Mean (expected value) of a discrete random variable | AP Statistics | Khan Academy
TLDRThis video script introduces the concept of expected value for a discrete random variable, exemplified by the number of weekly workouts. It explains that the expected value, denoted by the Greek letter mu, is a weighted sum of all possible outcomes, each multiplied by their respective probabilities. The script demonstrates the calculation of the expected number of workouts per week as 2.1, clarifying that this non-integer value is useful for predicting the average number of workouts over a longer period, even though individual outcomes are whole numbers.
Takeaways
- ๐ The instructor introduces a random variable 'x' to represent the number of workouts in a week.
- ๐ The probability distribution table for 'x' is provided, showing 'x' can only take finite values from zero to four.
- ๐ฒ 'x' is classified as a discrete random variable because it has a finite number of possible outcomes.
- ๐ข The probability distribution is valid as the sum of probabilities equals one and no probability is negative.
- ๐งฎ The concept of expected value for a discrete random variable is introduced, denoted by the Greek letter mu (ฮผ).
- ๐ค The expected value gives an idea of the mean or average number of workouts expected in a week.
- ๐ To calculate the expected value, a weighted sum of outcomes is used, where each outcome is multiplied by its probability.
- ๐ The calculation involves multiplying each possible number of workouts by its corresponding probability and then summing these products.
- ๐ก The result of the calculation shows an expected value of 2.1 workouts per week, which is a non-integer.
- ๐๏ธโโ๏ธ The expected value does not imply that exactly 2.1 workouts will occur but suggests an average over a longer period.
- ๐ Even with integer outcomes, a random variable can have a non-integer expected value, which is useful for predictions over many trials.
Q & A
What is the random variable x defined as in the script?
-The random variable x is defined as the number of workouts that the instructor will do in a given week.
Why is x considered a discrete random variable?
-X is considered a discrete random variable because it can only take on a finite number of values, specifically zero, one, two, three, or four.
How is the probability distribution for x described in the script?
-The probability distribution for x is described through a table that lists the possible outcomes and their corresponding probabilities.
What is the condition for a probability distribution to be valid as per the script?
-A probability distribution is valid if the combined probabilities of all outcomes add up to one and none of the probabilities are negative.
What is the expected value of a discrete random variable?
-The expected value of a discrete random variable is the mean or average of all possible outcomes, weighted by their respective probabilities.
How is the expected value of x computed in the script?
-The expected value of x is computed by taking the weighted sum of the various outcomes, where each outcome is multiplied by its probability and then all the results are added together.
What does the Greek letter mu represent in the context of the script?
-In the context of the script, the Greek letter mu (ยต) represents the mean of the random variable x.
What is the expected number of workouts per week according to the computed expected value?
-The expected number of workouts per week, given the probability distribution, is 2.1.
How does the script explain the concept of having a non-integer expected value for a variable with only integer outcomes?
-The script explains that even though the variable can only take on integer values, the expected value can be non-integer. It is useful for predicting the average number of workouts over a larger number of weeks, such as 10 or 100.
What is the significance of the expected value in understanding the instructor's workout habits over time?
-The expected value provides an average measure of the instructor's workouts over a week. It helps in understanding the long-term pattern of workouts, such as predicting the total number of workouts over 10 or 100 weeks.
How does the script illustrate the concept of expected value with an example?
-The script illustrates the concept of expected value by calculating the expected number of workouts per week as 2.1, using the weighted sum of outcomes (0, 1, 2, 3, 4) multiplied by their respective probabilities (0.1, 0.15, 0.4, 0.25, 0.1).
Outlines
๐๏ธโโ๏ธ Understanding Discrete Random Variables and Expected Value
The instructor introduces a discrete random variable 'x', representing the number of weekly workouts, which can only take on a finite set of integer values (0 to 4). A table is presented showing the probability distribution of 'x', ensuring the probabilities are non-negative and sum up to one, validating the distribution. The concept of expected value is explained as the mean of the random variable, denoted by the Greek letter mu (ฮผ). The expected value is calculated by taking a weighted sum of the possible outcomes, each multiplied by their respective probabilities, resulting in an expected number of workouts per week. The example provided calculates this to be 2.1, illustrating that even though 'x' is an integer, the expected value can be non-integer, providing insight into the average number of workouts over a longer period.
Mindmap
Keywords
๐กRandom Variable
๐กProbability Distribution
๐กDiscrete Random Variable
๐กExpected Value
๐กMean
๐กWeighted Sum
๐กNon-Negative Probabilities
๐กInteger Values
๐กContextual Application
๐กGreek Letter Mu (ฮผ)
Highlights
Introduction of the discrete random variable 'x' representing the number of workouts in a week.
Description of the probability distribution table for 'x' with finite values from zero to four.
Explanation of a valid probability distribution with non-negative probabilities summing to one.
Introduction of the concept of expected value for a discrete random variable.
Clarification that the expected value is also known as the mean of a random variable.
Use of the Greek letter mu (ฮผ) to denote the mean of the random variable 'x'.
Methodology to compute the expected value as a weighted sum of outcomes.
Illustration of the calculation process for the expected value with given probabilities.
Simplification of the expected value calculation by eliminating the zero outcome.
Multiplication of each outcome by its corresponding probability to find the weighted sum.
Addition of the weighted outcomes to find the total expected value of 2.1 workouts per week.
Discussion on the interpretation of a non-integer expected value for a variable with integer outcomes.
Explanation of how the expected value can be applied to predict the number of workouts over a longer period.
Clarification that the expected value does not imply exact outcomes but provides a predictive average.
Highlighting the usefulness of expected value in understanding the average behavior of a random variable.
Emphasis on the practical application of expected value in predicting long-term outcomes of random events.
Transcripts
Browse More Related Video
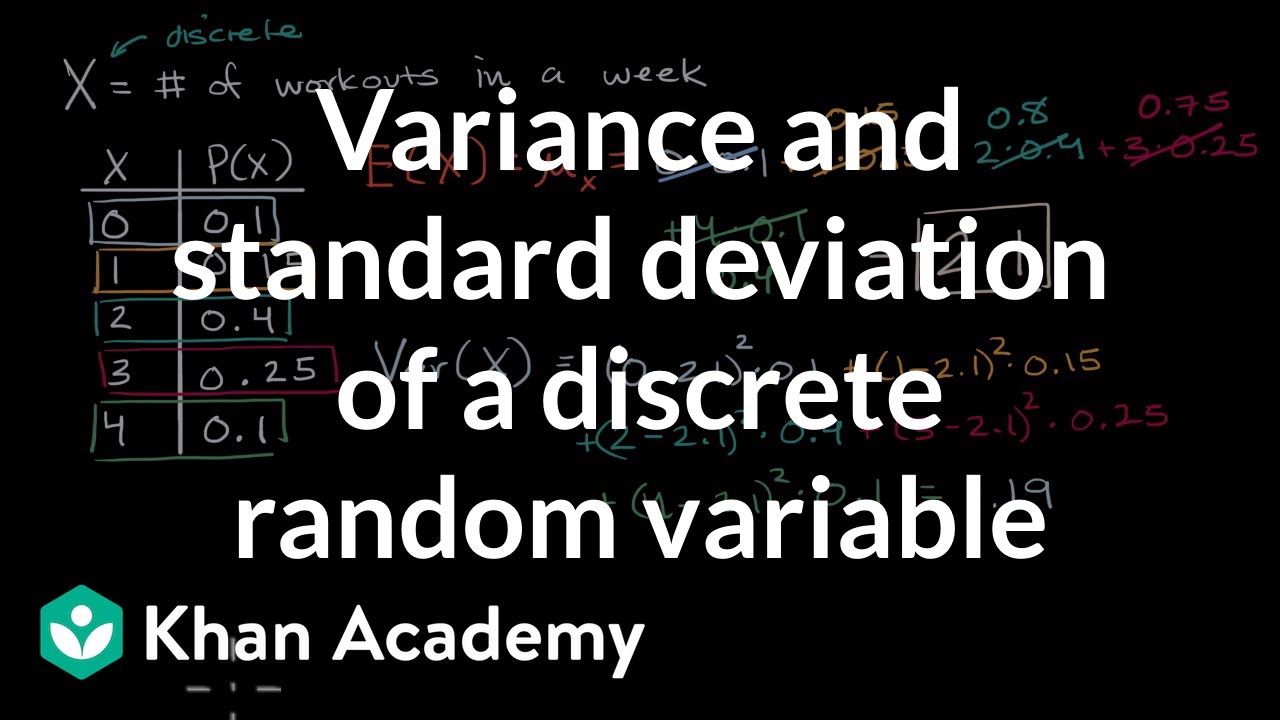
Variance and standard deviation of a discrete random variable | AP Statistics | Khan Academy
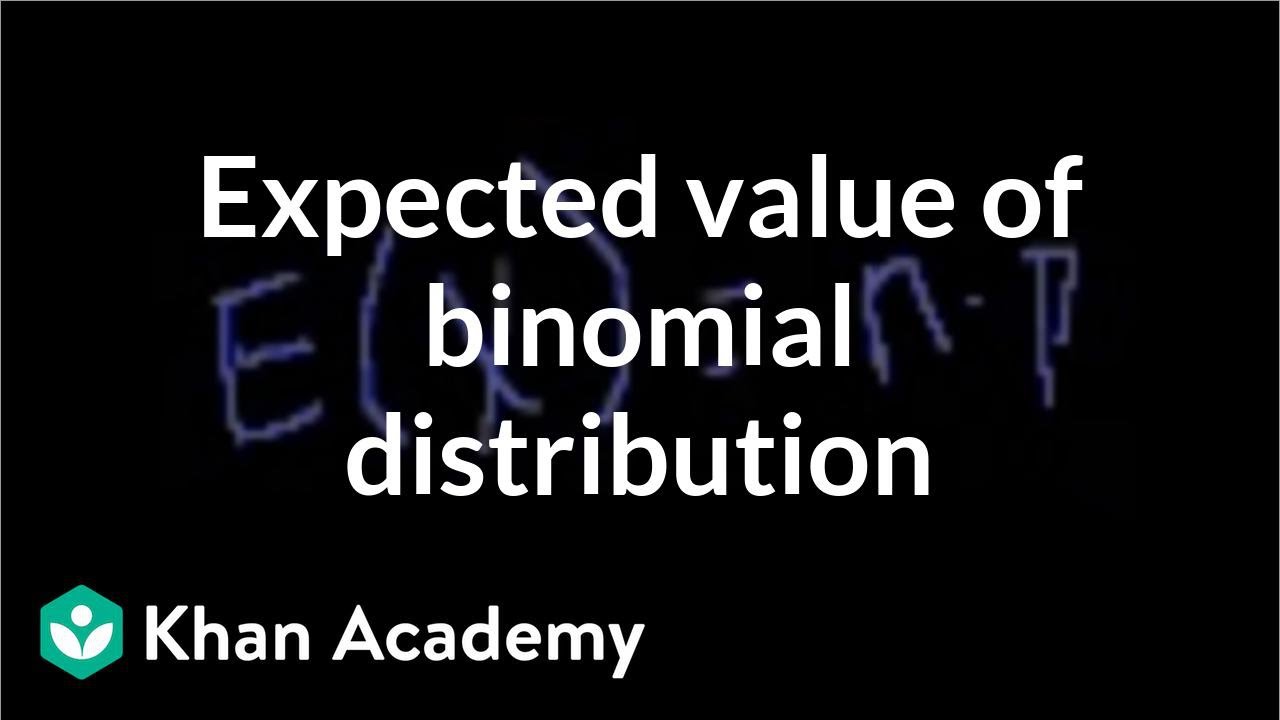
Expected value of binomial distribution | Probability and Statistics | Khan Academy
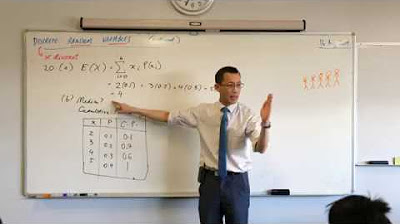
Discrete Random Variables (1 of 3: Expected value & median)
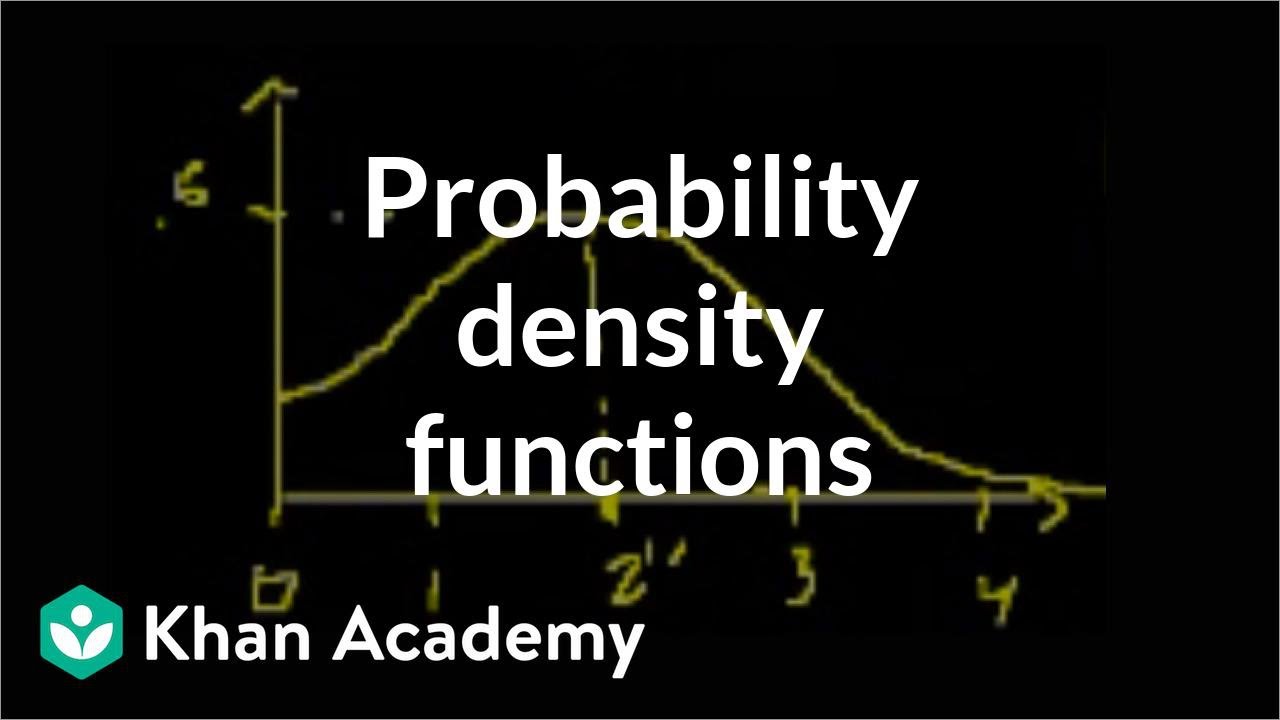
Probability density functions | Probability and Statistics | Khan Academy

5.1.6 Discrete Probability Distributions - Expected Value and Decision Theory

Bernoulli Distribution
5.0 / 5 (0 votes)
Thanks for rating: