Probability: Bayes' Theorem
TLDRThis lesson explores Bayes Theorem through a medical testing scenario. It demonstrates how to calculate the probability of having a virus given a positive test result, using both intuitive methods and Bayes Theorem. The example highlights the surprising low accuracy of positive tests and the importance of understanding conditional probabilities.
Takeaways
- π§ Bayes Theorem is a fundamental concept in probability theory, used to find the probability of an event based on prior knowledge of conditions related to the event.
- π The script introduces an example involving a virus and a diagnostic test to illustrate the application of conditional probability and Bayes Theorem.
- π The prevalence of the virus in the population is one in every four hundred people, which is the base rate for calculating probabilities.
- π‘ The diagnostic test has an 85% true positive rate and a 5% false positive rate, which are crucial for understanding the test's accuracy.
- π The script uses a table to organize information about the virus infection status and test results, facilitating the calculation of conditional probabilities.
- π’ A hypothetical sample of ten thousand people is used to demonstrate the calculations, making it easier to understand the probabilities involved.
- π€ The probability of having the virus (event A) is calculated as 0.025, based on the infection rate of one in four hundred.
- π’ Of those infected, 85% test positive, leading to a calculation of how many in the sample would test positive given they have the virus.
- π‘ 5% of those not infected test positive, which is the false positive rate, and this is used to calculate the number of false positives in the sample.
- π The probability of a person having the virus given a positive test result is surprisingly low at approximately 4.09%, indicating the test's limitations.
- π Conversely, the probability of not having the virus given a negative test result is high at approximately 99.6%, showing the test's reliability in ruling out the virus.
- π The script concludes by verifying the calculated probabilities using Bayes Theorem, reinforcing the theorem's validity in such calculations.
Q & A
What is Bayes Theorem?
-Bayes Theorem, also known as Bayes rule or Bayes' law, is a mathematical formula that describes the probability of an event based on prior knowledge of conditions related to the event.
What is conditional probability?
-Conditional probability is the probability of an event occurring given that another event has already occurred.
What is the significance of false positives in the context of the virus test?
-False positives refer to the cases where a test incorrectly indicates that a person has the virus when they do not, which can lead to incorrect diagnoses and unnecessary treatment.
What is the probability of the virus infecting a person according to the script?
-The script states that the virus infects one in every four hundred people, which can be represented as a probability of 0.0025.
How accurate is the test used to detect the virus?
-The test is 85% accurate in detecting the virus when a person is actually infected and has a 5% false positive rate when the person does not have the virus.
What are the events A and B defined in the script?
-In the script, event A is defined as the person having the virus, and event B is defined as the person testing positive for the virus.
How many people out of ten thousand would be expected to have the virus based on the given probability?
-Using the probability of 0.0025, 25 out of ten thousand people would be expected to have the virus.
What is the probability of a person testing positive given they have the virus (P(B|A))?
-The probability of a person testing positive given they have the virus is 85%, or 0.85.
What is the probability of a person testing positive but not having the virus (false positives)?
-The probability of testing positive without having the virus is 5%, which is the false positive rate.
What is the calculated probability of a person actually having the virus given they tested positive (P(A|B))?
-The calculated probability of a person having the virus given they tested positive is approximately 4.09%.
How does the script suggest using intuition to find conditional probabilities as opposed to memorizing Bayes Theorem?
-The script suggests that it might often be easier to use intuition and a logical approach to determine conditional probabilities, rather than relying solely on memorizing the formula of Bayes Theorem.
Outlines
𧬠Introduction to Bayes Theorem
This paragraph introduces Bayes Theorem, a fundamental principle in probability theory. It sets the stage by discussing the concept of conditional probability using an example involving a virus test. The example involves a virus that infects one in every four hundred people and a test that is 85% accurate for detecting the virus and 5% prone to false positives. The goal is to calculate the probability of having the virus given a positive test result (P(A|B)) and the probability of not having the virus given a negative test result (P(not A|not B)). The paragraph also outlines the initial steps in setting up a table to organize the information and calculate these probabilities.
π Calculating Conditional Probabilities
This paragraph delves into the calculation of conditional probabilities using the example from the previous paragraph. It explains how to use the test accuracy rates and the prevalence of the virus to determine the likelihood of a person having the virus given a positive test result. The calculations involve determining the number of people who would test positive and actually have the virus, as well as those who test positive but do not have the virus (false positives). The paragraph also discusses the calculation of the probability of not having the virus given a negative test result. The results show that only about 4.09% of people who test positive actually have the virus, indicating the test is more reliable for ruling out the virus than confirming it. The paragraph concludes by verifying these findings using Bayes Theorem, reinforcing the importance of understanding both the intuitive and mathematical approaches to probability.
Mindmap
Keywords
π‘Bayes Theorem
π‘Conditional Probability
π‘False Positives
π‘Probability of A given B (P(A|B))
π‘Probability of not A given not B (P(not A|not B))
π‘Virus Infection Rate
π‘Test Sensitivity
π‘Test Specificity
π‘Sample Population
π‘Intuitive Calculation
π‘Bayes Theorem Application
Highlights
Introduction to Bayes Theorem and its application in conditional probability problems.
Example of using intuition to solve a conditional probability problem before applying Bayes Theorem.
Definition of the virus infection rate and test accuracy in terms of true positives and false positives.
Setting up the problem with events A (having the virus) and B (testing positive).
Organizing information in a 2x2 table to calculate conditional probabilities.
Calculating the expected number of people with the virus in a sample of 10,000.
Determining the number of true positives based on the infection rate and test accuracy.
Calculating the number of false positives among those who do not have the virus.
Finding the number of true negatives by subtracting false positives from the total without the virus.
Summing up the columns to verify the total number of people testing positive and negative.
Calculating the probability of having the virus given a positive test (P(A|B)).
Surprising result that only 4.09% of people who test positive actually have the virus.
Calculating the probability of not having the virus given a negative test (P(Β¬A|Β¬B)).
High accuracy of the test in determining who does not have the disease (99.6%).
Verification of the calculated probabilities using Bayes Theorem.
Discussion on the ease of using intuition vs. memorizing Bayes Theorem for solving problems.
Conclusion emphasizing the practical implications of the example and the value of the lesson.
Transcripts
Browse More Related Video
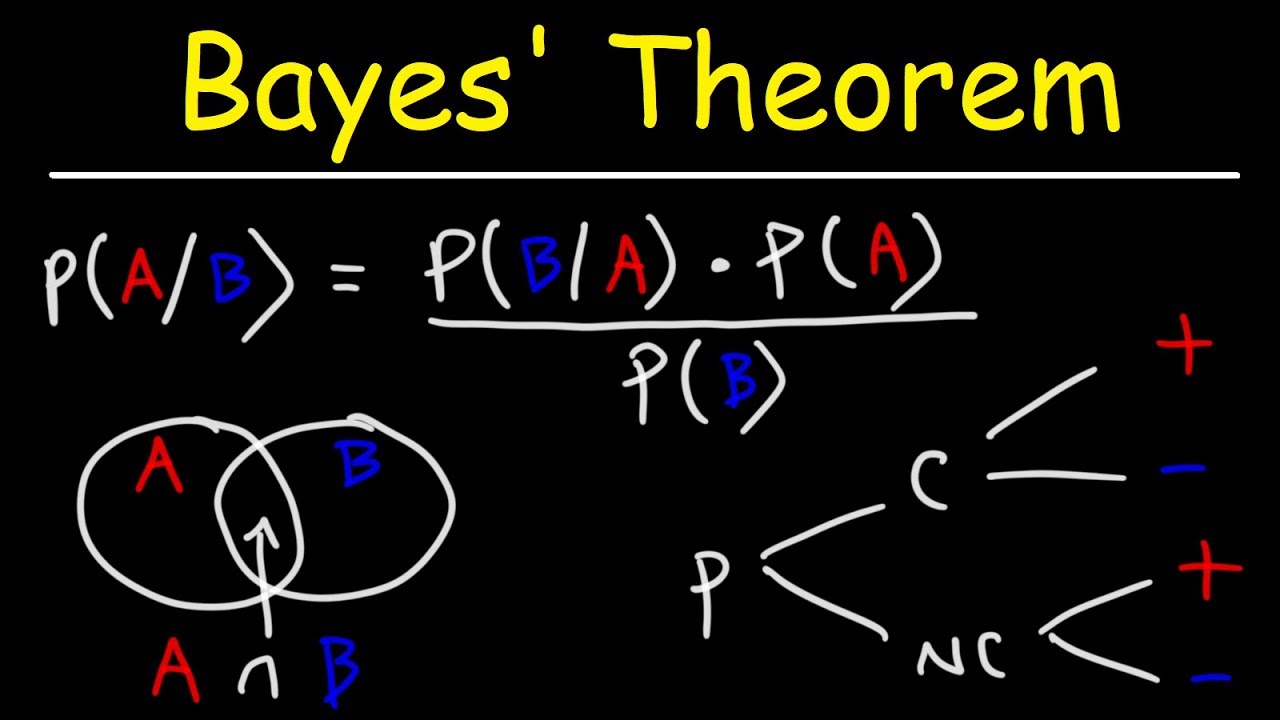
Bayes' Theorem of Probability With Tree Diagrams & Venn Diagrams
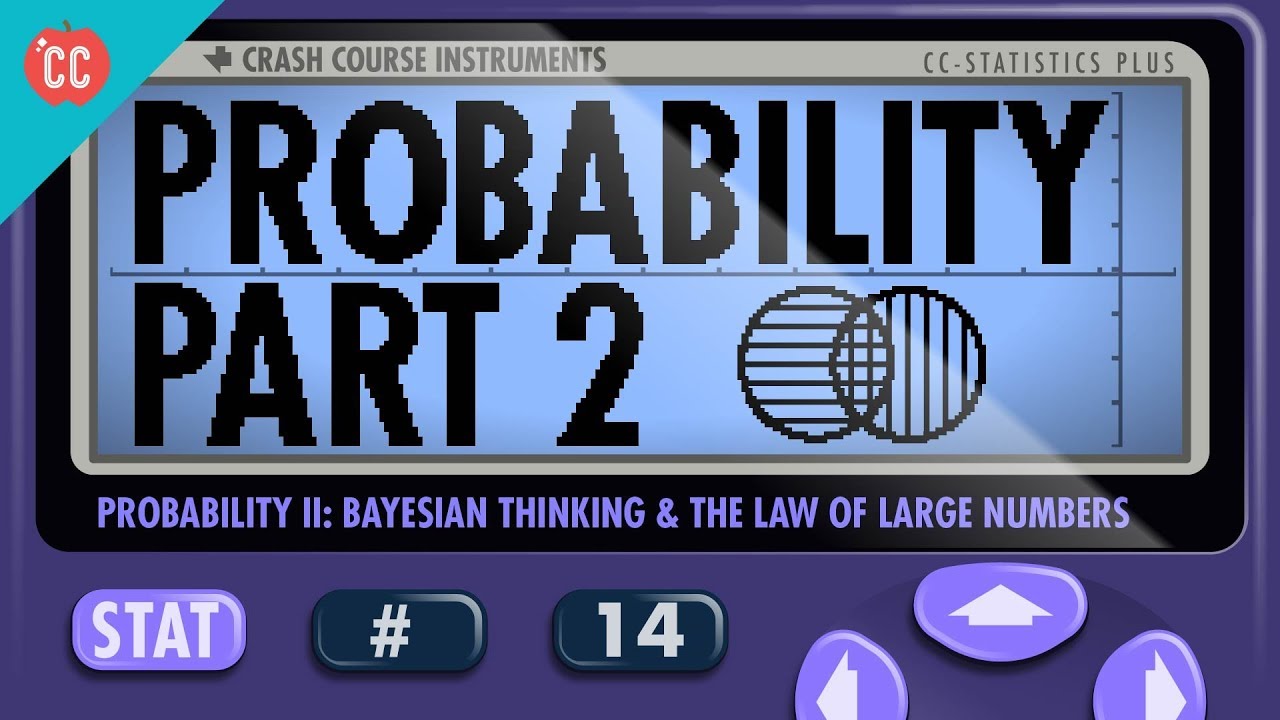
Probability Part 2: Updating Your Beliefs with Bayes: Crash Course Statistics #14
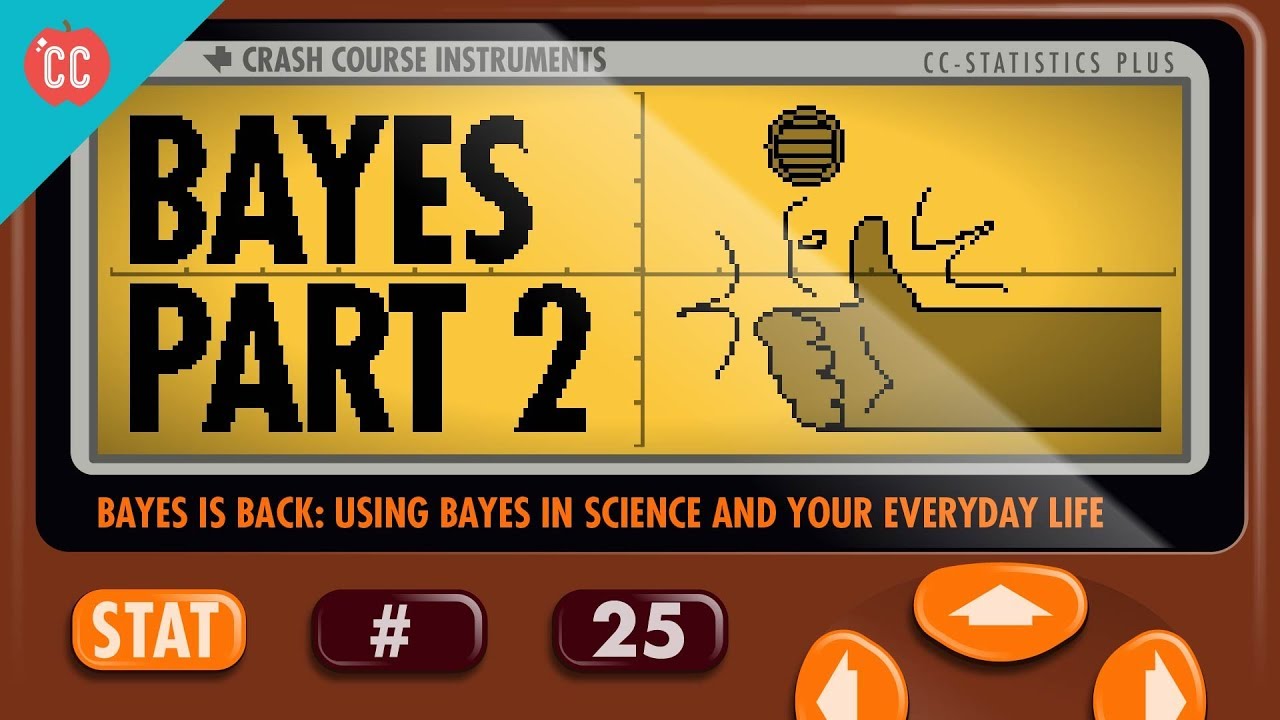
Bayes in Science and Everyday Life: Crash Course Statistics #25
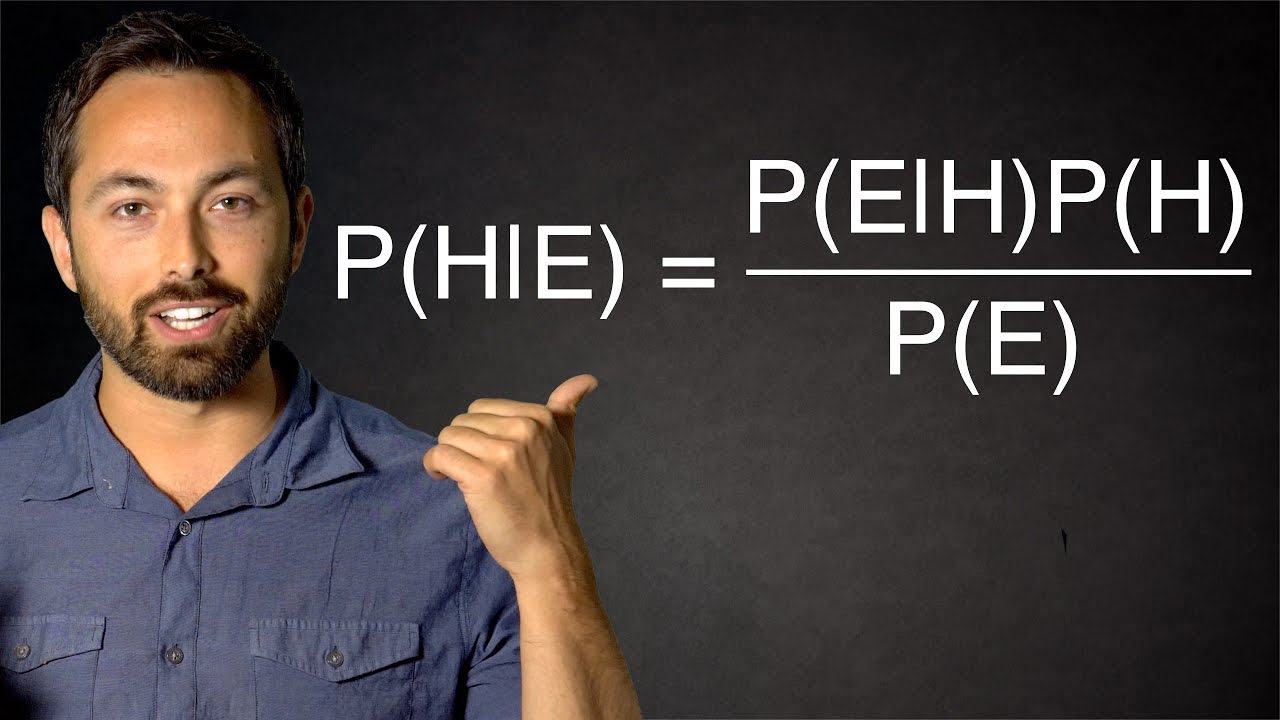
The Bayesian Trap
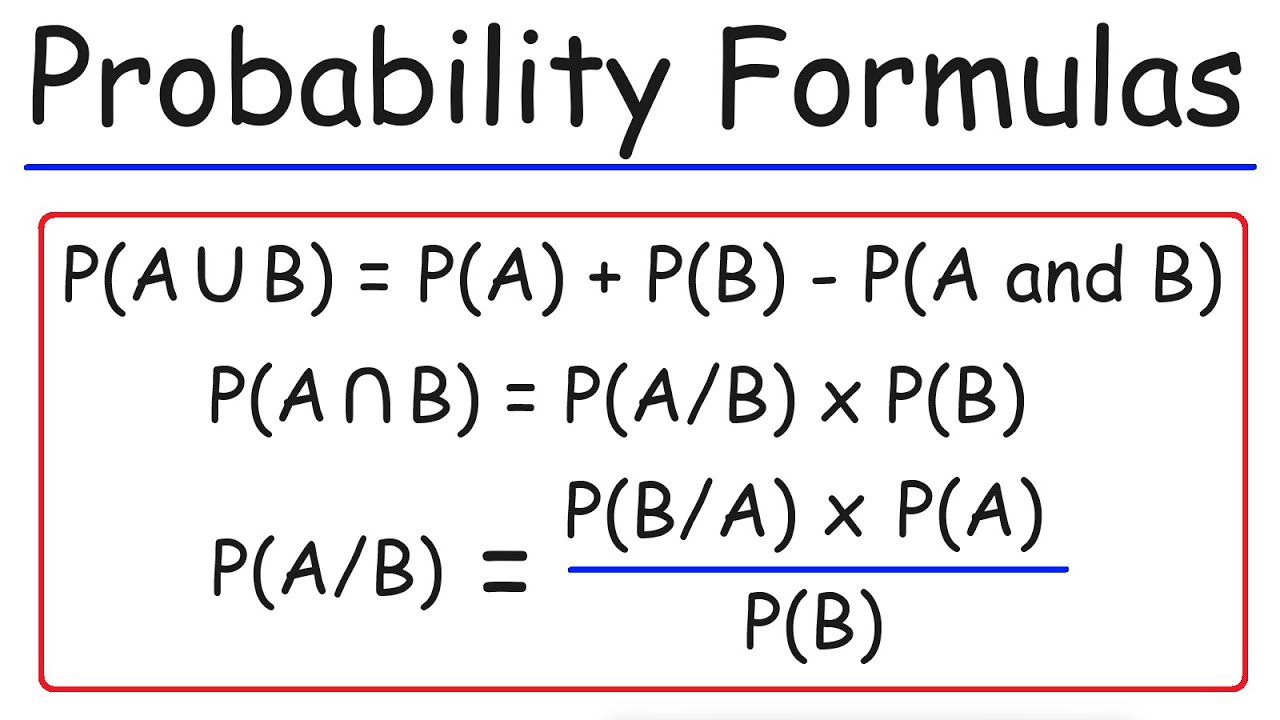
Probability Formulas, Symbols & Notations - Marginal, Joint, & Conditional Probabilities
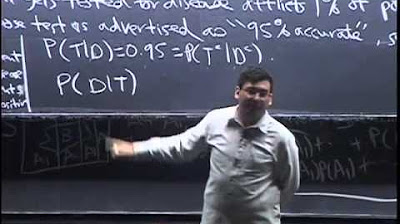
Lecture 5: Conditioning Continued, Law of Total Probability | Statistics 110
5.0 / 5 (0 votes)
Thanks for rating: