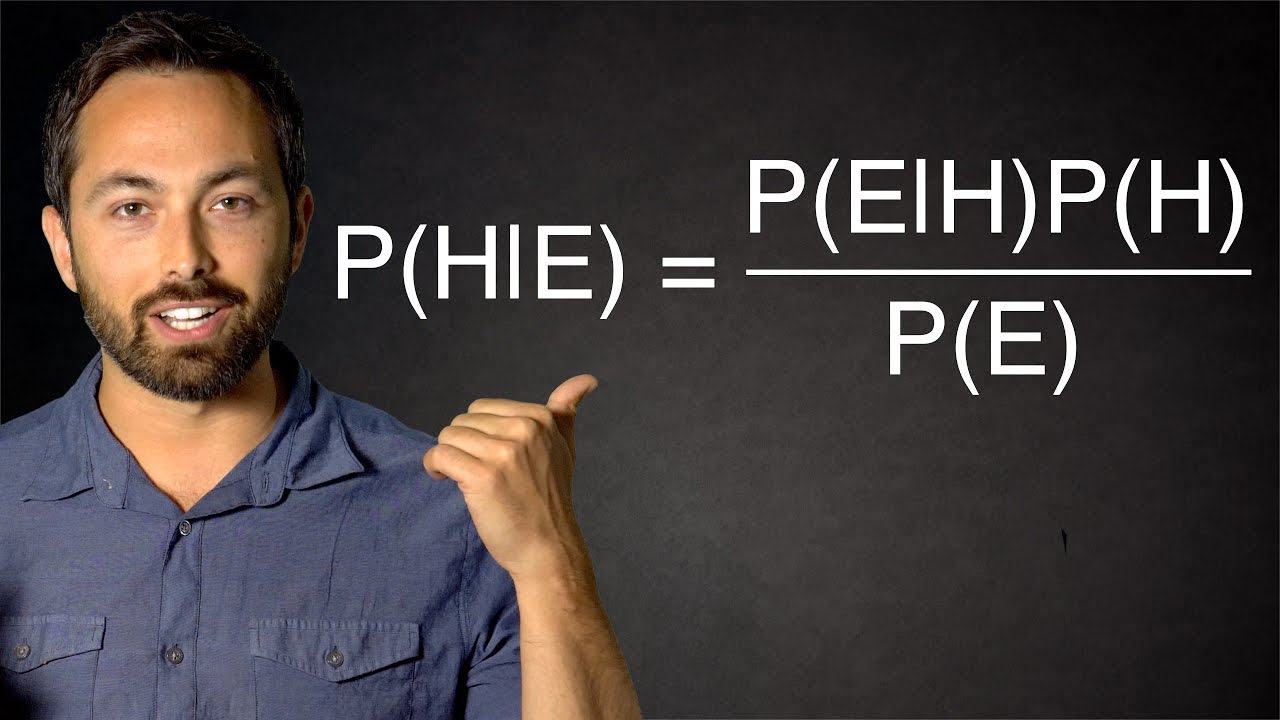
The Bayesian Trap
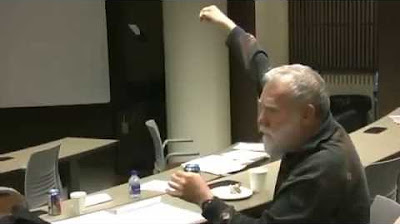
10. Saturday Panel: Conceptions of Empirical Success Conference

Bayes theorem, the geometry of changing beliefs
5.0 / 5 (0 votes)
Thanks for rating: