[Proof] MSE = Variance + Bias²
TLDRThe video script presents a detailed proof of the relationship between the mean square error (MSE), bias, and variance of an estimator. It explains that the MSE of an estimator, denoted as theta hat, is equal to the sum of the bias squared and the variance. The proof uses expectation equations for MSE, bias, and variance, and demonstrates how these components combine to form the MSE. The explanation is methodical, showing the step-by-step breakdown of the expected value expressions, leading to the conclusion that the variance of theta hat plus the bias squared equals the MSE, thus validating the initial claim.
Takeaways
- 📈 The script discusses a proof related to statistics and probability.
- 🔢 The main focus is on the relationship between mean square error (MSE), bias, and variance of an estimator.
- 🎩 The estimator used in the example is denoted as theta hat (θ̂).
- 📌 The proof aims to show that MSE of an estimator equals bias squared plus variance.
- 🧠 The proof uses expectation definitions and equations for MSE, bias, and variance.
- 🔄 The expected value of (θ̂ - θ)² is used to define MSE.
- 📂 The variance of θ̂ is defined as E[(θ̂)²] - E[θ̂]².
- 🏹 The bias of θ̂ is E[θ̂] - θ, with θ being a constant parameter.
- 📊 The script breaks down the expected value of (θ̂ - θ)² to derive the components of MSE.
- 🧩 By expanding and simplifying the expressions, the proof demonstrates that bias squared plus variance equals MSE.
- 🎓 The proof concludes that the variance of θ̂ plus the bias squared of θ̂ equals the MSE of θ̂, confirming the initial hypothesis.
Q & A
What is the main topic of the video?
-The main topic of the video is the proof of the relationship between the mean square error of an estimator, its bias, and its variance.
What is the estimator referred to as in the script?
-The estimator is referred to as theta hat (θ̂) in the script.
How is the mean square error (MSE) of an estimator defined?
-The mean square error (MSE) of an estimator is defined as the expected value of the squared difference between the estimator and the true parameter value.
What is the formula for bias in the context of the script?
-In the context of the script, bias is defined as the expected value of the estimator (theta hat) minus the true parameter value (theta).
How is variance of an estimator defined?
-The variance of an estimator is defined as the expected value of the estimator squared minus the square of the expected value of the estimator.
What is the relationship between MSE, bias, and variance that the video aims to prove?
-The video aims to prove that the mean square error (MSE) of an estimator is equal to the square of the bias plus the variance.
How does the script break down the expected value expression for MSE?
-The script breaks down the expected value expression for MSE by expanding the binomial expression (theta hat - theta) squared and distributing the expected value to each term.
What is the significance of the cancellation of terms in the final expression?
-The cancellation of terms in the final expression demonstrates that the sum of the variance of theta hat and the square of the bias equals the mean square error, thus proving the relationship.
How does the script handle the constant theta in its equations?
-In the script, theta is considered a parameter and is treated as a constant rather than a random variable, while theta hat is treated as a random variable.
What is the final result of the proof presented in the video?
-The final result of the proof is that the variance of theta hat plus the square of the bias of theta hat is equal to the mean square error of theta hat, confirming the relationship between these three quantities.
What is the role of expectation in the proof?
-Expectation plays a crucial role in the proof as it is used to define MSE, bias, and variance, and to manipulate and simplify the expressions to demonstrate their interrelationships.
Outlines
📊 Proof of Mean Square Error Relationship
This paragraph introduces a statistics and probability proof focused on demonstrating the relationship between the mean square error (MSE) of an estimator and its bias and variance. The proof aims to show that the MSE of an estimator (denoted as theta hat) is equal to the sum of the bias squared and the variance. The explanation begins with defining MSE, bias, and variance using expectation equations. It then proceeds to decompose the expected value definition of MSE and demonstrates its equivalence to the sum of variance and bias squared, thereby proving the theorem.
Mindmap
Keywords
💡Statistics
💡Probability
💡Mean Square Error (MSE)
💡Estimator
💡Bias
💡Variance
💡Expectation
💡Random Variable
💡Proof
💡Decomposition
💡Parameter
Highlights
The video aims to prove a relationship between mean square error, bias, and variance in statistics and probability. (Start time: 0s)
The mean square error (MSE) of an estimator is defined and will be proven to be equal to the sum of its bias squared and variance. (Start time: 2s)
The estimator used in the proof is denoted as theta hat (θ̂), and the parameter as theta (θ). (Start time: 4s)
The expectation definitions for MSE, bias, and variance are introduced and used in the proof. (Start time: 6s)
MSE is defined as the expected value of (θ̂ - θ)², which is the focus of the proof. (Start time: 8s)
Bias is defined as E[θ̂] - θ, representing the difference between the expected value of the estimator and the true parameter value. (Start time: 10s)
Variance is defined as E[(θ̂)²] - (E[θ̂])², measuring the spread of the estimator around its expected value. (Start time: 12s)
The proof begins by expanding the expectation of (θ̂ - θ)² using distribution properties. (Start time: 14s)
The expanded form of the MSE expectation is E[(θ̂)²] - 2θE[θ̂] + θ². (Start time: 16s)
The proof then shows that (E[(θ̂)²] - 2θE[θ̂] + θ²) can be rewritten as (E[(θ̂)²] - (E[θ̂])²) - 2θ(E[θ̂] - θ). (Start time: 18s)
The expression (E[(θ̂)²] - (E[θ̂])²) represents the variance of θ̂, and -2θ(E[θ̂] - θ) represents the bias squared. (Start time: 20s)
By combining the variance and bias squared terms, the proof shows that they equal the MSE. (Start time: 22s)
The proof concludes by showing that the variance of θ̂ plus the bias squared of θ̂ equals the MSE, confirming the initial hypothesis. (Start time: 24s)
The proof is a demonstration of the fundamental relationship between MSE, bias, and variance in statistical estimation. (Start time: 26s)
The video provides a clear and concise explanation of the statistical concepts and their interrelationships. (Start time: 28s)
The proof methodically breaks down the components of MSE and relates them to bias and variance, enhancing understanding of these concepts. (Start time: 30s)
The video is an educational resource for those interested in statistics, probability, and the theory behind estimation methods. (Start time: 32s)
The proof is relevant for anyone studying or working in fields that require statistical analysis and estimation. (Start time: 34s)
The video's approach to explaining the proof is accessible, making complex statistical concepts more understandable. (Start time: 36s)
The proof serves as a foundation for further studies in advanced statistical estimation techniques. (Start time: 38s)
The video's content is a valuable addition to the educational material available on statistics and probability. (Start time: 40s)
Transcripts
Browse More Related Video
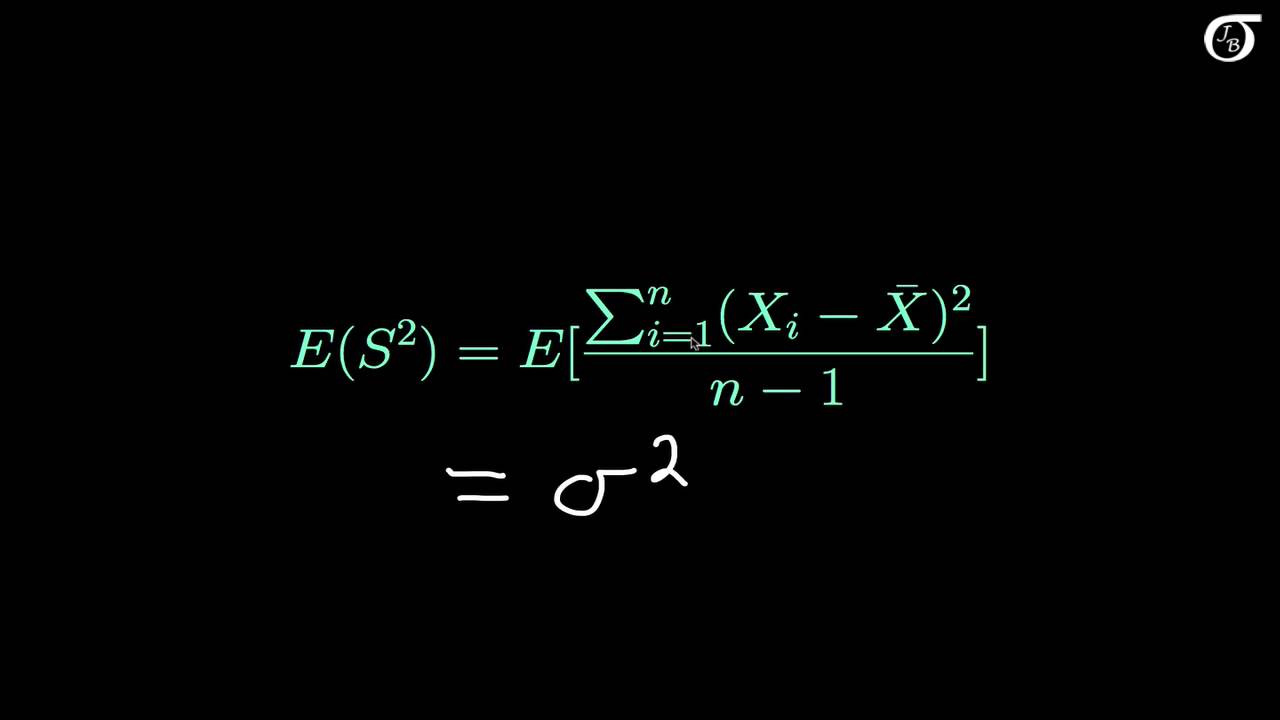
Proof that the Sample Variance is an Unbiased Estimator of the Population Variance
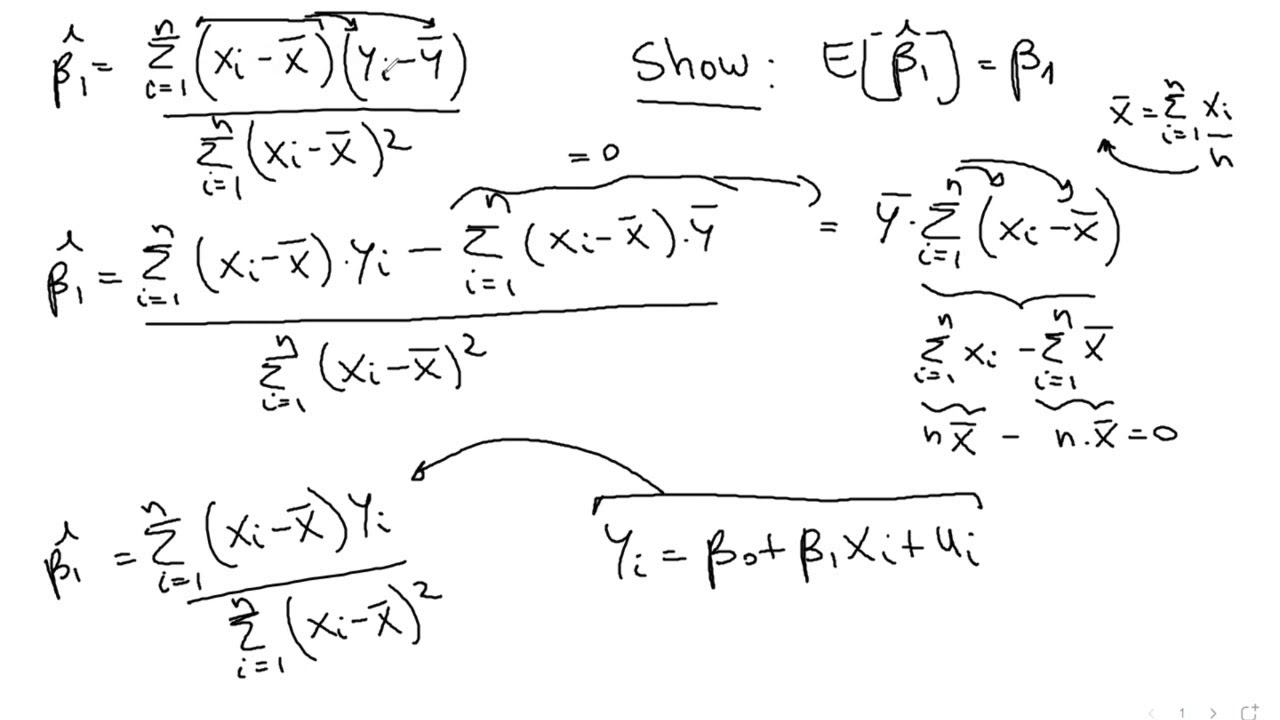
Proof ols estimator is unbiased
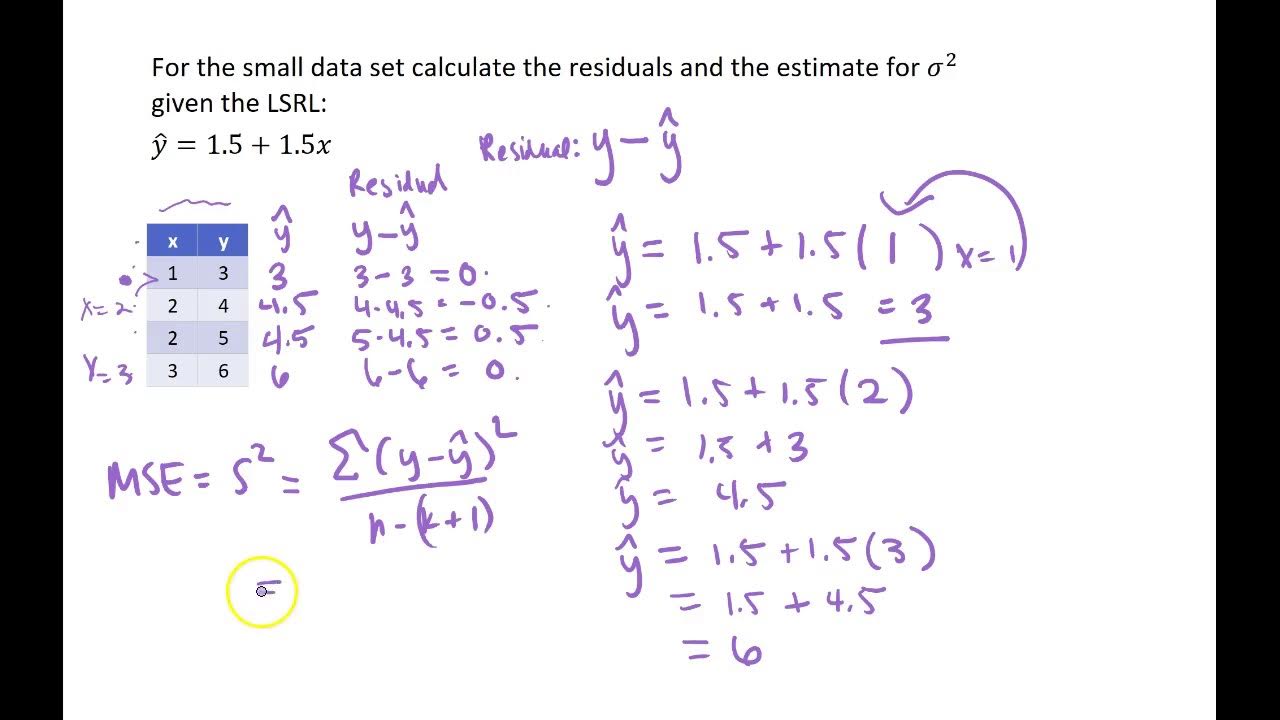
Calculating Residuals and MSE for Regression by hand
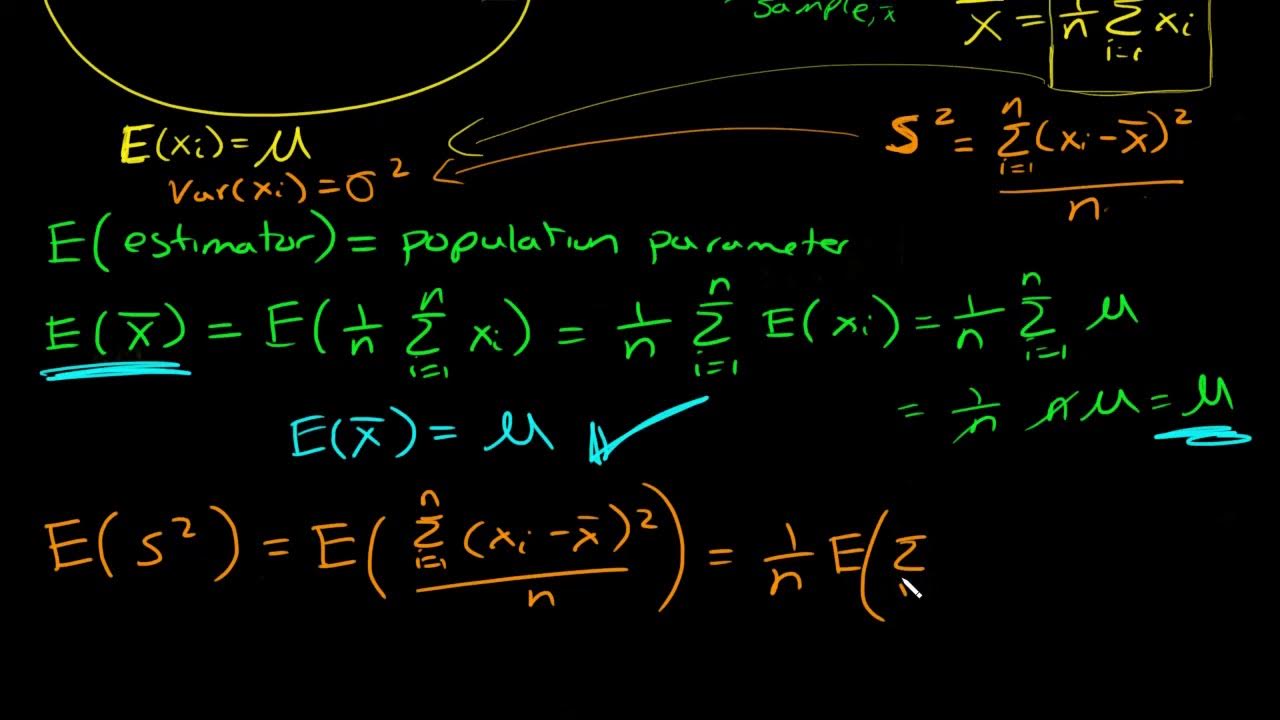
What is an unbiased estimator? Proof sample mean is unbiased and why we divide by n-1 for sample var

The Gradient of Mean Squared Error — Topic 78 of Machine Learning Foundations

Variance: Why n-1? Intuitive explanation of concept and proof (Bessel‘s correction)
5.0 / 5 (0 votes)
Thanks for rating: