Sensitivity and Specificity for the USMLE
TLDRThis transcript delves into the application of biostatistics, focusing on sensitivity and specificity in diagnostic testing. It illustrates these concepts using hypothetical tests for syphilis and hepatocellular carcinoma, explaining how to calculate and interpret these metrics. The discussion includes the trade-offs between sensitivity and specificity, the importance of choosing appropriate cutoff values for tests, and the clinical implications of ruling in or out diseases. The transcript also addresses how to calculate false negatives and false positives, providing practical examples to reinforce understanding.
Takeaways
- π Biostatistics can be divided into three arms: performing the study, evaluating the study quality, and applying the study clinically.
- π Sensitivity refers to the test's ability to correctly identify those with the disease (true positives / (true positives + false negatives)).
- π Specificity is the test's ability to correctly identify those without the disease (true negatives / (true negatives + false positives)).
- π₯ In clinical practice, a test with high sensitivity is preferred for screening to ensure most cases are detected, while a test with high specificity is used for confirmation to avoid misdiagnosis.
- π©Έ For example, Test A with 90% sensitivity will detect 9 out of 10 syphilis patients, making it more effective at catching the disease than Test B with 20% sensitivity.
- π¬ The 2x2 table is a useful tool for visualizing and calculating sensitivity and specificity in a study.
- π The ideal diagnostic test has 100% sensitivity and 100% specificity, but in reality, there is often a trade-off between the two.
- π The choice of diagnostic cutoff value affects sensitivity and specificity; moving the cutoff affects one while inversely affecting the other.
- π« False negatives occur when the test fails to detect the disease in those who have it, while false positives incorrectly identify healthy individuals as having the disease.
- π To calculate false positives: 1 - specificity, and to calculate false negatives: 1 - sensitivity.
- π‘ A test that is 100% specific means that a positive result rules in the disease, while a test that is 100% sensitive means that a negative result rules out the disease.
Q & A
What are the three arms of biostatistics mentioned in the transcript?
-The three arms of biostatistics are performing the study, evaluating the study quality, and applying the study clinically.
What is the sensitivity of Test A in the given example of syphilis screening tests?
-The sensitivity of Test A is 90%. This means that Test A correctly identifies 9 out of every 10 syphilis patients as positive.
How is specificity defined in the context of diagnostic tests?
-Specificity is defined as the probability that a diagnostic test will yield a negative result for individuals who do not have the disease. It is calculated by dividing the true negatives by the sum of true negatives and false positives.
What is the specificity of Test A in the transcript example?
-The specificity of Test A is approximately 33%. This indicates that only 5 out of 15 non-syphilis patients tested negative, while 10 tested positive (false positives).
How is a two-by-two table used to calculate sensitivity and specificity?
-A two-by-two table is used by categorizing patients into four groups based on their disease status (diseased or not) and test results (positive or negative). Sensitivity is calculated by dividing the true positives (diseased and tested positive) by the sum of true positives and false negatives. Specificity is calculated by dividing the true negatives (not diseased and tested negative) by the sum of true negatives and false positives.
What is the sensitivity of Test X for diagnosing hepatocellular carcinoma (HCC) based on the given data?
-The sensitivity of Test X for diagnosing HCC is 40%. This means that Test X correctly identifies 100 out of 250 HCC patients as positive.
What is the specificity of Test X for diagnosing HCC, and what does this imply for disease-free patients?
-The specificity of Test X for diagnosing HCC is 100%. This implies that all 550 disease-free patients tested negative, indicating that the test has no false positives.
How does the cutoff value for a diagnostic test affect its sensitivity and specificity?
-Adjusting the cutoff value affects both sensitivity and specificity inversely. Moving the cutoff value to the right (higher values indicating positive) increases specificity (more true negatives) but decreases sensitivity (fewer true positives). Conversely, moving the cutoff value to the left (lower values indicating positive) increases sensitivity (more true positives) but decreases specificity (more false positives).
What does a 100% specific test mean in clinical practice?
-A 100% specific test means that if a patient tests positive, they definitely have the disease. This is because a test with 100% specificity has no false positives, so every positive result can be confidently attributed to the presence of the disease.
What does a 100% sensitive test mean in clinical practice?
-A 100% sensitive test means that if a patient tests negative, they definitely do not have the disease. This is because a test with 100% sensitivity will correctly identify all diseased patients, so a negative result can confidently rule out the presence of the disease.
What is the typical clinical pattern for screening and confirming a disease?
-The typical clinical pattern is to first screen with a highly sensitive test to catch as many disease cases as possible, and then confirm the diagnosis with a highly specific test to ensure that the identified cases are truly positive.
How can you calculate the number of false negatives for a diagnostic test?
-To calculate the number of false negatives, you subtract the sensitivity of the test from 1 (or 100%). This gives you the false negative rate, which is the proportion of diseased patients who are incorrectly identified as not having the disease.
How can you calculate the number of false positives for a diagnostic test?
-To calculate the number of false positives, you subtract the specificity of the test from 1 (or 100%). This gives you the false positive rate, which is the proportion of disease-free patients who are incorrectly identified as having the disease.
Outlines
π Understanding Sensitivity and Specificity in Biostatistics
This section introduces the application of biostatistics in clinical settings, focusing on sensitivity and specificity using syphilis screening tests as examples. Sensitivity measures the percentage of true positives correctly identified by the test, demonstrated by comparing Test A and Test B, where Test A shows higher sensitivity (90%) than Test B (20%). The concept of specificity is introduced through a scenario involving non-syphilis patients, with an emphasis on the importance of accurately identifying true negatives to avoid false positives. The use of a two-by-two table is explained for calculating these metrics, with sensitivity defined as the probability of testing positive if the disease is present, and specificity as the probability of testing negative if the disease is absent.
π Applying Sensitivity and Specificity to Hepatocellular Carcinoma Screening
This paragraph delves into the application of sensitivity and specificity calculations in the context of screening for Hepatocellular Carcinoma (HCC) using Test X. A detailed example involving 800 patients, of which 250 have confirmed HCC, is used to calculate the sensitivity of Test X at 40%. The paragraph further explores specificity calculation, demonstrating a scenario where Test X achieves 100% specificity, meaning all non-disease patients were correctly identified. The theoretical understanding of sensitivity and specificity is further enhanced by introducing how these concepts are visually represented on a graph, discussing the ideal but unrealistic scenario of a test having both 100% sensitivity and specificity.
π Diagnostic Test Efficacy: Sensitivity, Specificity, and Cutoff Values
The focus shifts to the practical implications of adjusting cutoff values on the sensitivity and specificity of diagnostic tests, using rheumatoid arthritis and the serum level of rheumatoid factor as an example. It details how changing the cutoff value affects the rates of true positives, true negatives, false positives, and false negatives, demonstrating the trade-off between sensitivity and specificity. This section illustrates the concept through graphs, showing the impact of moving the cutoff value to include more true positives (increasing sensitivity but decreasing specificity) or to exclude false positives (increasing specificity but decreasing sensitivity).
π Adjusting Diagnostic Test Cutoffs: Impacts on Sensitivity and Specificity
This segment explains the consequences of adjusting the cutoff value on a diagnostic test's sensitivity and specificity through theoretical graphs. It uses the diagnostic test Y, with a sensitivity of 40% and specificity of 100%, as a case study to show how the position of the cutoff value affects the classification of patients as positive or negative. The discussion further clarifies how moving the cutoff value can lead to changes in true positive and true negative rates, ultimately impacting the test's ability to correctly identify diseased and non-diseased individuals. The segment concludes by highlighting how specific cutoff values are chosen based on the desired balance between sensitivity and specificity.
π¬ Rule-in and Rule-out: Making Clinical Decisions with Diagnostic Tests
The final section explores the clinical application of diagnostic tests, focusing on the concepts of 'rule-in' and 'rule-out' diseases based on test sensitivity and specificity. It discusses how tests with high specificity can confirm disease presence (spin), while those with high sensitivity can exclude it (snout). The paragraph also touches on the strategy of using a highly sensitive test for initial screening followed by a highly specific test for confirmation. Additionally, it covers the calculation of false positives and negatives, offering a practical example involving a screening test for hyperlipidemia among obese patients, illustrating how to calculate the number of false positives based on the test's specificity.
Mindmap
Keywords
π‘Biostatistics
π‘Sensitivity
π‘Specificity
π‘True Positives
π‘False Negatives
π‘True Negatives
π‘False Positives
π‘Two-by-Two Table
π‘Diagnostic Test
π‘Hepatocellular Carcinoma (HCC)
π‘Rheumatoid Factor (RF)
Highlights
Introduction to applying biostatistics, focusing on sensitivity and specificity.
Comparison between Test A and Test B for screening syphilis, highlighting Test A's higher sensitivity.
The concept of specificity introduced through the performance of Test A among non-syphilis patients.
Explanation of calculating sensitivity and specificity using a two-by-two table.
Mathematical definitions of sensitivity and specificity with formulas.
Application example using Test X for diagnosing hepatocellular carcinoma to calculate sensitivity.
Determining specificity of Test X with a detailed calculation example.
Introduction to graphical representation of sensitivity and specificity.
Demonstration of ideal and realistic scenarios of diagnostic tests' performance on a graph.
Exploring the impact of changing cutoff values on sensitivity and specificity.
Clarification of diagnostic tests' sensitivity and specificity through graphical examples.
Discussion on tests that can 'rule in' and 'rule out' disease, including 'SPIN' and 'SNOUT' mnemonics.
Question application on using a sensitive test for ruling out a disease.
Calculating false negatives and positives with examples.
Practical application question on calculating false positives in a hyperlipidemia screening test.
Transcripts
Browse More Related Video
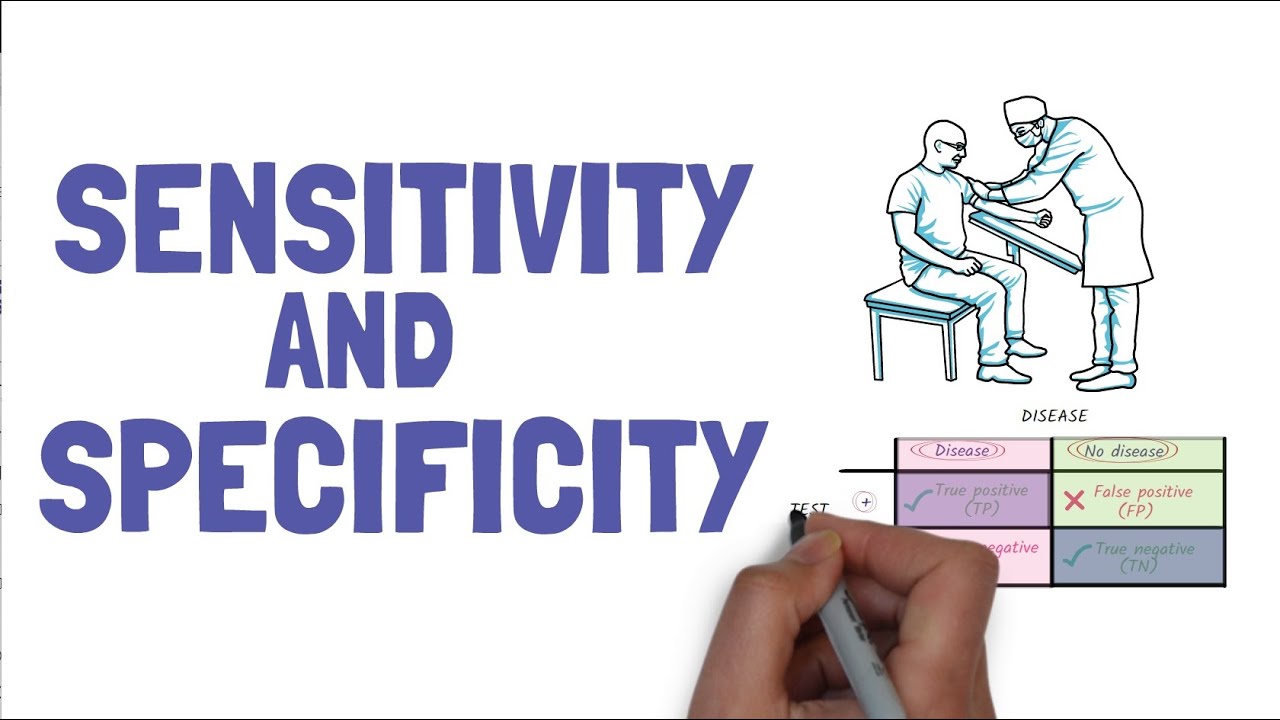
Sensitivity and Specificity simplified
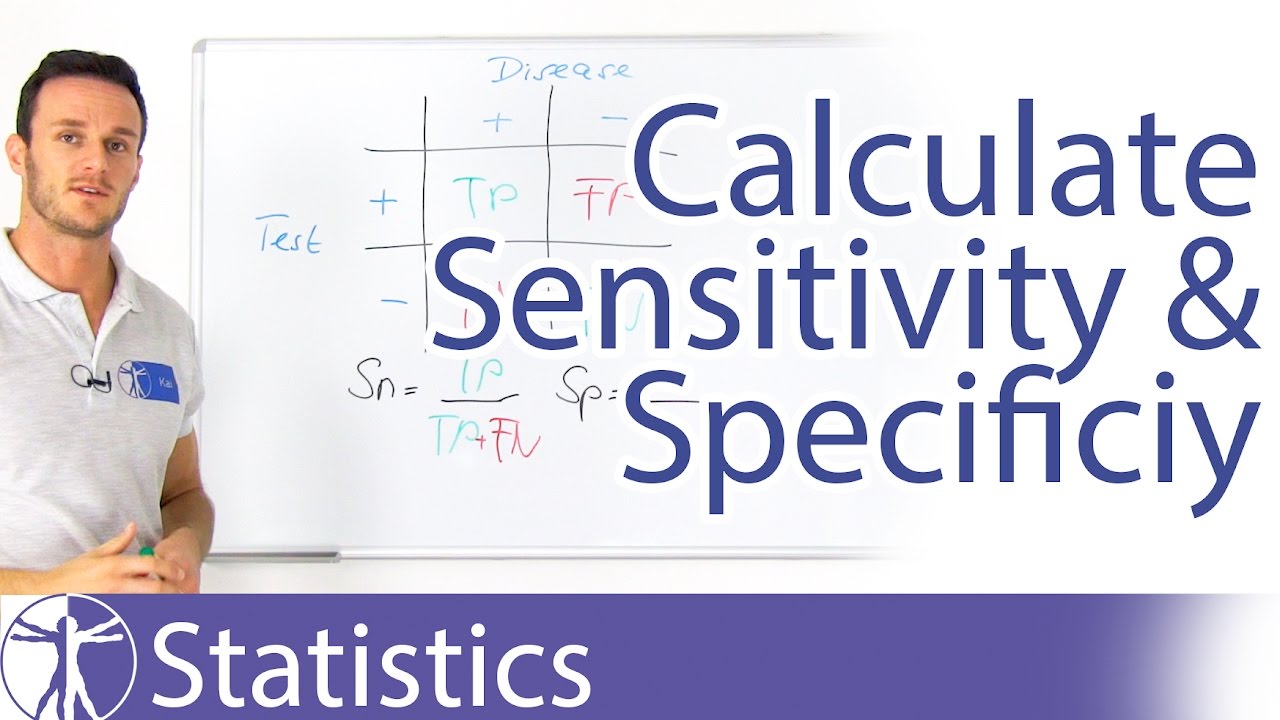
How to calculate Sensitivity and Specificity
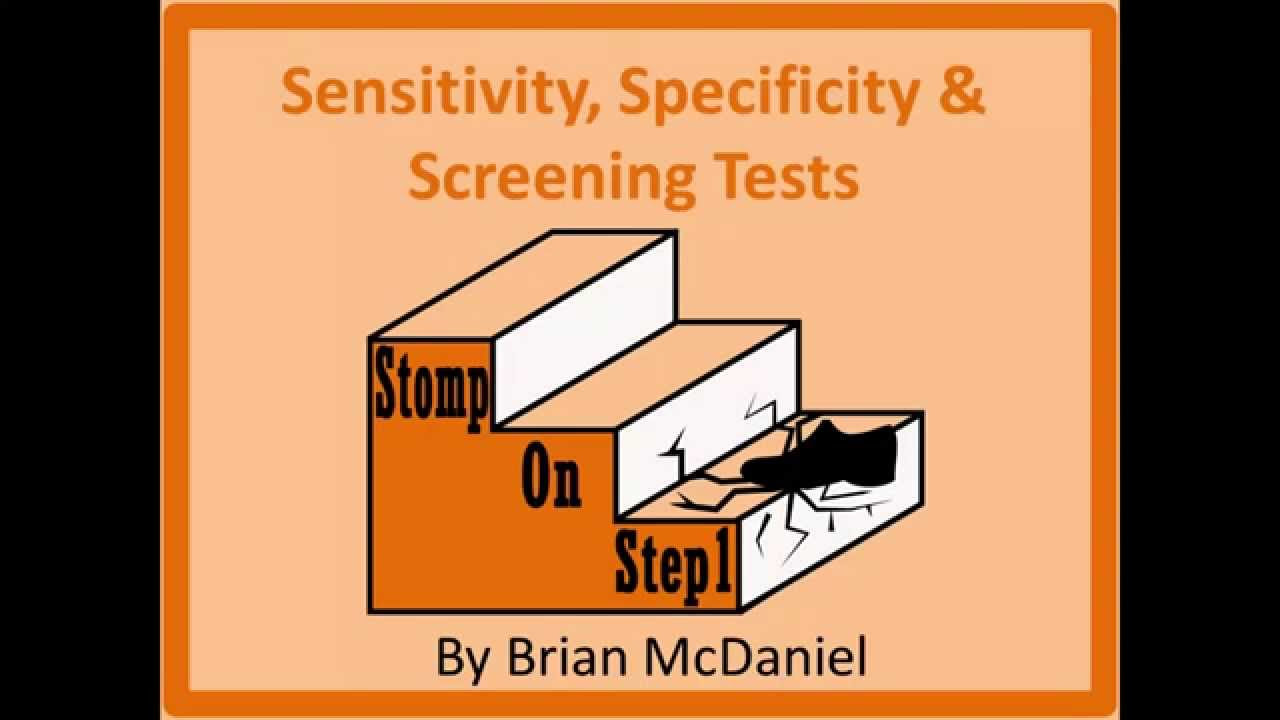
Sensitivity, Specificity, Screening Tests & Confirmatory Tests

Sensitivity and Specificity - Part 1
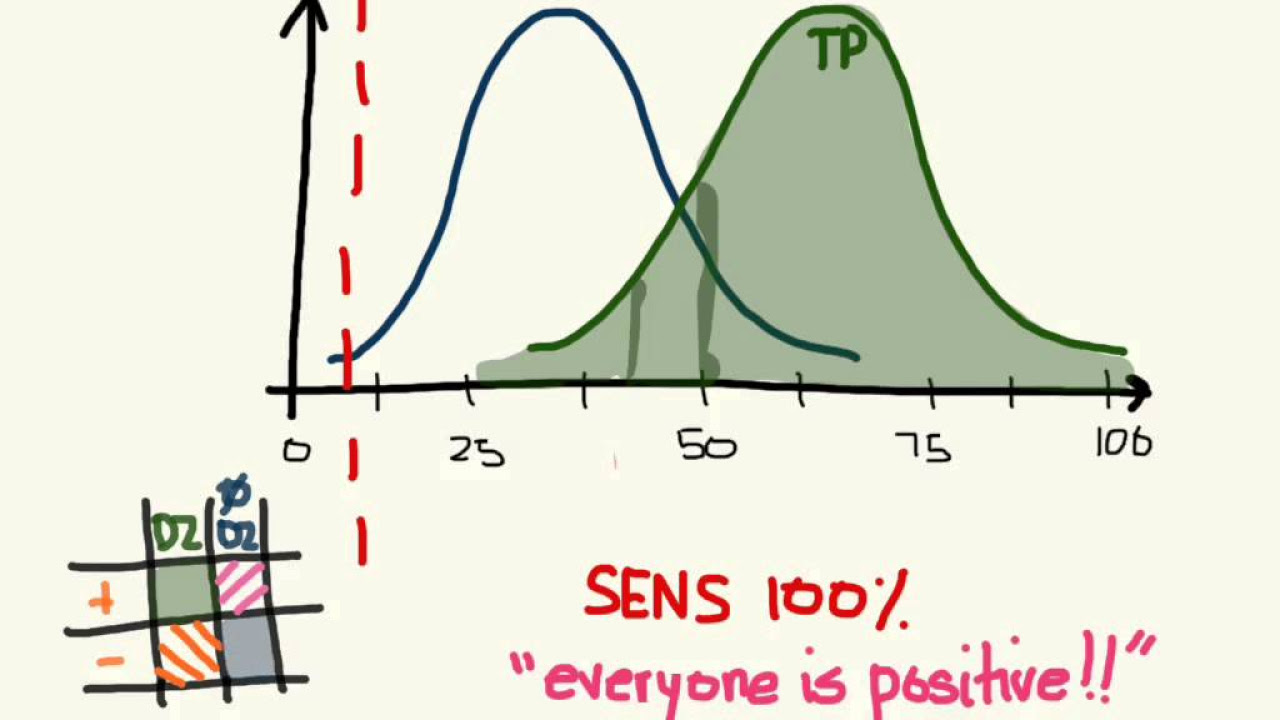
The tradeoff between sensitivity and specificity
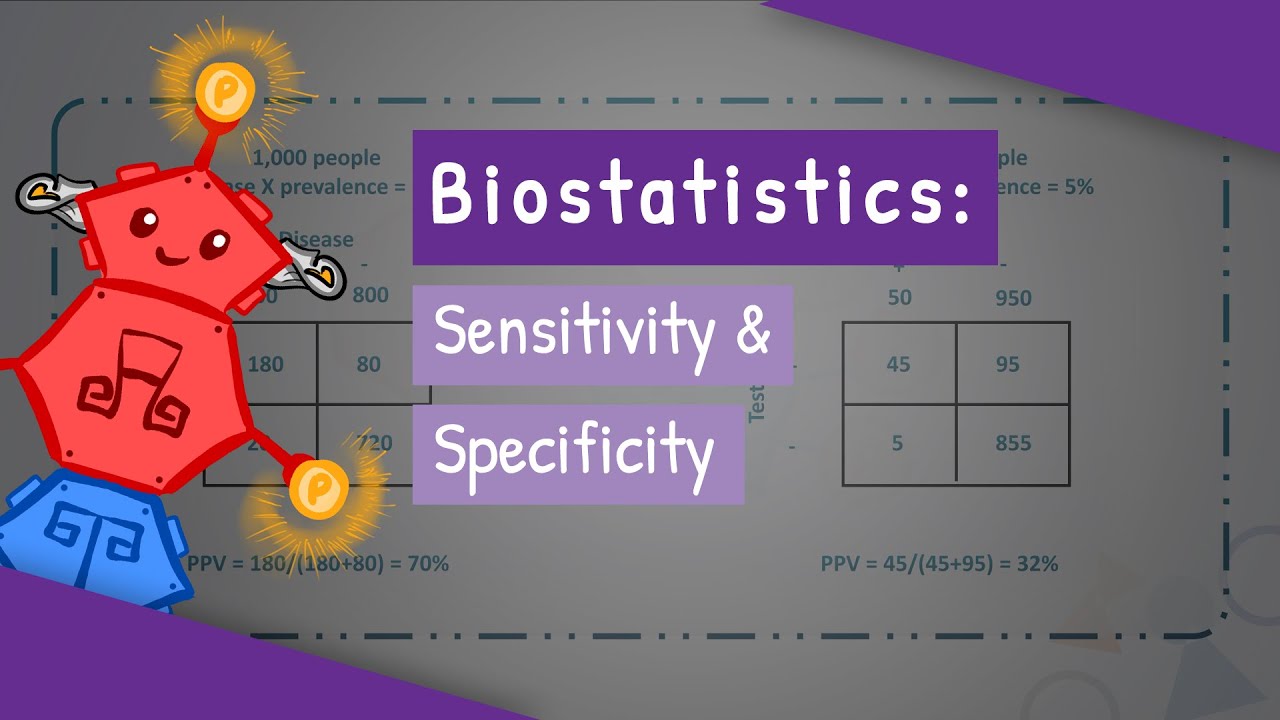
Biostatistics - Evaluation of Diagnostic Tests: Sensitivity & Specificity
5.0 / 5 (0 votes)
Thanks for rating: