Basics of Probability, Binomial and Poisson Distribution
TLDRThis video tutorial introduces the fundamentals of probability and statistics, focusing on probability distributions essential for MSA. It explains concepts like events, experiments, sample space, and probability calculation. The video delves into discrete distributions, particularly Binomial and Poisson, detailing their formulas, conditions, and applications. It also touches on the normal approximation for both distributions, illustrating how they can be approximated under certain conditions, providing practical examples and using Excel functions for probability calculation.
Takeaways
- π The importance of understanding probability distributions and their types in MSA (Managerial Statistics and Analytics).
- π Fundamentals of probability include events, experiments, sample space, and the calculation of probability.
- π² An event is any possible outcome of an experiment, such as getting a head or tail in a coin toss.
- π§© The sample space is the set of all possible outcomes of an experiment, like the outcomes of rolling a die.
- π’ Probability is the ratio of favorable outcomes to the total possible outcomes.
- π« Mutually exclusive events are those where only one can occur at a time, like head or tail in a coin toss.
- π A collectively exhaustive list includes every possible outcome of an experiment, ensuring no outcomes are missed.
- π Independent events are those where the outcome of one does not affect the outcome of another, like consecutive coin tosses.
- π The script covers five main types of distributions: Binomial, Poisson, Exponential, Weibull, and Normal.
- π Binomial and Poisson distributions are for discrete variables, while Exponential, Weibull, and Normal are for continuous variables.
- π Binomial distribution is characterized by two possible outcomes per trial, with a fixed probability of success and independence between trials.
- π The Poisson distribution models the number of events occurring in a fixed interval of time or space, with a given average rate.
- π The normal distribution can be used as an approximation for the binomial distribution when the sample size is large enough.
- π Differences between Binomial and Poisson distributions include the number of possible outcomes, the nature of the events, and the relationship between mean and variance.
Q & A
What is an event in the context of probability?
-An event is one or more possible outcomes of doing something, such as getting a tail when tossing a coin.
What is an experiment in probability?
-An experiment is an activity that produces an event, like tossing a coin or drawing a card from a deck.
What is the sample space in an experiment?
-The sample space is the set of all possible outcomes of an experiment, such as {head, tail} in a coin toss.
How is probability defined in the context of an event?
-Probability is the chance of an event occurring, calculated as the ratio of favorable chances to the total possible events.
What is a mutually exclusive event?
-Mutually exclusive events are those where only one of them can occur at a time, like getting a head or a tail in a coin toss.
What is a collectively exhaustive list in probability?
-A collectively exhaustive list includes every possible outcome of an experiment, ensuring no outcomes are left out.
What does it mean for two events to be independent?
-Two events are independent if the outcome of one does not influence the outcome of the other, such as the result of two separate coin tosses.
What are the main types of distributions discussed in the script?
-The main types of distributions discussed are Binomial, Poisson, Exponential, Weibull, and Normal Distribution.
What is the difference between discrete and continuous variables in terms of distributions?
-Discrete variables can take a countable number of values, while continuous variables can take any value within an interval.
What are the conditions for applying the Binomial distribution?
-The conditions include having only two outcomes per trial, a fixed probability of outcomes over time, and the trials being statistically independent.
How can Excel be used to find probabilities related to the Binomial distribution?
-Excel can be used with the BINOMDIST function, where you input the number of successes, number of trials, success probability, and cumulative argument.
What is the Normal Approximation to the Binomial distribution?
-The Normal Approximation is used when np and n(1-p) are both greater than 5, making the binomial distribution closely resemble a normal distribution.
What is the difference between the Binomial and Poisson distributions in terms of outcomes?
-The Binomial distribution has two possible outcomes (success or failure), while the Poisson distribution can have an unlimited number of outcomes.
How does the Poisson distribution model the occurrence of events?
-The Poisson distribution models the count of independent events occurring randomly within a given period of time, such as the number of patients arriving at a doctor's office.
How can Excel be used to find probabilities related to the Poisson distribution?
-Excel can be used with the POISSON function, where you input the number of occurrences, the mean, and the cumulative argument.
What is the relationship between the Normal Approximation and the Poisson distribution?
-When the number of observations is large (n > 20), the Poisson distribution can be approximated well by the normal distribution.
Outlines
π Introduction to Probability and Distributions
This paragraph introduces the fundamental concepts of probability and statistics, essential for understanding MSA (Management Science and Applied Statistics). It defines an event, an experiment, and the sample space, explaining how probability is calculated as the ratio of favorable outcomes to total possible outcomes. The paragraph also covers mutually exclusive events, collectively exhaustive lists, and independent events. It introduces five key distributions: Binomial, Poisson, Exponential, Weibull, and Normal, with a focus on Binomial and Poisson for discrete variables and a brief mention of the Normal distribution's previous detailed explanation.
π Binomial and Poisson Distributions Explained
This paragraph delves into the specifics of the Binomial and Poisson distributions, both of which are used for discrete data. It explains the Binomial distribution, which applies to situations with two possible outcomes, such as pass/fail, and provides the formula for calculating the probability of success in a series of trials. The paragraph also discusses the conditions for applying the Binomial distribution and gives an example involving a student guessing answers on a multiple-choice exam. It then explains the Poisson distribution, which is used for modeling the number of events occurring within a fixed interval of time or space, and provides an example of typographical errors in a textbook. The paragraph concludes with the use of Excel functions BINOMDIST and POISSON to calculate probabilities related to these distributions.
π Normal Approximations for Binomial and Poisson Distributions
The final paragraph discusses the normal approximation for both the Binomial and Poisson distributions, which is applicable when the sample size is large enough. It explains that as the number of trials (n) increases, the Binomial distribution approaches a Normal distribution, especially when both np and n(1-p) are greater than 5. The paragraph also touches on the Poisson distribution's approximation to the Binomial when the number of observations is large, and provides the conditions under which the normal distribution is a suitable substitute. The differences between the Binomial and Poisson distributions are highlighted, such as the number of possible outcomes, the nature of the events, and the relationship between mean and variance.
Mindmap
Keywords
π‘Probability Distribution
π‘Sample Space
π‘Mutually Exclusive Events
π‘Collectively Exhaustive List
π‘Independent Events
π‘Binomial Distribution
π‘Poisson Distribution
π‘Normal Distribution
π‘Continuous Variable
π‘Normal Approximation
π‘Excel Functions
Highlights
Introduction to the fundamentals of probability and its importance in MSA (Management Science and Applied Statistics).
Explanation of the concept of an event as one or more possible outcomes of an activity.
Definition of an experiment as an activity that produces an event, with examples provided.
Clarification of the sample space as the set of all possible outcomes of an experiment.
Discussion on the probability of an event being the ratio of favorable chances to total possible events.
Illustration of calculating the probability of getting a head when tossing a coin.
Description of mutually exclusive events where only one event can occur at a time.
Explanation of a collectively exhaustive list that includes every possible outcome of an experiment.
Concept of independent events where the outcome of one does not influence another.
Introduction to five main types of distributions: Binomial, Poisson, Exponential, Weibull, and Normal.
Differentiation between discrete and continuous variables in the context of statistical distributions.
Detailed explanation of the Binomial distribution, its parameters, and conditions for its application.
Formulas for calculating the mean and standard deviation of a binomial distribution.
Use of Excel's BINOMDIST function to find probabilities related to binomial distribution.
Conditions under which the normal distribution can be used as an approximation for the binomial distribution.
Introduction to the Poisson distribution, its characteristics, and applications.
Formula for calculating the probability in a Poisson distribution and its use in Excel.
Conditions when the Poisson distribution can be approximated by the normal distribution.
Comparison between Binomial and Poisson distributions highlighting their differences and specific use cases.
Conclusion summarizing the key points about distributions for discrete variables.
Transcripts
Browse More Related Video
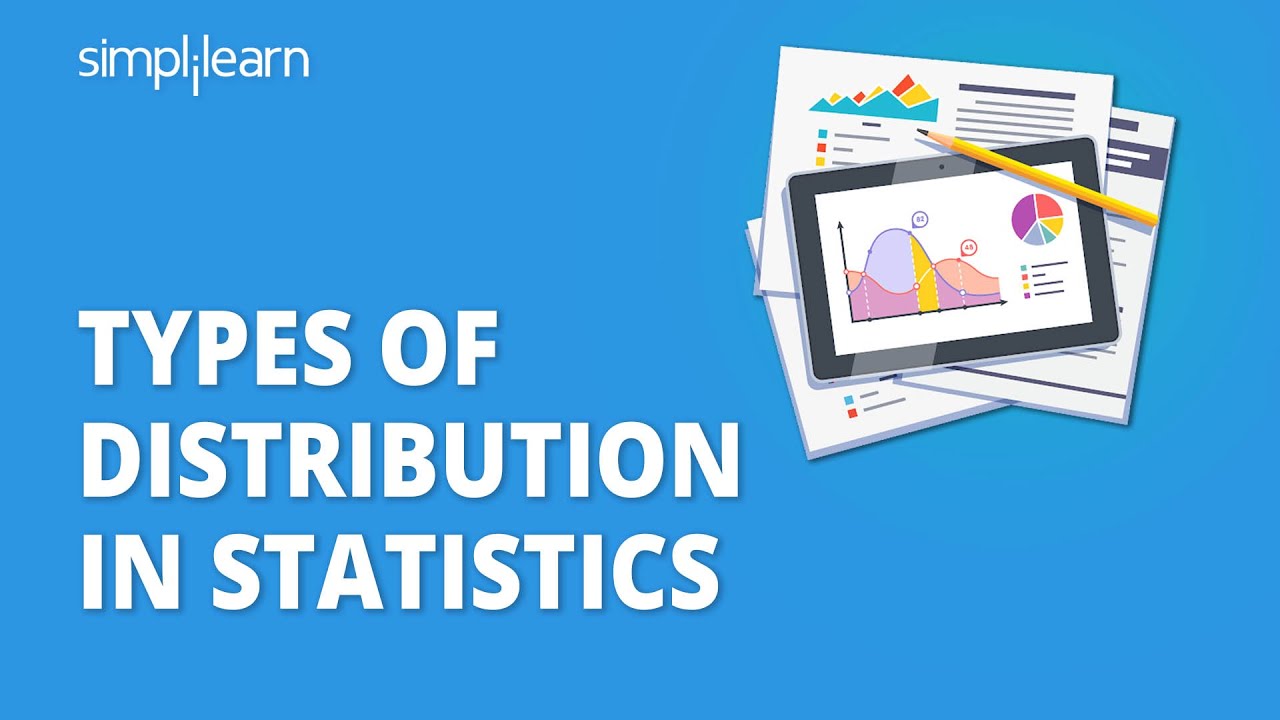
Types Of Distribution In Statistics | Probability Distribution Explained | Statistics | Simplilearn
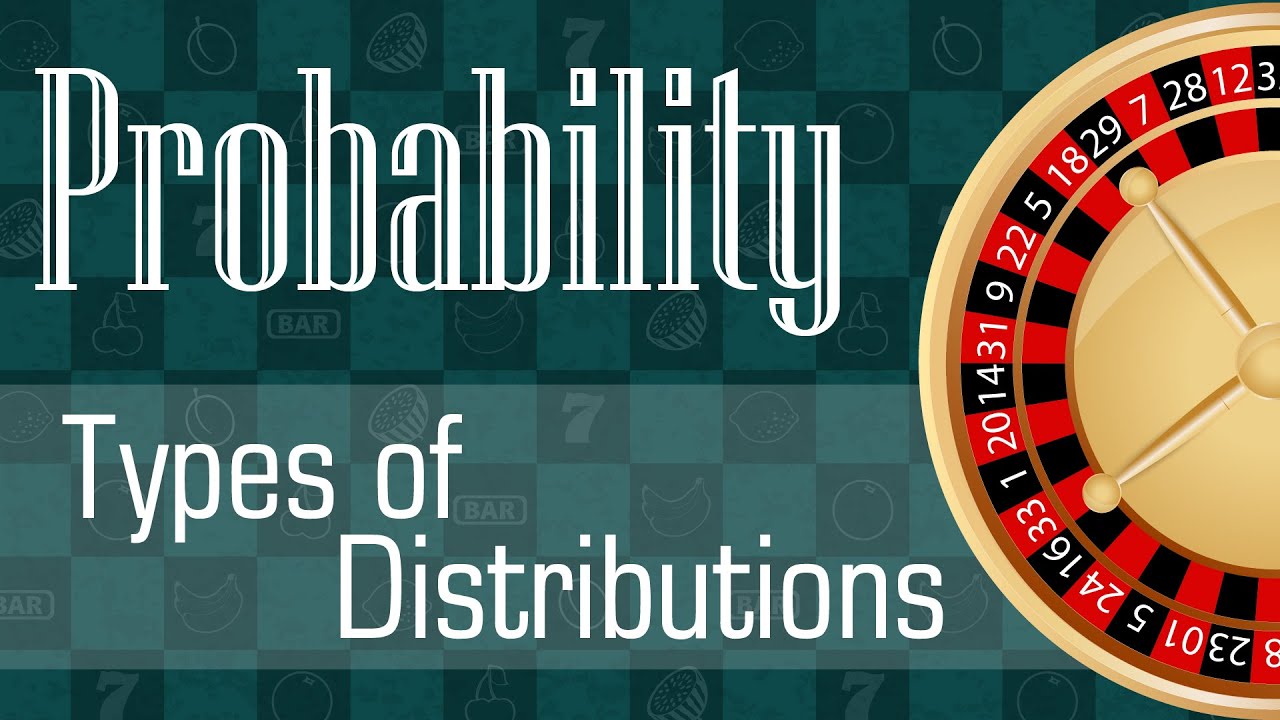
Probability: Types of Distributions

Introduction to Probability Distributions

Ultimate Probability Review for AP Statistics to Score a 5
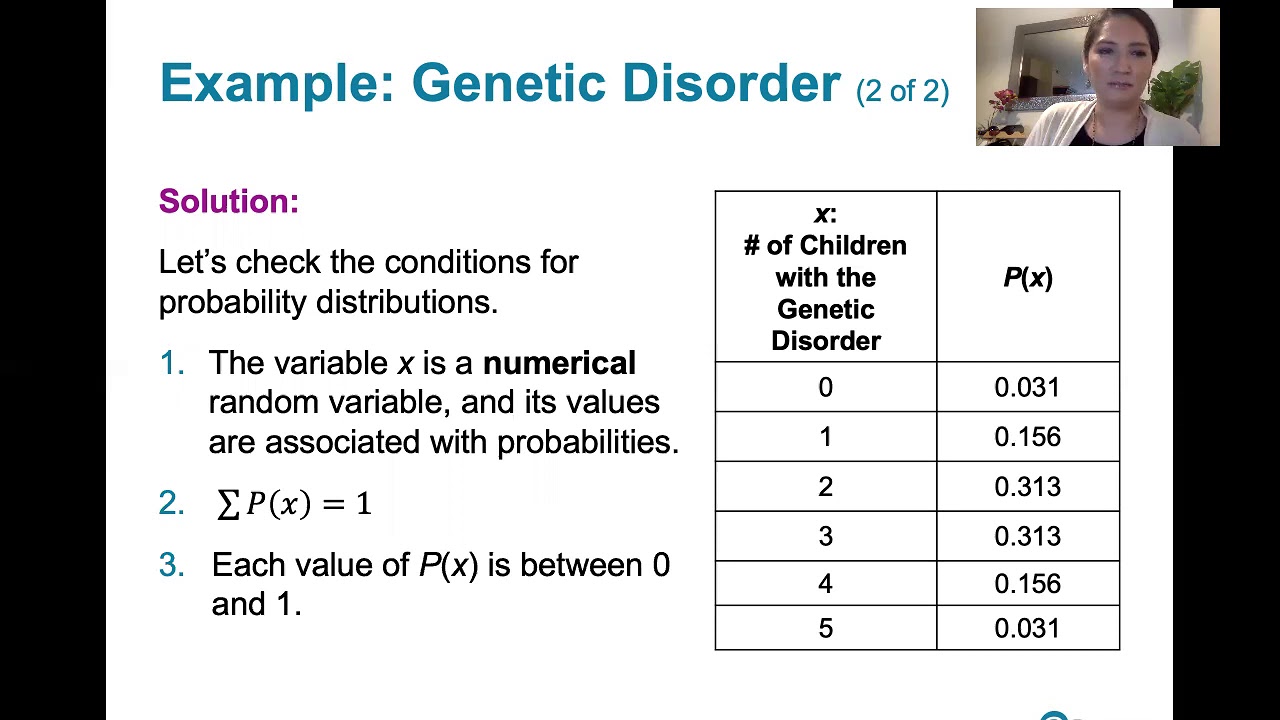
5.1.2 Discrete Probability Distributions - Probability Distributions and Probability Histograms
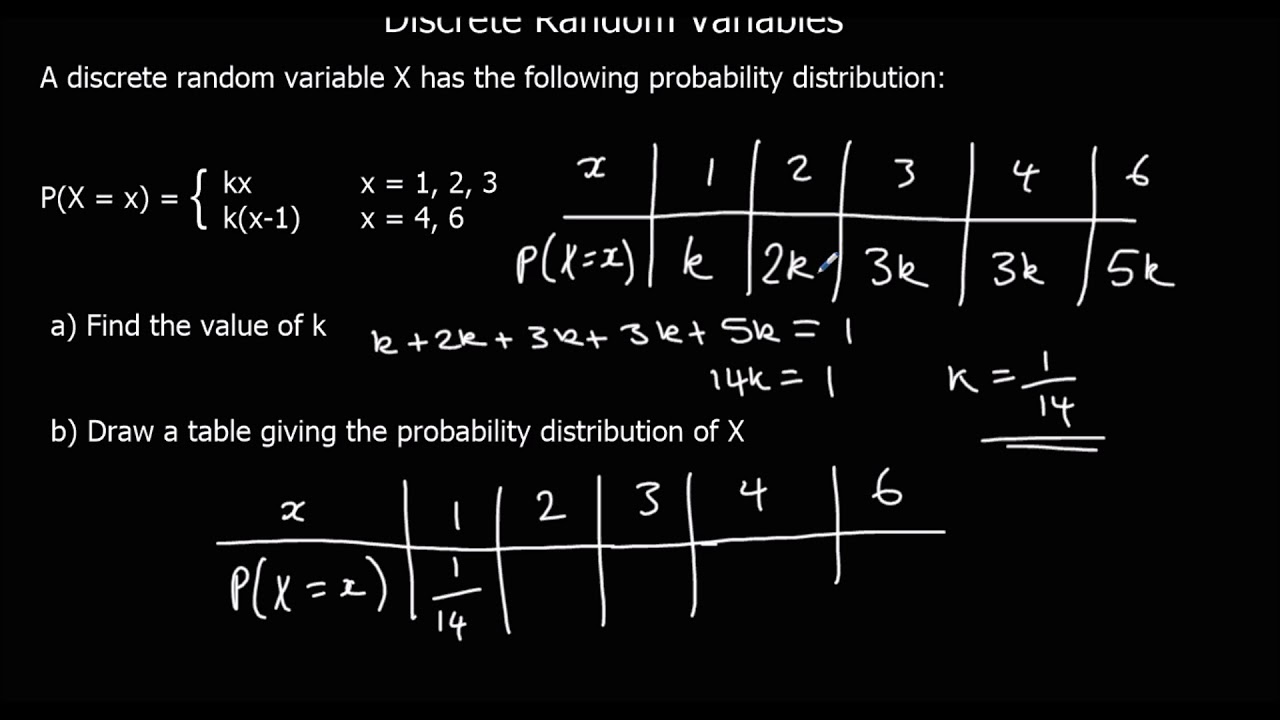
Discrete Random Variables
5.0 / 5 (0 votes)
Thanks for rating: