How to Learn Probability Distributions
TLDRIn this video, the host explores the most effective way to understand probability distributions by using an analogy of memorizing a complex pattern. He argues that focusing on the stories that connect various distributions, rather than their individual definitions, simplifies the learning process. The video illustrates how several distributions, including the Bernoulli, Geometric, Negative Binomial, Binomial, Exponential, Gamma, and Poisson, are related through simple counting rules and continuous analogies, providing insights that make them easier to remember and apply.
Takeaways
- π§ The video emphasizes the importance of finding simple patterns to understand complex concepts, such as probability distributions.
- π It introduces an analogy with a complex diagram to illustrate the idea that recognizing patterns makes memorization and understanding easier.
- π The presenter suggests focusing on the stories that relate different probability distributions rather than their individual definitions.
- π’ The script explains how different probability distributions, like Bernoulli, Geometric, Negative Binomial, Binomial, Exponential, Gamma, and Poisson, are related through simple counting rules.
- π It uses the concept of 'thinning' a distribution to transition from discrete to continuous distributions, providing an intuitive understanding of their parameters.
- π The video highlights the discrete-to-continuous analogies, such as the Exponential being the continuous version of the Geometric, and the Gamma being the continuous version of the Negative Binomial.
- π The script discusses summation relationships between distributions, showing how summing certain distributions results in others, like the Negative Binomial being a sum of Geometric distributions.
- π It argues that understanding these relationships provides additional properties of the distributions almost for free, without needing to memorize them individually.
- π The presenter shares personal stories and examples that helped demystify certain distributions, such as the Student's t-distribution, Laplace distribution, and Cauchy distribution.
- π The video mentions a comprehensive graph and a dedicated website that map out the relationships between various probability distributions, suggesting it as a resource for further exploration.
- π The video concludes by encouraging viewers to learn about these relational stories as a more efficient way to understand a multitude of probability distributions.
Q & A
What is the main teaching strategy suggested by the speaker for learning about probability distributions?
-The speaker suggests learning about probability distributions by focusing on the stories that relate them rather than memorizing their individual definitions. This approach helps in understanding the patterns and relationships between different distributions.
How does the analogy of memorizing a complex pattern relate to learning probability distributions?
-The analogy illustrates that it's easier to remember a complex pattern if you understand the simple rules behind it. Similarly, understanding the underlying stories or patterns in probability distributions makes it easier to grasp their properties and relationships.
What is the Bernoulli distribution and what parameter does it have?
-The Bernoulli distribution is the simplest random variable that yields one of two outcomes. It has one parameter, the probability 'p' of one of the outcomes occurring, which in the script is given as 0.3.
How is the Geometric distribution related to the Bernoulli distribution?
-The Geometric distribution is related to the Bernoulli distribution by counting the number of trials needed to get a success (blue box), and it carries the same parameterization as the Bernoulli distribution, which is the probability 'p' of success.
What is the Negative Binomial distribution and how is it derived from the Bernoulli distribution?
-The Negative Binomial distribution is derived from the Bernoulli distribution by drawing lines over every 'r'th blue box and counting the number of yellow boxes between them. It has a parameter 'r' which equals the number of trials between successes.
How does the Binomial distribution differ from the Geometric and Negative Binomial distributions?
-The Binomial distribution differs by counting the number of successes (blue boxes) in a fixed number of trials (separated by lines every six boxes in the example), whereas the Geometric counts the number of trials to the first success and the Negative Binomial counts the number of trials between a fixed number of successes.
What is the continuous version of the Geometric distribution and how is it derived?
-The continuous version of the Geometric distribution is the Exponential distribution. It is derived by dividing the probability of a blue box by a large positive number 'c' and summing the boxes between all blue boxes, where each box now counts for 1 over 'c'.
What is the relationship between the Gamma distribution and the Negative Binomial distribution?
-The Gamma distribution can be viewed as a continuous version and a generalization of the Negative Binomial distribution. It is derived by summing up exponential distributions, analogous to summing up geometric distributions to get the Negative Binomial.
How is the Poisson distribution related to the Binomial distribution?
-The Poisson distribution is the continuous version of the Binomial distribution. It is derived by counting the number of blue boxes between blocks that sum to a fixed number, in this case, six.
What are some additional insights provided by the speaker regarding the relationships between different distributions?
-The speaker provides insights such as the discrete-to-continuous analogies (e.g., Exponential to Geometric), summation relationships (e.g., summing Geometric distributions results in a Negative Binomial), and how certain distributions can be derived from others through specific processes, like the Student's t-distribution from a specific process involving a Gamma distribution and a Normal distribution.
What is the purpose of the large graph mentioned at the end of the script?
-The large graph is a comprehensive visual representation that shows the relationships between various probability distributions. It is meant to be a starting point for those who wish to explore the connections between distributions in more depth.
Outlines
π§ Mastering Probability Distributions Through Analogies
The video introduces a unique approach to understanding probability distributions by using an analogy of memorizing a complex pattern within a minute. The presenter, DJ, suggests that recognizing patterns simplifies the learning process. The analogy is extended to probability distributions, where focusing on the stories that connect them, rather than individual definitions, is advocated. The video aims to show that by understanding the relationships between different distributions, one can grasp a vast amount of information with less effort. The summary of this paragraph emphasizes the importance of finding simple patterns to explain complexity in learning probability distributions.
π The Storytelling Method for Probability Distributions
This paragraph delves into the storytelling method by using the Bernoulli distribution as a starting point and explaining how different criteria for counting can lead to various distributions such as geometric, negative binomial, binomial, exponential, gamma, and Poisson. The presenter illustrates how these distributions are interconnected and how understanding their relationships can provide insights into their properties. The summary highlights the discrete-to-continuous analogies, the concept of summation relationships, and how these connections can help in memorizing and understanding the distributions without needing to memorize individual properties.
π Exploring Advanced Relationships and Insights
The final paragraph discusses advanced relationships between different probability distributions, providing examples that demystify certain distributions like the Student's t, Laplace, and Cauchy. It explains how these distributions can be derived from simpler ones through processes like sampling and transformation. The presenter also mentions a comprehensive graph that visually represents the relationships between various distributions, which is a valuable resource for further exploration. The summary emphasizes the value of these stories in providing additional properties and insights, helping to solidify the understanding of probability distributions.
Mindmap
Keywords
π‘Probability Distributions
π‘Analogy
π‘Bernoulli Distribution
π‘Geometric Distribution
π‘Negative Binomial Distribution
π‘Binomial Distribution
π‘Continuous Distribution
π‘Exponential Distribution
π‘Gamma Distribution
π‘Poisson Distribution
π‘Central Limit Theorem
π‘Student's t-distribution
π‘Laplace Distribution
π‘Cauchy Distribution
Highlights
The video introduces an analogy to explain the complexity of memorizing details versus understanding patterns.
The importance of finding simple patterns to explain complexity in learning about probability distributions.
The suggestion to focus on stories that relate probability distributions rather than their individual definitions.
An explanation of the Bernoulli distribution as the simplest random variable with two outcomes.
The geometric distribution is derived from the Bernoulli distribution by counting the number of trials until the first success.
The negative binomial distribution is introduced as a generalization of the geometric distribution with varying criteria for success.
The binomial distribution is explained as a result of grouping boxes and counting successes within those groups.
A method to create continuous versions of discrete distributions by dividing the probability of success by a large number.
The exponential distribution is presented as the continuous analog of the geometric distribution.
The gamma distribution is shown as a continuous generalization of the negative binomial distribution.
The Poisson distribution is explained as a continuous version of the binomial distribution, counting occurrences in fixed intervals.
The concept of discrete to continuous analogies is highlighted to aid in understanding complex distributions.
Summation relationships between distributions, such as the sum of geometric distributions leading to the negative binomial.
An introduction to the gamma distribution as a sum of exponential distributions.
The central limit theorem is mentioned as a reason distributions approach the normal distribution under certain conditions.
Additional stories are provided to demonstrate the range of relationships between different probability distributions.
The video concludes by emphasizing the value of learning stories that relate distributions for better understanding and memorization.
A call to action for viewers to like, subscribe, and support the content for continued learning about statistics and machine learning.
Transcripts
Browse More Related Video

Introduction to Probability Distributions
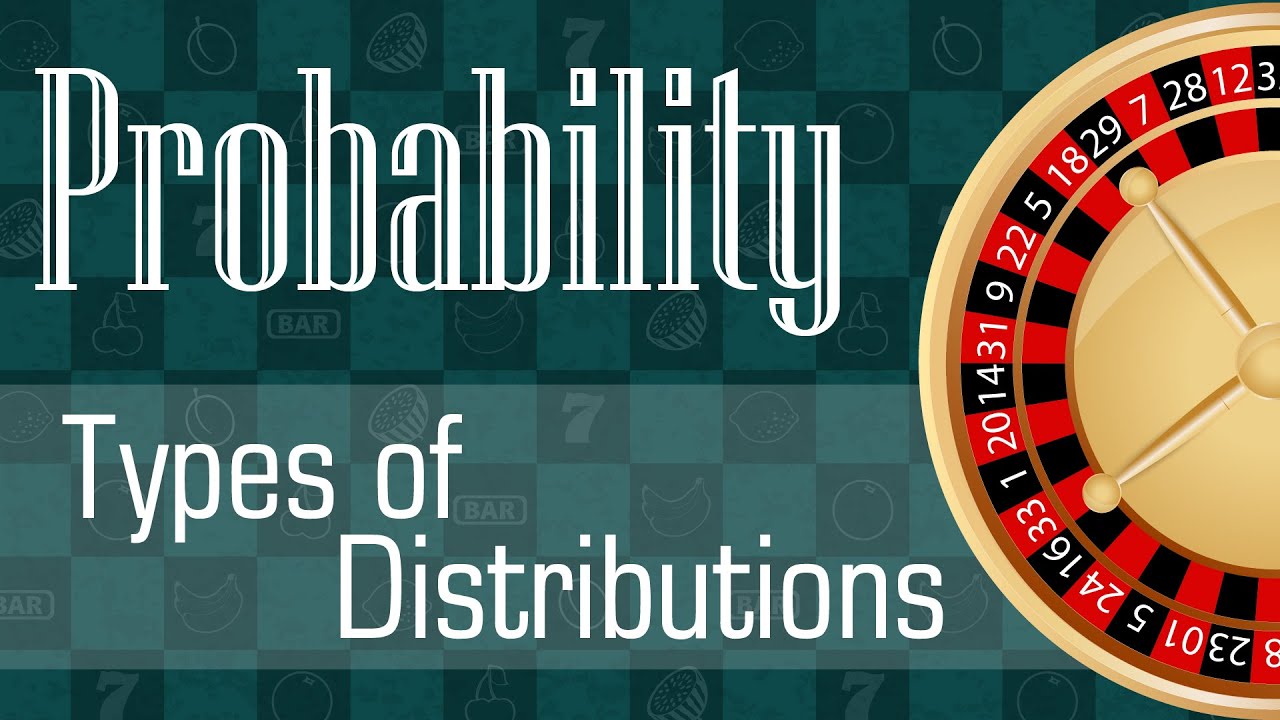
Probability: Types of Distributions
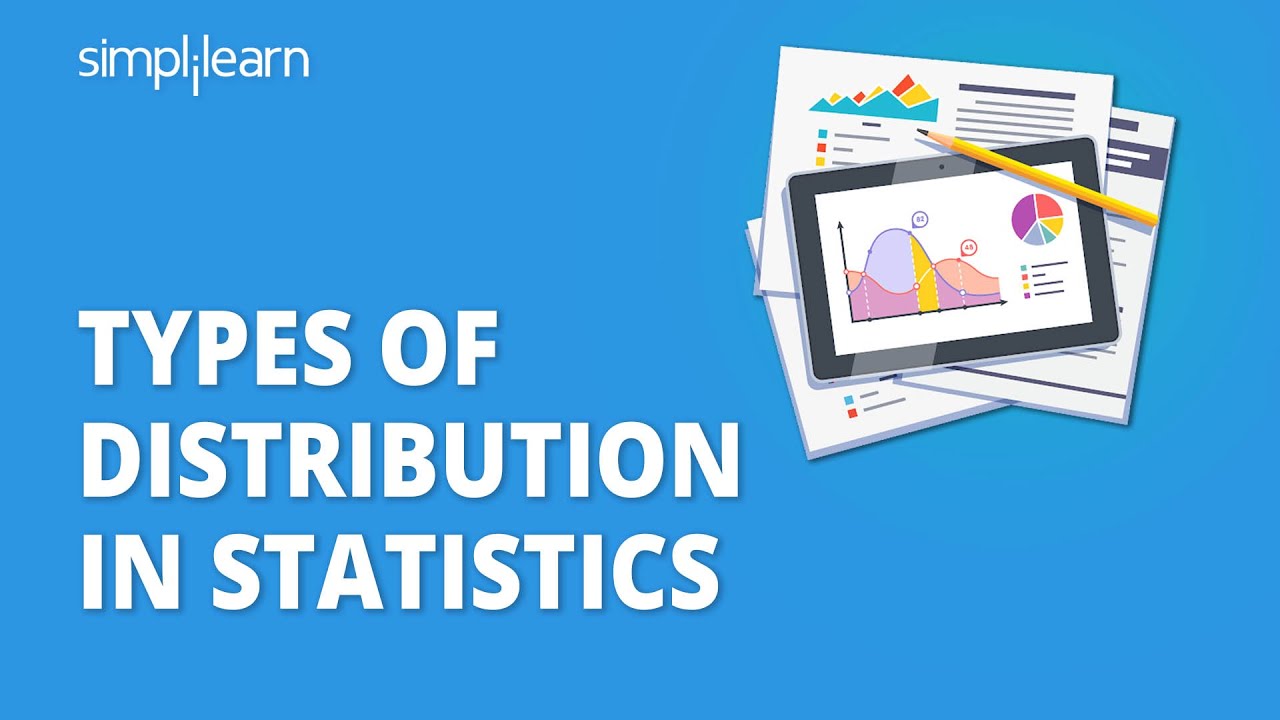
Types Of Distribution In Statistics | Probability Distribution Explained | Statistics | Simplilearn
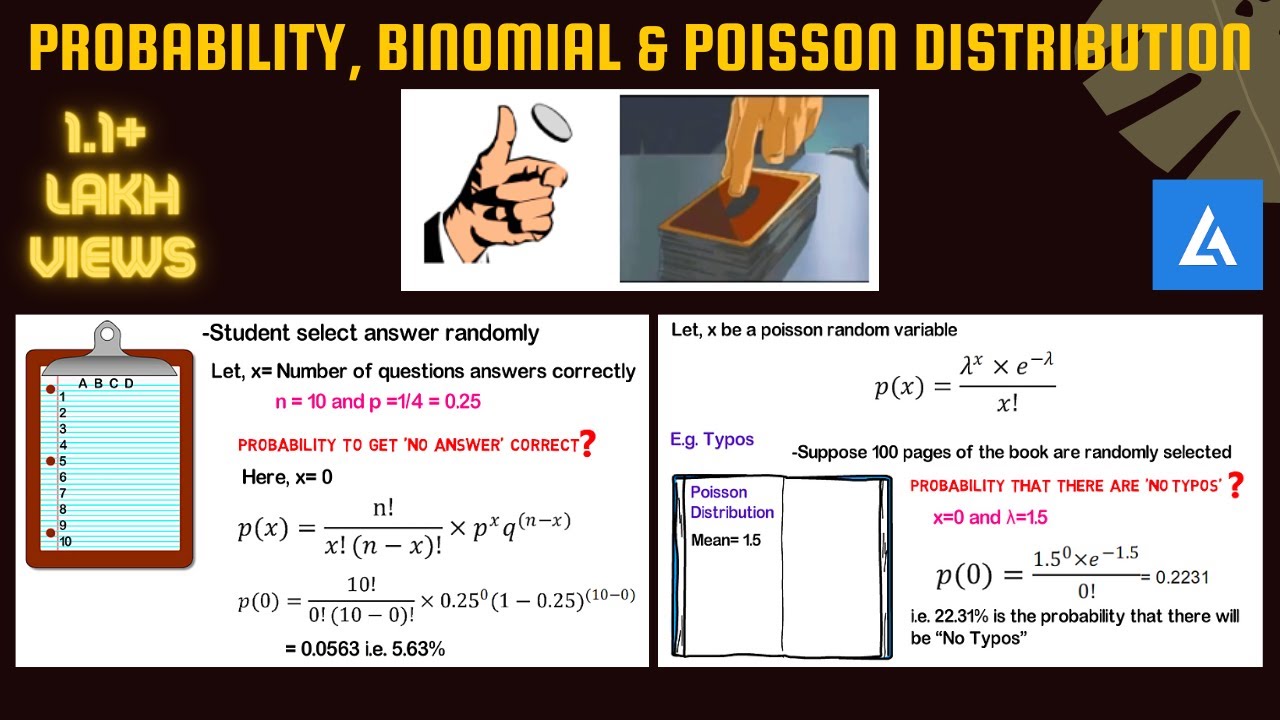
Basics of Probability, Binomial and Poisson Distribution
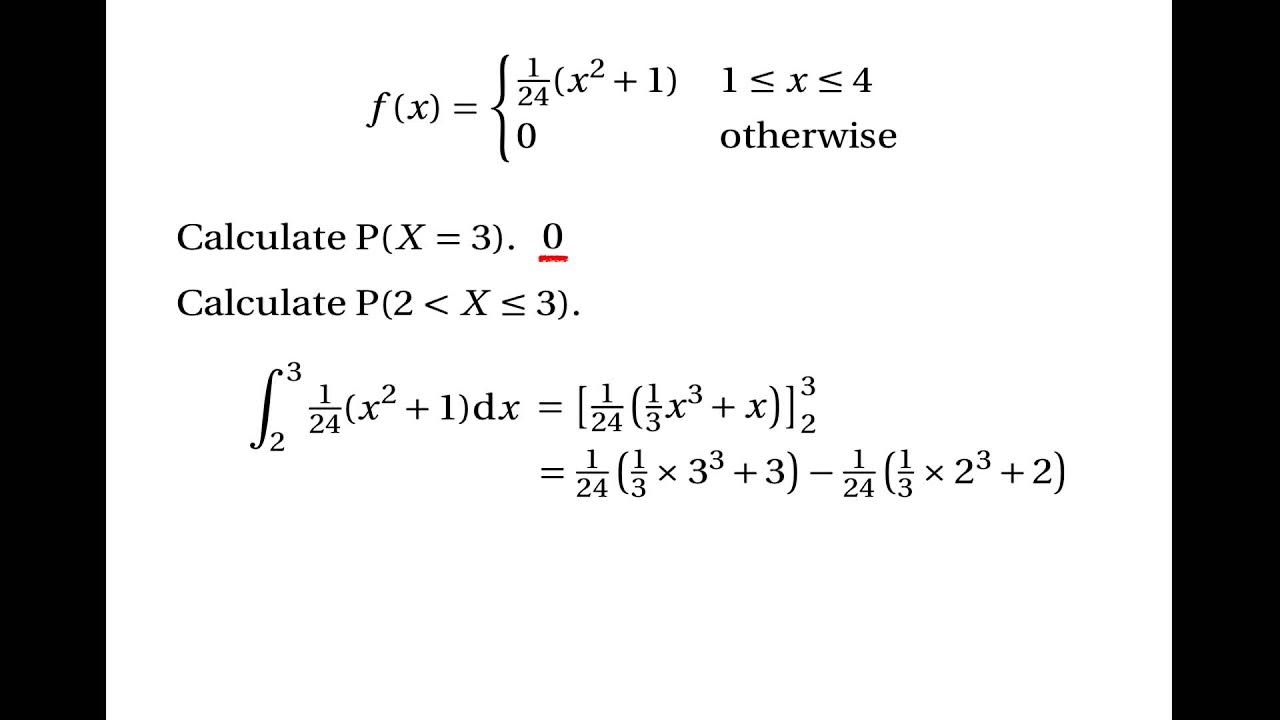
Continuous Random Variables: Probability Density Functions
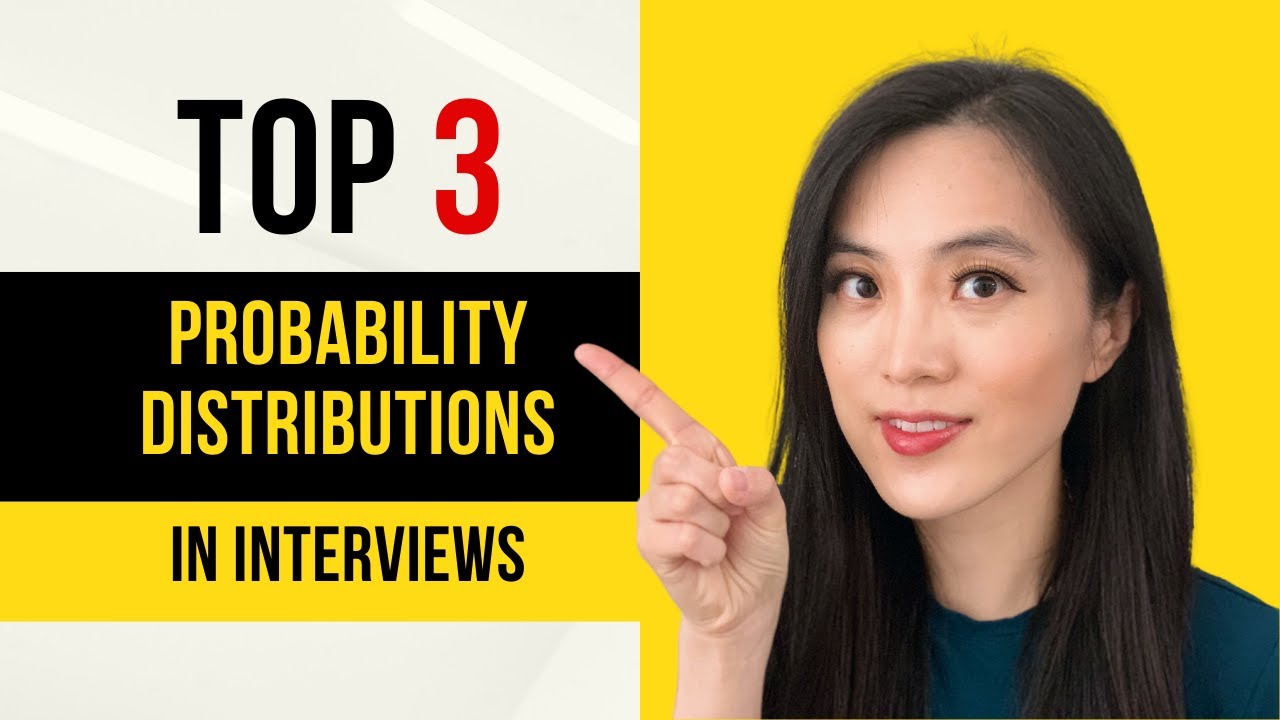
Probability Distributions Made Easy: Top 3 to Know for Data Science Interviews
5.0 / 5 (0 votes)
Thanks for rating: