Eta Squared Effect Size for One-Way ANOVA (12-7)
TLDRThe video script explains the importance of reporting effect size alongside statistical significance in hypothesis testing, particularly for one-way ANOVA. It clarifies the distinction between statistical and practical significance, emphasizing that while statistical significance is influenced by sample size, practical significance, measured by effect size, indicates real-world impact. The script teaches how to calculate effect size measures like Eta squared, partial Eta squared, and Omega squared using the ANOVA summary table. It also demonstrates how to obtain these measures using SPSS, highlighting partial Eta squared as the most commonly reported measure due to its unbiased nature.
Takeaways
- π Reporting an effect size is essential alongside statistical tests to distinguish between statistical and practical significance.
- π Statistical significance indicates that observed differences are unlikely to occur by chance, but it can be influenced by sample size.
- π Practical significance measures the real-world impact of differences, independent of sample size.
- π’ There are two types of practical significance: strength of association and effect size.
- π Eta squared is a measure of strength of association in ANOVA, equivalent to R squared, explaining the variance in the dependent variable.
- βοΈ Partial Eta squared is an unbiased correction to Eta squared, providing a more accurate measure of the strength of association.
- π The formula for calculating partial Eta squared can be derived from the ANOVA summary table.
- π Omega squared is another effect size measure for ANOVA, which is also unbiased and always smaller than partial Eta squared.
- π§© Both partial Eta squared and Omega squared can be calculated using data from the ANOVA summary table.
- π» In SPSS, effect sizes for ANOVA can be computed using the 'General Linear Model > Univariate' procedure.
- π The video script uses the example of complaints about wicked witches from 'The Wizard of Oz' to illustrate the calculation of effect sizes.
Q & A
Why is it important to report an effect size when reporting a statistical test?
-Reporting an effect size is important because it helps distinguish between statistical significance and practical significance. While statistical significance indicates that observed differences are unlikely to occur if the null hypothesis is true, effect size measures the magnitude and practical importance of these differences, independent of sample size.
What is the difference between statistical significance and practical significance?
-Statistical significance is a function of sample size and indicates that observed differences are unlikely to be due to chance. Practical significance, on the other hand, refers to the real-world impact or importance of these differences, regardless of sample size.
What are the two types of practical significance mentioned in the script?
-The two types of practical significance mentioned are strength of association and effect size. Strength of association measures the percentage of variance in the dependent variable accounted for by the independent variable, while effect size provides a standardized measure of the difference between the sample and the null hypothesis.
What is Eta squared and how is it interpreted?
-Eta squared is a measure of strength of association in ANOVA, equivalent to R squared in regression analysis. It explains the percentage of variance in the dependent variable that is accounted for by the independent variable.
Why might Eta squared overestimate the true effect size?
-Eta squared may overestimate the true effect size because it does not account for the bias that can occur, especially in small sample sizes or when there are many levels in the independent variable.
What is Partial Eta squared and how does it differ from Eta squared?
-Partial Eta squared is an unbiased correction to Eta squared. It is interpreted in the same way as Eta squared, representing the percentage of variance in the dependent variable accounted for by the independent variable, but it adjusts for the bias, making it a more accurate measure of effect size.
How can Partial Eta squared be calculated manually?
-Partial Eta squared can be calculated manually using the information from the ANOVA summary table. The formula involves the sum of squares between and within, and the specific values can be plugged in to calculate the strength of association.
What is Omega squared and how does it relate to other measures of effect size?
-Omega squared is the standard effect size measurement for ANOVA. It is interpreted similarly to R squared, representing the percentage of variance explained, but it is an unbiased measure of effect size. It is always smaller than partial eta squared, which in turn is always smaller than eta squared.
How can effect size for ANOVA be computed using SPSS?
-In SPSS, effect size for ANOVA can be computed by using the 'Analyze > General Linear Model > Univariate' path. After setting up the model, one can request estimates of effect size under the 'Options' menu.
What is the difference between using the 'Explore Means' command and the 'General Linear Model' in SPSS for ANOVA?
-The 'Explore Means' command is simpler and easier for teaching purposes as it only contains information for ANOVA. However, it does not provide an option for partial Eta squared. The 'General Linear Model' in SPSS offers more options and includes the ability to calculate partial Eta squared, making it more suitable for detailed analysis.
Can the same procedure for calculating effect size be applied to any one-way ANOVA?
-Yes, the procedure for calculating effect size, including the formulas for eta squared, partial eta squared, and omega squared, can be applied to any one-way ANOVA, making it a versatile method for assessing the magnitude of differences in various studies.
Outlines
π Understanding Effect Size in One-Way ANOVA
This paragraph discusses the importance of reporting effect size alongside statistical significance in hypothesis testing, particularly in one-way ANOVA. It explains the distinction between statistical significance, which can be influenced by sample size, and practical significance, which measures the real-world impact of observed differences. The paragraph introduces two types of practical significance: strength of association and effect size. It defines Eta squared as a measure of strength of association, explaining its interpretation and how it can be biased. To address this, the paragraph introduces partial Eta squared as an unbiased correction to Eta squared, which can be calculated using the ANOVA summary table. The Wizard of Oz example is used to illustrate the calculation of partial Eta squared, resulting in 66.3% of variability explained by the region. The paragraph also mentions Omega squared as another effect size measure, which is unbiased and always smaller than Eta squared and partial Eta squared.
π Calculating and Interpreting Effect Sizes in SPSS
The second paragraph focuses on calculating effect sizes for ANOVA using SPSS. It explains that Omega squared, being an unbiased measure, is always smaller than partial eta squared, which in turn is smaller than eta squared. The paragraph provides a formula for calculating Omega squared, which is more complex than that for partial eta squared but uses data available from the ANOVA summary table. Using data from the Wizard of Oz example, the paragraph demonstrates how to compute Omega squared, resulting in 58.7% of variability explained by the region. The paragraph then outlines the steps to conduct an ANOVA analysis in SPSS, including navigating through the software's menus and selecting the appropriate options to obtain effect size estimates. It concludes by showing how to find the partial Eta squared value in SPSS output, which confirms the manual calculation and indicates the percentage of variability explained by the independent variable.
Mindmap
Keywords
π‘Effect Size
π‘Statistical Significance
π‘Practical Significance
π‘Strength of Association
π‘Eta Squared
π‘Partial Eta Squared
π‘Omega Squared
π‘ANOVA Summary Table
π‘SPSS
π‘GLM 1 Model
Highlights
Importance of reporting effect size along with statistical significance.
Difference between statistical and practical significance.
Statistical significance depends on sample size and may not reflect meaningful differences.
Practical significance measures real-world impact of differences.
Two types of practical significance: strength of association and effect size.
Eta squared as a measure of strength of association in ANOVA.
Eta squared is equivalent to R squared and explains variance in dependent variable.
Partial Eta squared as an unbiased correction to Eta squared.
Formula for calculating partial Eta squared from ANOVA summary table.
Example calculation of partial Eta squared using data from The Wizard of Oz.
Introduction of Omega squared as a standard unbiased effect size measurement.
Omega squared is always smaller than partial eta squared and eta squared.
Formula for calculating Omega squared using data from ANOVA summary table.
SPSS method for computing effect size in ANOVA.
Using SPSS to calculate partial Eta squared and Omega squared.
Detailed SPSS output explanation including partial Eta squared value.
Transcripts
Browse More Related Video

Significance vs. Effect Size for One Way ANOVA using SPSS

P value versus effect size
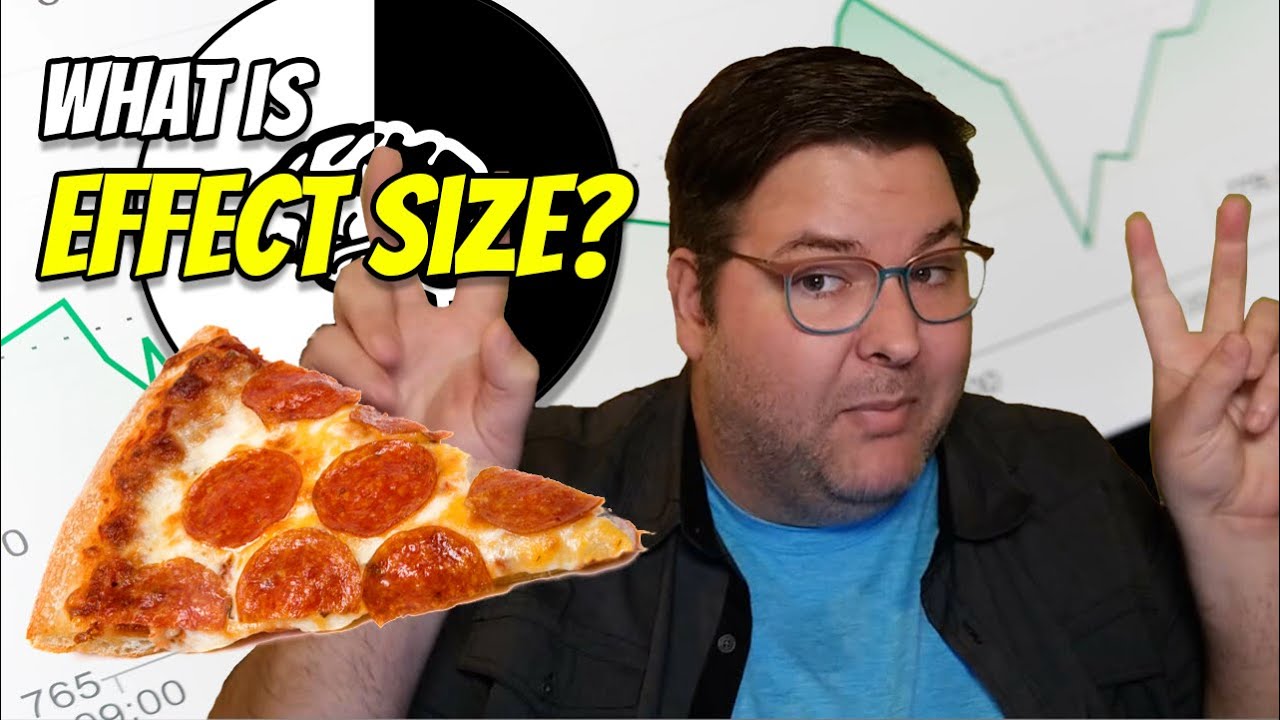
What is Effect Size? Statistical significance vs. Practical significance
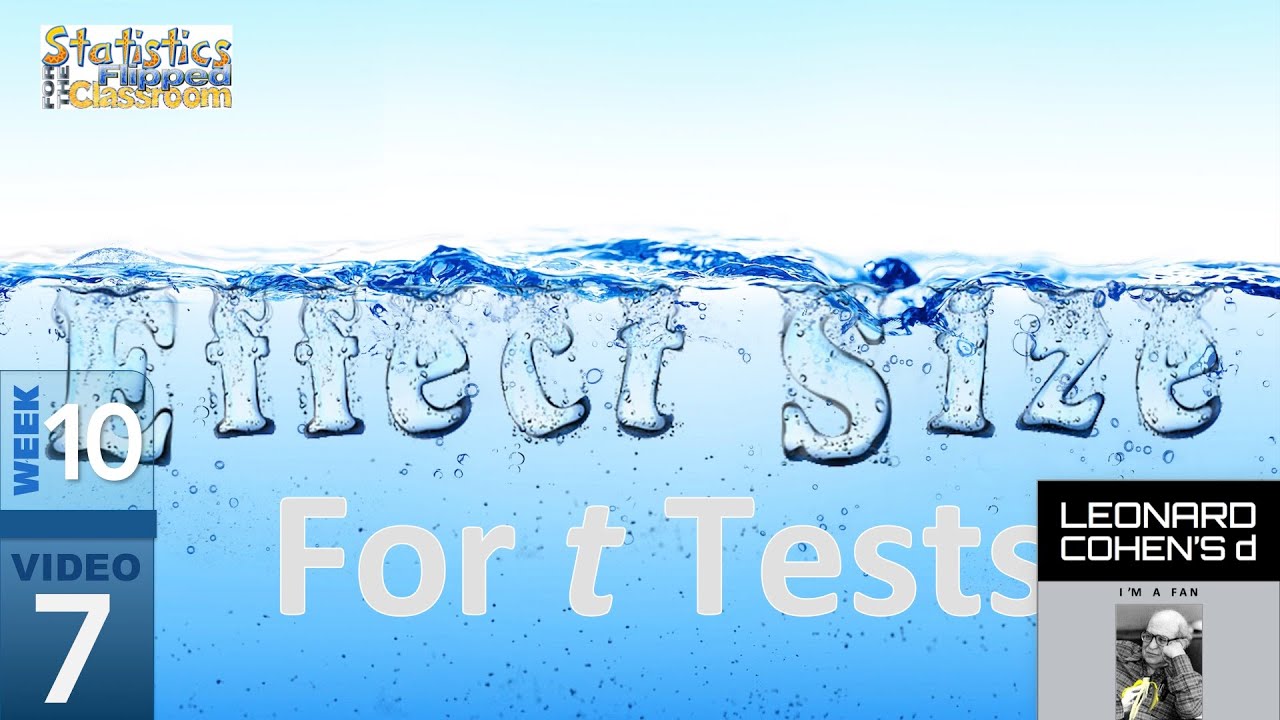
Cohenβs d Effect Size for t Tests (10-7)
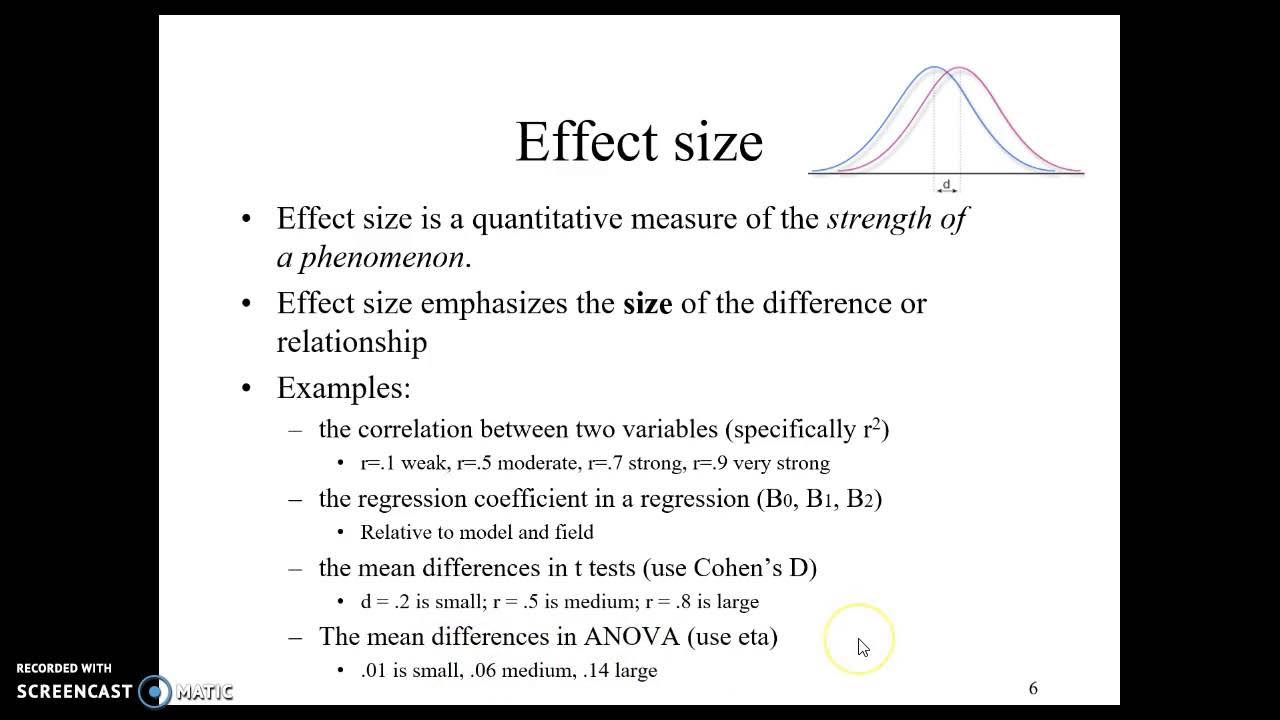
Power & Effect Size
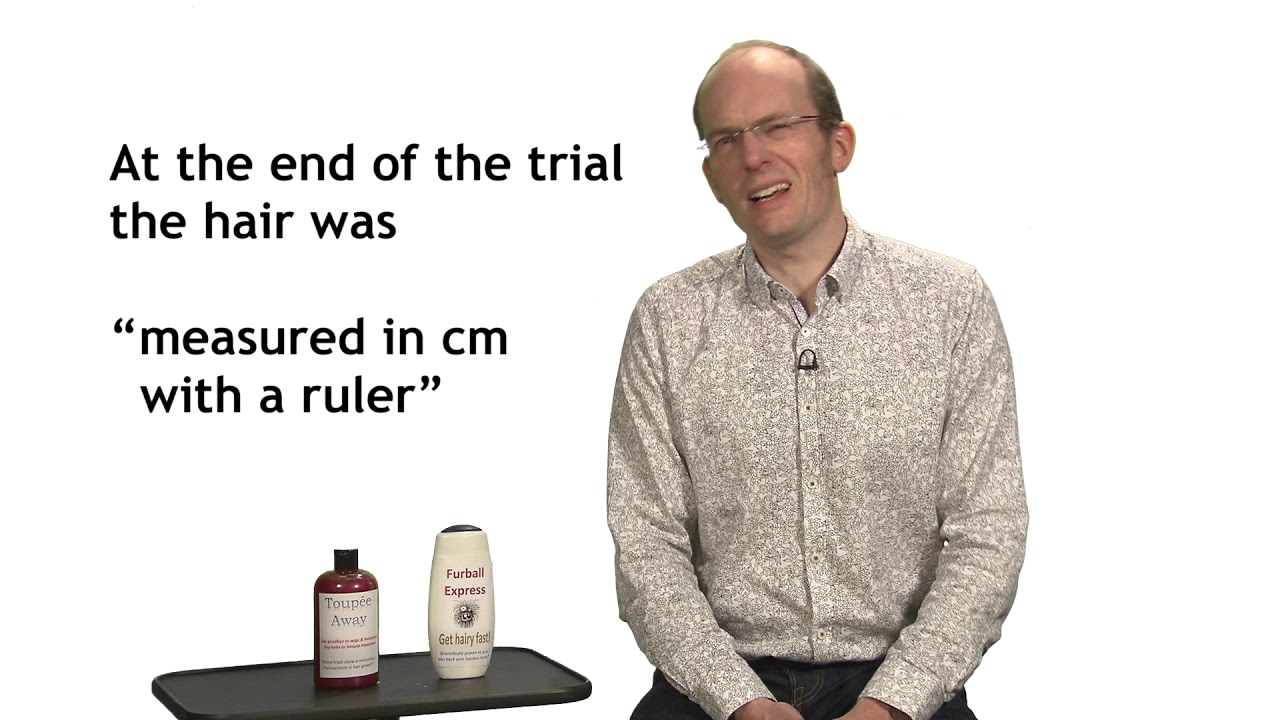
What do my results mean Effect size is not the same as statistical significance. With Tom Reader.
5.0 / 5 (0 votes)
Thanks for rating: