10. Saturday Panel: Conceptions of Empirical Success Conference
TLDRThe video script discusses the application of Bayesian theory and decision theory in scientific inference, highlighting their strengths and limitations. Various philosophers and scientists are quoted, addressing how these methods model beliefs, handle new hypotheses, and converge on agreement. The dialogue explores the flexibility of Bayesian methods, their idealized nature, and their relevance in explaining scientific phenomena. The conversation also touches on the significance of understanding science beyond just belief systems, emphasizing the need for epistemic skills and decision theory in philosophical and scientific inquiry.
Takeaways
- π The discussion revolves around the application of probability theory and decision theory to real-world scientific inference and the philosophical insights they provide.
- π οΈ Bayesianism is highlighted as a flexible framework that can model beliefs about any subject matter, including scientific ones, despite being highly idealized.
- π The transcript mentions the use of Bayesian methods to illuminate episodes in science, with a preference for robust reconstructions that consider a range of probabilities.
- π€ There's an acknowledgment of the limitations of Bayesian methods, such as the problem of priors and the quick divergent convergence to agreement, indicating that these methods should not be overstated in their epistemological significance.
- π The paradox of the Bayesian approach is noted, where it can rationalize too much, including the gambler's fallacy through counterinductive priors.
- π The richness of Bayesian theory is praised for its connections to decision theory, confirmation theory, and causal modeling, and its ability to illuminate features of confirmation in science.
- π§ The transcript suggests a division of labor between Bayesianism and other methods in traditional epistemology, with each taking up different aspects of scientific understanding.
- π§© The importance of Bayesianism in current scientific practice is emphasized, with its methods used widely across various sciences.
- π The role of philosophy in developing informal tools for understanding concepts like causation and inference is discussed, noting the impact on statistics and the importance of these concepts in modern science.
- π The need for recognizing limitations in inductive methods and the value of philosophers in critically examining these methods are underscored, especially in the context of scientific inference.
Q & A
What is the significance of Bayesian methods in illuminating episodes in science?
-Bayesian methods have been successful in providing robust reconstructions of scientific reasoning by examining the range of probabilities that still yield the same results, offering insights into how surprising evidence can confirm theories and the value of diverse evidence.
How does Bayesianism handle the problem of old evidence?
-Bayesianism can struggle with the problem of old evidence, where the impact of new data might seem to diminish the importance of previously accepted evidence. However, it can accommodate this by updating beliefs in light of new information, reinforcing the idea that neither piece of evidence is merely an artifact.
What is the role of decision theory in scientific inference?
-Decision theory plays a crucial role in scientific inference by helping to determine the importance of pursuing certain lines of investigation and assessing the expected utility of changes in belief as a result of proposed investigations.
How does the concept of 'lexicographic probabilities' relate to Bayesianism?
-Lexicographic probabilities extend Bayesianism by allowing for the handling of probability zero events, which can be useful in modeling scenarios where agents are committed to certain equilibria or assumptions that may be violated by alternatives.
What is the issue with the introduction of new hypotheses in Bayesian models?
-Bayesian models may not have a clear mechanism for introducing completely new hypotheses that were not previously considered within the framework, which can be a challenge when dealing with scientific progress that involves the formulation of novel concepts.
What is the problem of new evidence in Bayesian theory?
-The problem of new evidence refers to the challenge Bayesians face when new data or hypotheses arise that were not anticipated in their priors, requiring a reassessment of the prior probabilities and potentially leading to significant shifts in belief.
How does Bayesianism address the issue of empirical success in science?
-Bayesianism can address empirical success by providing a framework for understanding how agreement between diverse measurements reinforces beliefs in a theory, and how systematic deviations from predictions can indicate areas for further investigation.
What is the role of cognitive utilities in decision theory?
-Cognitive utilities in decision theory involve attaching utilities to belief states, which can help determine the importance of pursuing certain lines of inquiry or the value of accepting a proposition based on its truth or falsity.
How does Bayesianism deal with the problem of convergence to agreement?
-Bayesian convergence theorems suggest that with sufficiently rich data, discrepant agents will come to agreement. However, critics point out that for any dataset, there can be agents so discrepant that they will not agree, indicating a need for caution in overstating the epistemological significance of these results.
What is the philosophical absence in models of belief and inquiry?
-There is a lack of formal models that explain how questions arise, become more or less important, and spawn new questions within the context of belief and inquiry, particularly regarding the role of ignorance and the assessment of its importance.
How does the historical approach to scientific knowledge differ from a Bayesian perspective?
-A historical approach focuses on describing what actually happened in the development of scientific knowledge, whereas a Bayesian perspective may offer a rational reconstruction of events based on Bayesian principles, potentially leading to different interpretations of the same events.
Outlines
π The Integration of Bayesianism and Scientific Inference
The speaker discusses the applicability of probability theory and decision theory to real-world scientific inference. They mention the flexibility of Bayesianism to model beliefs on various subjects, including scientific ones. The idealized nature of Bayesian methods is compared to the idealizations in physics, such as frictionless planes. Success stories of Bayesian methods in science are highlighted, including the use of Bayesian reconstructions to analyze a range of probabilities and their outcomes. The speaker also addresses some criticisms of Bayesian methods, such as the problem of priors and the issue of divergent convergence. They conclude by appreciating Bayesianism's richness and its connections to various fields, suggesting it can complement traditional epistemology.
π The Role of Bayesian Methods in Philosophy and Science
This paragraph delves into the value of Bayesian methods in philosophy and their impact on scientific thought. The speaker reflects on the importance of engaging with probability and decision-making in philosophy, citing the significant role philosophers have played in developing concepts of causation and inference. They highlight the interdisciplinary relevance of these concepts in statistics and the acknowledgment of statisticians like Donald Rubin for their work in causal reasoning. The speaker also emphasizes the importance of understanding the limitations of inductive methods and the role of philosophers in exploring these limitations.
π± The Bayesian Approach to Causal Knowledge and Scientific Method
The speaker explores the Bayesian perspective on causal knowledge and the scientific method, emphasizing the importance of robust Bayesian treatments. They discuss the significance of understanding why control experiments provide causal knowledge through Bayesian priors over causations. The speaker also touches on the importance of agreement on certain features of foreign accreditation and the role of Bayesian thinking in understanding the success of control experiments and causal models. They conclude by expressing a desire for Bayesian models to be extended to capture the richness of scientific methodology.
π The Relevance of Bayesian Methods in Modern Science
The paragraph examines the prevalence and relevance of Bayesian methods in contemporary science. The speaker acknowledges the widespread use of Bayesian methods across various scientific disciplines and discusses the role of decision theory in scientific practice. They highlight the use of models in evolutionary processes and the verification of predictions made by these models. The speaker also discusses the importance of Bayesian considerations in evaluating the significance of diverse phenomena and the role of Bayesian models in understanding the agreement from independent measurements.
π§ The Bayesian Model and Newton's Scientific Method
The speaker discusses the application of the Bayesian model to Newton's scientific method, emphasizing the need for a Bayesian model to accommodate the concept of acceptance and division. They highlight Newton's moon test as an example of empirical success and discuss the importance of cruder moon test estimates providing resistance to large changes. The speaker also touches on the limitations of Bayesian models in capturing the full richness of Newton's methodology and the potential for extending these models to better reflect scientific practice.
π€ The Philosophical Absence in Understanding Scientific Inquiry
This paragraph focuses on the philosophical gap in understanding the nature of scientific inquiry, particularly the process of question formation and the varying importance of questions. The speaker notes the lack of formal models addressing how questions arise and gain significance. They call for a model that includes the element of ignorance and the assessment of its importance. The speaker also discusses the role of decision theory in scientific investigations and the importance of cognitive utilities in determining the significance of questions.
π The Challenge of New Hypotheses in Bayesian Epistemology
The speaker addresses the challenge of introducing new hypotheses within the Bayesian framework, particularly when these hypotheses were not anticipated by prior beliefs. They discuss the epistemic effects of new possibilities and the difficulty Bayesianism faces in dealing with such situations. The speaker also touches on the problem of new hypothesis and the need for Bayesian theory to accommodate the introduction of novel concepts and hypotheses.
π― The Bayesian Arsenal and the Sampling of New Species
The paragraph discusses a small aspect of the Bayesian arsenal that relates to the introduction of new concepts or 'species'. The speaker refers to the sampling problem noted by Bayes and the subsequent development of the concept by later philosophers and statisticians. They highlight the ability of lexicographic probabilities to handle many probability zero events and the use of these probabilities in game theory and economics. The speaker also notes the criticism of Bayesians for their use of different frameworks when needed.
π The Historical Approach to Scientific Understanding
The speaker discusses their approach to understanding science by focusing on historical descriptions rather than committing to a specific philosophical theory. They emphasize the importance of describing what happened historically to avoid distortion and the role of judgment in assessing how scientists reacted to evidence and new questions. The speaker also touches on the distinction between scientific facts and conjecture, as exemplified by Newton's work.
π οΈ The Skill Element in Scientific Understanding
In this paragraph, the speaker introduces the concept of understanding in science as involving a skill element, beyond just having the right beliefs or theories. They suggest that understanding phenomena involves deploying theory with skill, which is an epistemic skill that may be subject-specific. The speaker invites further discussion on this aspect of understanding in the context of scientific inquiry.
Mindmap
Keywords
π‘Probability Theory
π‘Decision Theory
π‘Bayesian Methods
π‘Scientific Inference
π‘Epistemology
π‘Convergence Theorems
π‘Causal Inference
π‘Lexicographic Probabilities
π‘Hypothetical Deductive Methodology
π‘Empirical Success
π‘Acceptance and Revision
Highlights
Discussion on the applicability of probability theory and decision theory to real-world scientific inference.
The importance of Bayesian methods in illuminating episodes in the history of science.
The robustness of Bayesian reconstructions in the face of varying probabilities.
Challenges with Bayesian methods, such as the problem of priors and the issue of quick divergent convergence.
The epistemological significance of convergence theorems and the caution against overstating their impact.
The richness of Bayesianism and its connections to various fields like decision theory, confirmation theory, and causal modeling.
The role of Bayesian methods in addressing skeptical challenges in science.
The value of pursuing Bayesian methods in philosophy for understanding scientific practices.
The significance of causal reasoning in statistics and its philosophical development.
The importance of understanding the limitations of inductive methods in scientific inference.
The contrarian view on the role of decision theory in understanding scientific evidence and the introduction of new hypotheses.
The discussion on the role of credence in torrent and the agreement on features of foreign accreditation.
The importance of control experiments in providing causal knowledge from a Bayesian perspective.
The potential of Bayesian models to capture the empirical success of scientific theories.
The challenge of introducing new concepts and hypotheses within the Bayesian framework.
The exploration of the role of understanding in science, beyond just having the right beliefs or theories.
The historical perspective on the distinction between established scientific facts and conjecture as demonstrated by Newton.
Transcripts
Browse More Related Video
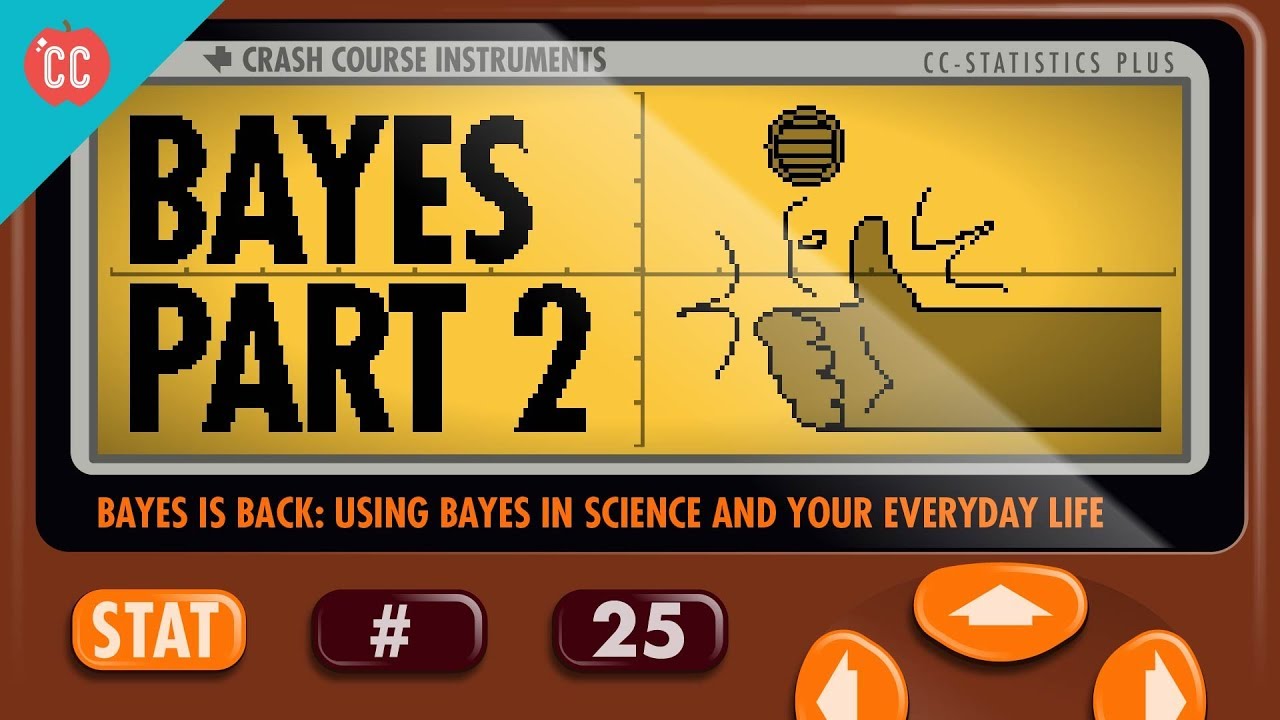
Bayes in Science and Everyday Life: Crash Course Statistics #25
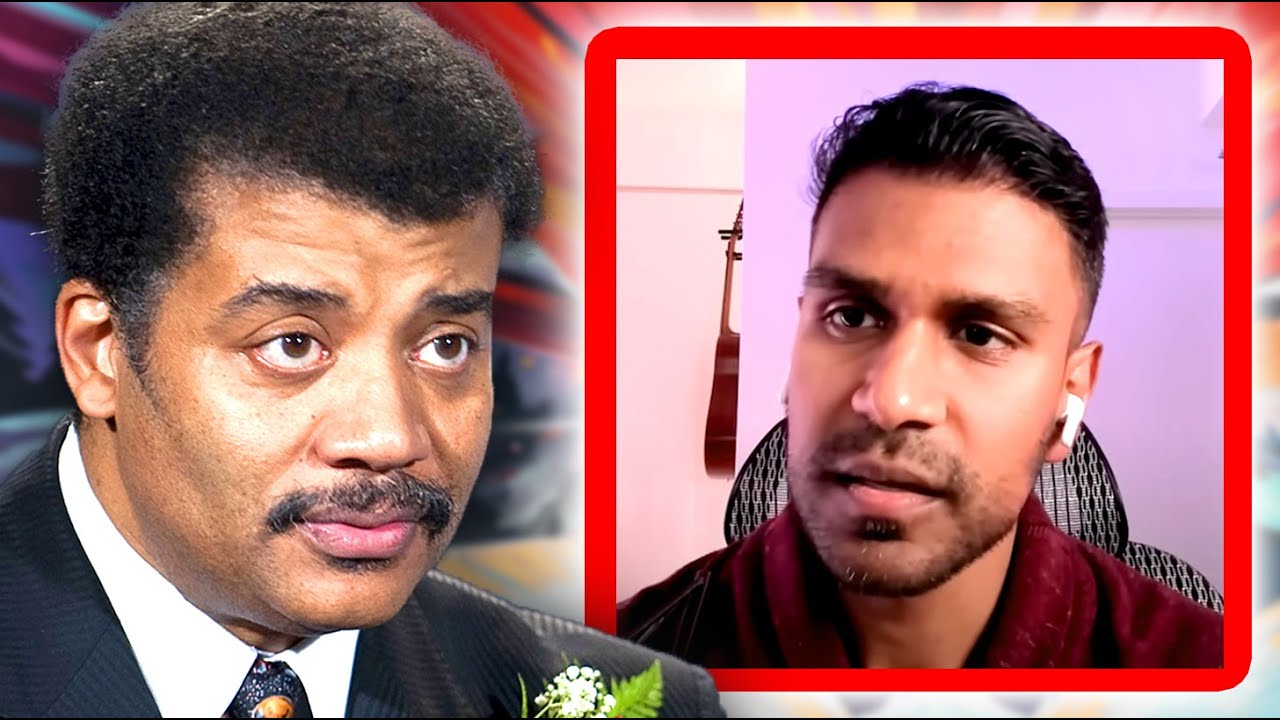
"Philosophers Are USELESS!" Neil & Curt Clash on Physics
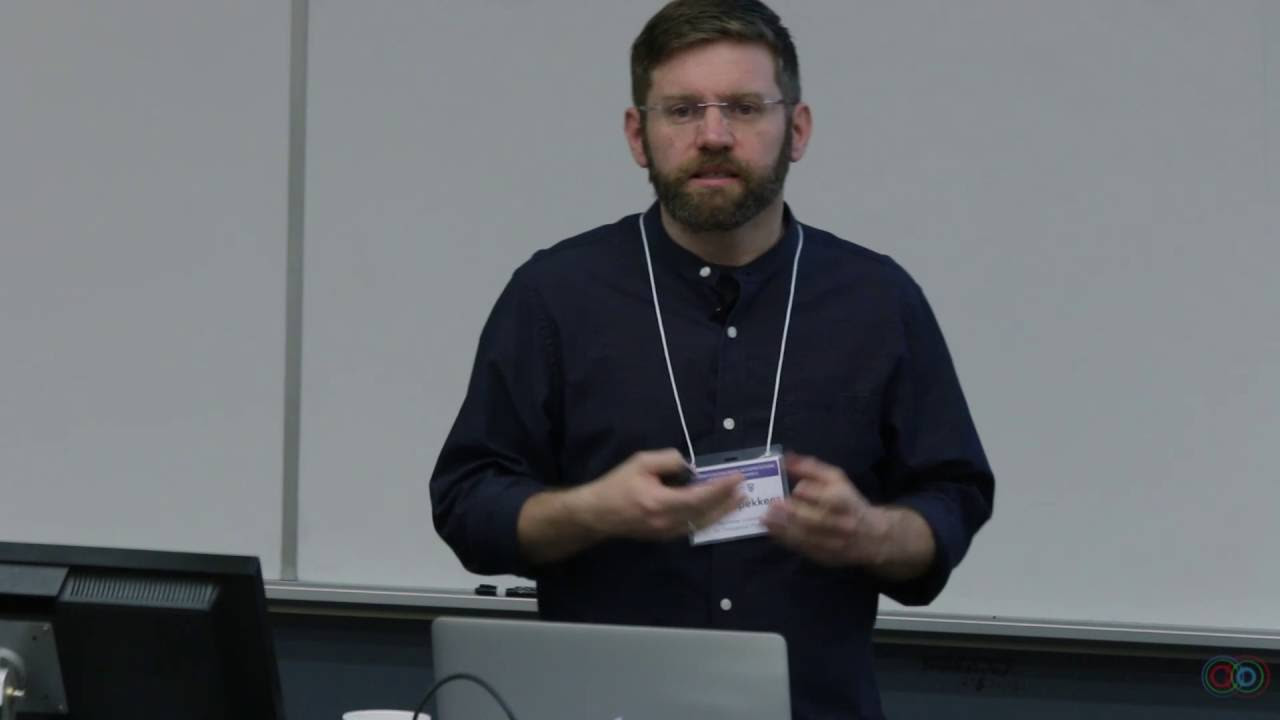
Robert Spekkens: Leibnizβs principle of the identity of indiscernible as...

Wayne Myrvold: Einstein and the Atom
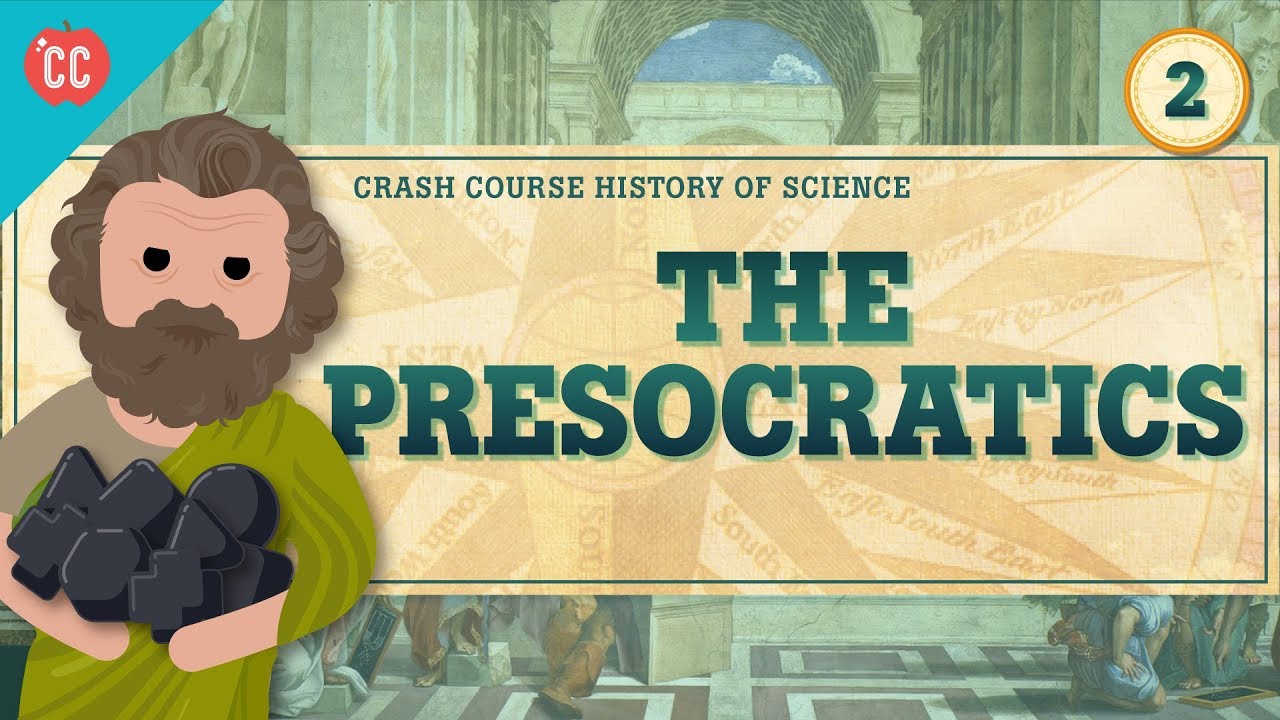
The Presocratics: Crash Course History of Science #2
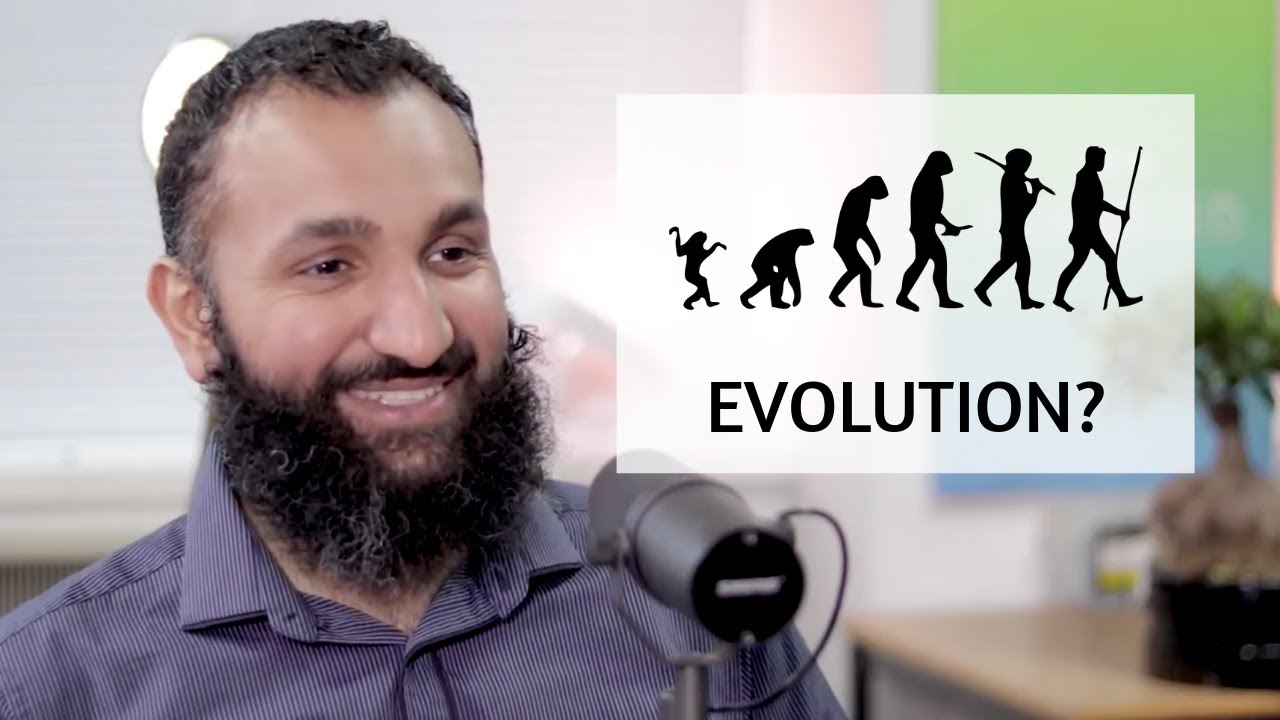
Should Muslims Believe In Evolution? | Subboor Ahmad
5.0 / 5 (0 votes)
Thanks for rating: