Two Sample t-Test:Equal vs Unequal Variance Assumption| Statistics Tutorial #24| MarinStatsLectures
TLDRThe transcript discusses the difference between assuming equal and unequal variance in the context of two-sample t-tests and analysis of variance. It explains the concept of variability around the mean in two groups and how this affects the analysis approach. The video introduces the 'eyeball test' and formal tests like Levine's and Bartlett's for assessing equal variance assumption. It also delves into calculating the standard error for the difference in means, highlighting the importance of understanding these concepts for a deeper comprehension of statistical methods.
Takeaways
- π The discussion revolves around the choice between assuming equal variance (or standard deviation) versus non-equal variance in the context of two-sample t-tests and analysis of variance (ANOVA).
- π‘ The decision to assume equal or unequal variance hinges on the belief about the population variability in the two groups being compared.
- π The simplest approach to assess equal variance is the 'eyeball test', which involves comparing box plots of the two groups to visually estimate their variability.
- π A more quantitative method involves comparing the standard deviations directly, where a ratio greater than 2 suggests non-equal variance, while less than 2 indicates possibly equal variance.
- π§ Formal statistical tests like Levine's test and Bartlett's test can also be used to test the null hypothesis of equal population standard deviations, with the latter being sensitive to normality assumptions.
- π Understanding the properties of variance is crucial, such as the variance of the difference between two variables being equal to the sum of their variances when independent.
- π The standard error for the difference in means is derived by considering the variance of each group separately under the non-equal variance assumption.
- π Under the equal variance assumption, a pooled estimate of variance is calculated using a weighted average of the sample variances from both groups.
- π€ The choice between equal and unequal variance assumptions has implications for the precision of the standard error estimate and the underlying assumptions in statistical methods.
- π’ The degrees of freedom for the t-test differ under equal and unequal variance assumptions, with the former combining all observations to estimate common variability.
- π The assumption of equal variance is a common thread in many statistical methods, including ANOVA and linear regression, where it's important for the validity of the results.
Q & A
What is the main difference between assuming equal variance and not assuming equal variance in a two-sample t-test?
-The main difference lies in the assumption about the variability of the two groups. If equal variance is assumed, it is believed that the variability around the mean in both groups is roughly the same at the population level. If not equal variance is assumed, it is thought that one group might be more variable than the other, and the two estimates of variability are kept separate.
How can we visually assess whether the variances are equal or not?
-One can use an eyeball test by comparing box plots of the two groups to visually assess if the variability appears roughly the same or if there are significant differences between the groups.
What is the mathematical method to decide if the standard deviations of two groups are equal?
-By comparing the largest standard deviation to the smaller one, if the larger standard deviation is more than double the smallest, we work with the assumption of not equal variances. If the largest is not more than double the smallest, we can assume they are approximately equal at the population level.
What are some formal statistical tests to determine if the population standard deviations are equal?
-Levine's test and Bartlett's test are formal statistical tests that can be used to determine if the population standard deviations of two groups are equal. Bartlett's test is sensitive to departures from normality and assumes approximate normal distribution of the groups.
How is the standard error for the difference in means calculated under the assumption of not equal variances?
-The standard error for the difference in means is calculated by taking the sum of the squared sample standard deviations of each group divided by their respective sample sizes, and then taking the square root of this sum.
What is the pooled estimate in the context of equal variance assumption?
-The pooled estimate is a weighted average of the sample variances of the two groups, with each variance being weighted by its respective sample size and degrees of freedom.
How does the assumption of equal variance affect the degrees of freedom in a two-sample t-test?
-When assuming equal variance, the degrees of freedom are calculated as the sum of the sample sizes of both groups minus 2 (n1 + n2 - 2). This is different from the degrees of freedom when not assuming equal variance, which is more complex to calculate.
What are the advantages and disadvantages of assuming equal variance versus not assuming equal variance?
-Assuming equal variance has the advantage of using all available data to estimate variability, thus potentially providing a more precise estimate of the standard error for the difference in means. However, it is a stricter assumption and may not be realistic if the true population variances are not equal. Not assuming equal variance has fewer assumptions, which can be an advantage, but it may result in a less precise estimate of the standard error.
How does the assumption of equal variance apply to other statistical methods?
-The assumption of equal variance is a common thread in many statistical methods. For example, analysis of variance assumes approximately equal variability across groups, and linear regression assumes constant variability around the regression line.
Why is understanding the difference between equal and not equal variance assumptions important?
-Understanding these differences is crucial for selecting the appropriate statistical method and making accurate inferences. It helps in determining the reliability and precision of the standard error estimate, which in turn affects the validity of the conclusions drawn from the analysis.
What is the conceptual example given in the script to explain the combination of variability?
-The conceptual example given is a company's profits, which are calculated as revenue minus expenses. The variability in profits depends on the variability in both revenue (money coming in) and expenses (money going out), illustrating how the overall variability is the combination of these two components.
Outlines
π Exploring Variance Assumptions in Two-Sample T-Tests
This paragraph discusses the difference between assuming equal variances (or standard deviation) and non-equal variances in the context of two-sample t-tests and analysis of variance (ANOVA). It emphasizes the importance of determining whether the variability around the mean in two groups is roughly the same or significantly different. The paragraph introduces the concept of using an 'eyeball test' through box plots to visually assess the variability and introduces the method of comparing standard deviations to decide on the appropriate assumption. It also mentions formal tests like Levine's test and Bartlett's test, noting the latter's sensitivity to normality.
π Calculating Standard Error with Non-Equal Variances
The second paragraph delves into the calculation of the standard error for the difference in means when variances are assumed to be non-equal. It explains the process of deriving the standard error by starting with the variance of the mean for each group and combining them under the assumption of independence. The paragraph uses the concept of the sum of variances being equal to the variance of the sum to illustrate the calculation. It provides a step-by-step explanation of how to find the standard deviation for the difference in means, emphasizing the conceptual understanding of these calculations.
π Pooled Variance and Equal Variance Assumption
This paragraph focuses on the assumption of equal variances, explaining the concept of pooled variance as a weighted average of the sample variances from both groups. It details the process of calculating the standard error for the difference in means under this assumption, highlighting the use of pooled variance instead of individual group variances. The paragraph also discusses the degrees of freedom associated with this approach and contrasts it with the non-equal variance assumption. It concludes by emphasizing the importance of understanding the difference between these two assumptions and their implications in statistical methods.
Mindmap
Keywords
π‘Equal Variance
π‘Unequal Variance
π‘Standard Error
π‘Two-Sample T-Test
π‘Analysis of Variance (ANOVA)
π‘Pooled Variance
π‘Degrees of Freedom
π‘Variance
π‘Standard Deviation
π‘Eyeball Test
π‘Formal Tests
Highlights
The discussion focuses on the difference between assuming equal variance and non-equal variance in population level for two-sample t-tests and analysis of variance.
The main question is whether the variability around the mean in two groups is roughly the same or significantly different at the population level.
The approach to analysis depends on the assumption of equal variability between the two groups.
The simplest approach to determine equal variance is the eyeball test, using box plots to visually assess the variability between the two groups.
A more quantitative method involves comparing the largest standard deviation to the smallest; if the largest is more than double the smallest, the assumption of equal variance may not hold.
Formal tests such as Levine's test and Bartlett's test can be used to determine if the population standard deviations are equal.
Bartlett's test is sensitive to departures from normality and assumes approximate normal distribution of the groups.
The standard error for the difference in means is derived, first under the assumption of non-equal variances.
The variance of the difference in two variables is equal to the sum of their variances if they are independent.
A conceptual example is given, relating the variability of profits to the variability of revenue and expenses.
The standard error for the difference in means is calculated by summing the variances of the two groups and taking the square root.
Assuming equal variances involves calculating a pooled estimate, which is a weighted average of the two sample variances.
The pooled estimate is used to calculate a more reliable standard error for the difference in means under the equal variance assumption.
The degrees of freedom for the equal variance assumption is n1 + n2 - 2, combining all data to estimate the common variance.
The assumption of equal variance is stricter and adds an additional assumption that may not always be realistic.
The equal variance assumption allows for a more precise estimate of the standard error for the difference in means, using all data points.
The assumption of equal variance is foundational in many statistical methods, including analysis of variance and linear regression.
The transcript emphasizes the importance of understanding the conceptual differences between these two assumptions rather than just the calculations.
Transcripts
Browse More Related Video

Range, variance and standard deviation as measures of dispersion | Khan Academy
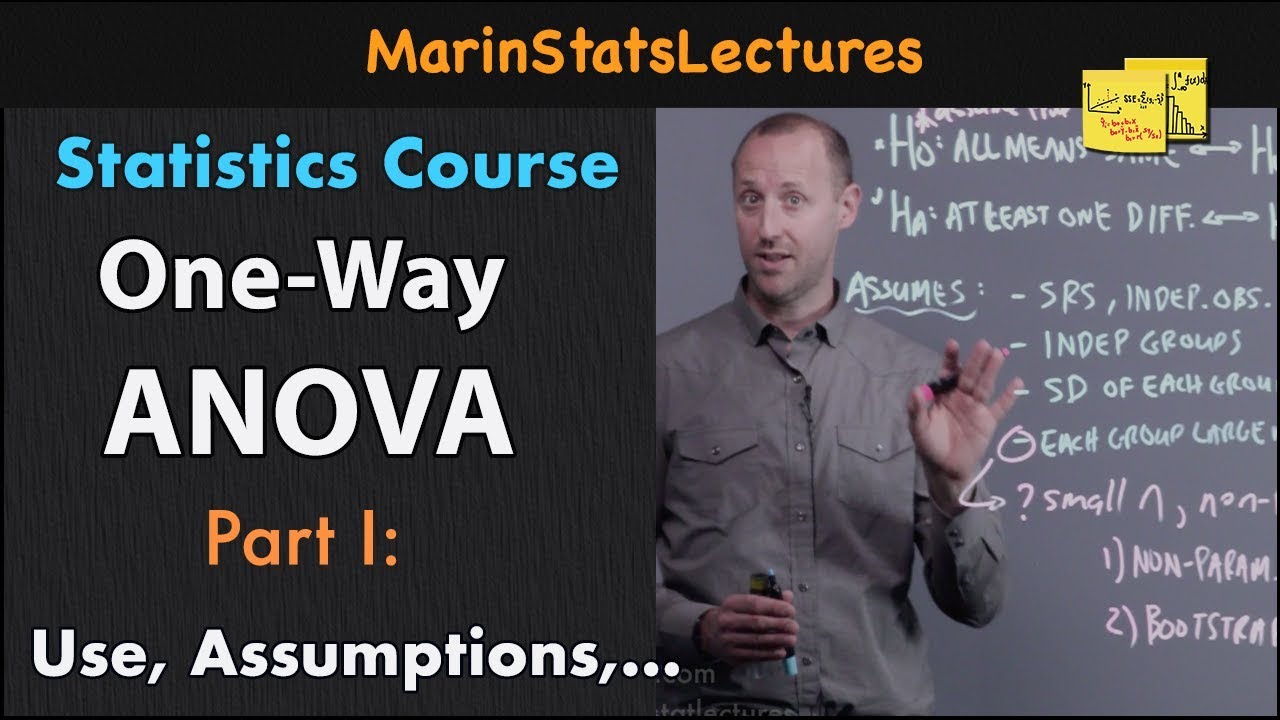
One Way ANOVA (Analysis of Variance): Introduction | Statistics Tutorial #25 | MarinStatsLectures
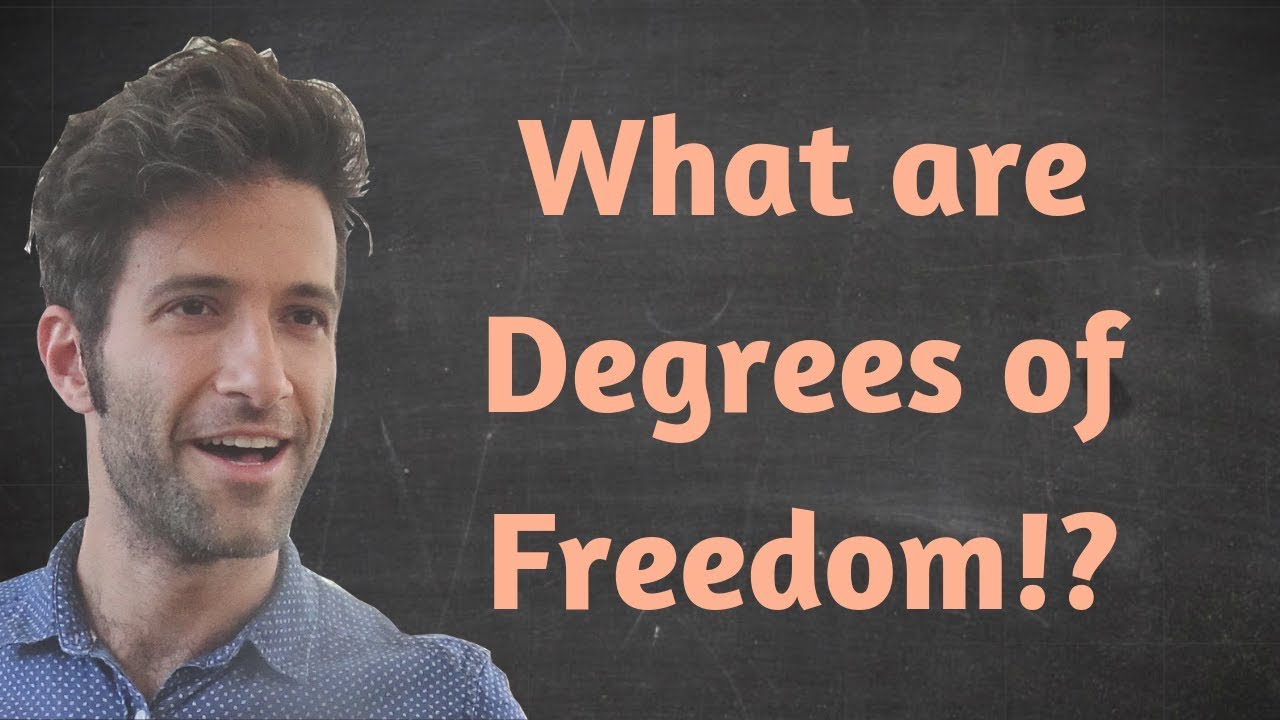
What are degrees of freedom?!? Seriously.
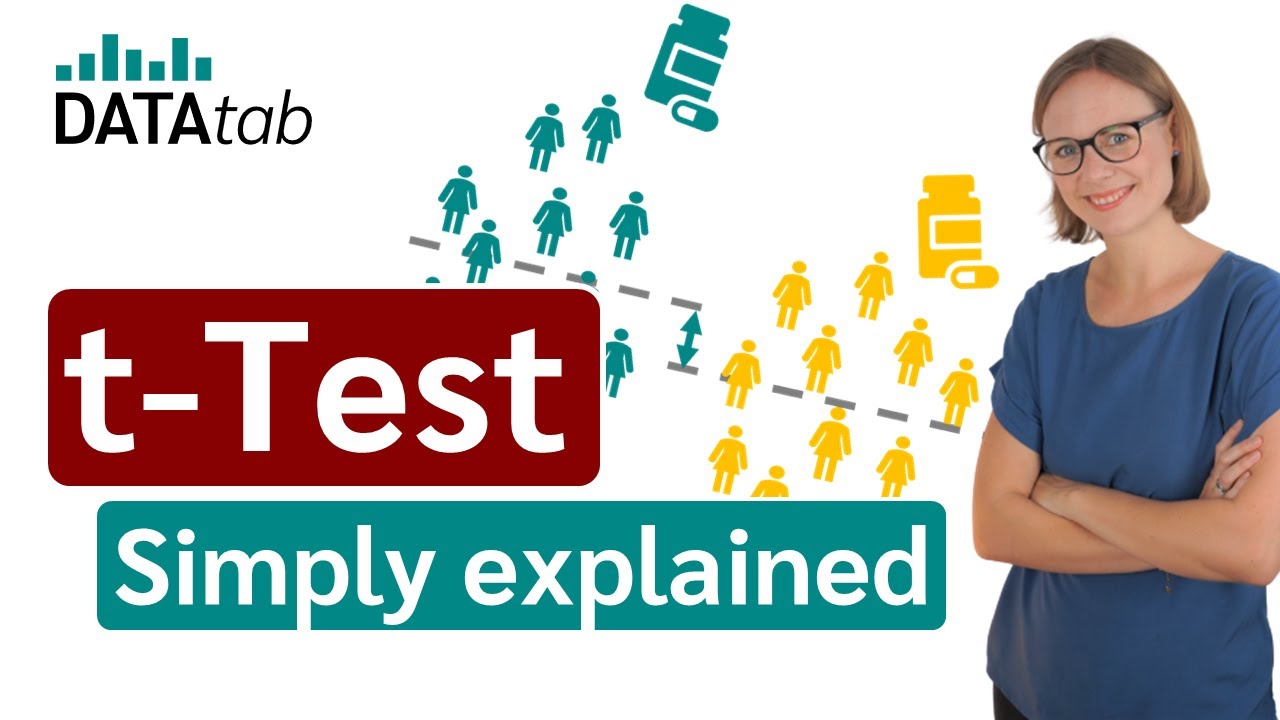
t-Test - Full Course - Everything you need to know

Measures of Dispersion (Ungrouped Data) | Basic Statistics
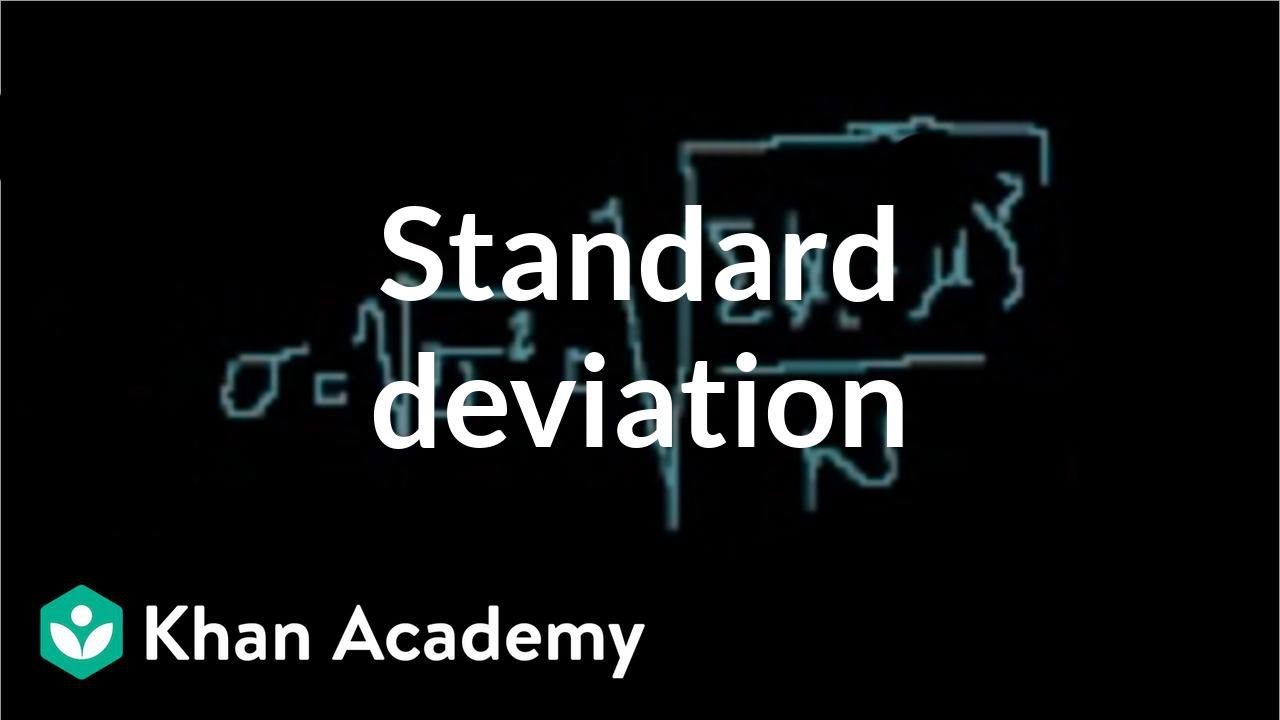
Statistics: Standard deviation | Descriptive statistics | Probability and Statistics | Khan Academy
5.0 / 5 (0 votes)
Thanks for rating: