Ace Statistics Interviews: A Data-driven Approach For Data Scientists
TLDRThis video offers a data-driven approach to preparing for common statistics questions in data science job interviews. Host Emma from Amazon.com identifies top concepts like p-value, linear regression, t-tests, correlation coefficient, and types of errors. She explains the p-value's significance in hypothesis testing and provides a real-world example using a productivity app. The video also covers linear regression assumptions, t-test conditions, and the difference between covariance and correlation coefficient. A free cheat sheet is available to help viewers tackle over 40% of potential interview questions, boosting confidence and interview success.
Takeaways
- π Statistics can be daunting, especially in data science job interviews, where unexpected questions may arise.
- πΌ Emma from Amazon.com offers proactive tips and strategies for interviews, including preparing for statistics questions.
- π Emma analyzed over 300 statistics interview questions from 50+ companies, identifying common concepts and patterns.
- π The most common statistics questions focus on fundamental concepts such as p-value, linear regression, t-test, correlation coefficient, and types of errors.
- π― P-value is the most important concept, appearing in over 10% of interview questions, and is crucial for hypothesis testing.
- π P-value measures the likelihood of observing results as extreme as the sample, assuming the null hypothesis is true, with a common threshold of 0.05.
- π Linear regression assumptions can be remembered by the acronym LINE, standing for Linearity, Independence, Normality, and Equal variance of residuals.
- π§ T-tests are used to determine if two groups have different means and share most assumptions with linear regression, except for the linearity aspect.
- π The correlation coefficient indicates the strength of the linear relationship between two variables, while covariance focuses on the direction of the relationship.
- π« Type 1 error occurs when incorrectly rejecting a true null hypothesis, while Type 2 error happens when failing to reject a false null hypothesis.
- π Emma provides a free cheat sheet covering frequently asked statistics interview questions to help prepare for over 40% of potential interview questions.
Q & A
What is the main purpose of the video?
-The main purpose of the video is to explore the top statistics questions that often come up in data science job interviews and to present them in an easy-to-understand way, even for those who haven't studied statistics recently.
Who is the speaker in the video?
-The speaker in the video is Emma from Amazon.com, who aims to help viewers land their dream data scientist job by providing tips and strategies for interviews and offer negotiations.
How many statistics interview questions did Emma analyze from different companies?
-Emma analyzed over 300 statistics interview questions from over 50 different companies.
What are the top five statistics concepts that frequently come up in interviews according to the video?
-The top five statistics concepts that frequently come up in interviews are p-value, linear regression, t-test, correlation coefficient, and types of errors.
What is the significance of the p-value in data science interviews?
-The p-value is significant in data science interviews as it is the most commonly asked question, appearing in over 10 percent of the questions, with almost half of the companies asking about it.
How is the p-value defined and what does it measure?
-The p-value is a tool in hypothesis testing that measures the likelihood of obtaining results as extreme as the ones observed in a sample, assuming the null hypothesis is true.
What is the common cut-off value for the p-value and what does it imply?
-The common cut-off value for the p-value is 0.05. If the p-value is less than 0.05, it implies strong evidence against the null hypothesis, allowing for its rejection. If it's greater than 0.05, it indicates weak evidence and the null hypothesis cannot be rejected.
Can you provide an example of how to explain the p-value to a non-technical audience?
-An example given in the video involves a productivity app called Notion. By comparing the productivity of two groups, one using the app and the other not, the p-value can determine if the difference in productivity is statistically significant or due to chance.
What are the four key assumptions of linear regression and how can they be remembered?
-The four key assumptions of linear regression are linearity (L), independence (I), normality (N), and equal variance (E). They can be remembered using the acronym LINE.
What does the acronym 'LINE' stand for in the context of linear regression assumptions?
-The acronym 'LINE' stands for Linearity, Independence, Normality, and Equal variance, which are the four key assumptions to consider in linear regression.
How can you differentiate between covariance and correlation coefficient?
-Covariance focuses on the direction of the relationship between two variables, while the correlation coefficient measures the strength of the linear relationship. The correlation coefficient is unitless and ranges between -1 and 1, whereas covariance has units that are the product of the units of the two variables.
What are the two main types of errors in hypothesis testing?
-The two main types of errors in hypothesis testing are Type I error, which is a false positive (mistakenly concluding there is a difference when there isn't), and Type II error, which is a false negative (failing to detect a true difference).
How can you remember the difference between Type I and Type II errors?
-Type I error can be remembered as a false positive, which contains only one instance of the word 'false'. Type II error can be remembered as a false negative or a 'false false', which helps by repeating the word 'false'.
What resource does Emma offer to help viewers prepare for statistics interview questions?
-Emma offers a free cheat sheet that covers the most frequently asked statistics interview questions, which can be downloaded by clicking the link provided in the video description.
Outlines
π Mastering Statistics for Data Science Interviews
This paragraph introduces the video's focus on preparing for data science job interviews with a particular emphasis on statistics. The speaker, Emma from Amazon.com, shares her experience and insights gathered from analyzing over 300 interview questions from various companies. The goal is to make complex statistical concepts easy to understand and to highlight the top five most frequently asked questions: p-value, linear regression, t-test, correlation coefficient, and types of errors. The p-value is emphasized as the most important concept, with a structured approach to explaining it in interviews. The video promises to cover all this in under 15 minutes, aiming to boost viewers' confidence in tackling statistical questions in interviews.
π Understanding P-Values and Linear Regression Assumptions
The second paragraph delves into the concept of the p-value, explaining its role in hypothesis testing and how it helps to determine the significance of observed results. A structured approach to explaining p-values in interviews is provided, including its definition, the interpretation of different p-value thresholds, and its practical application in A/B testing. The paragraph also introduces the assumptions of linear regression, using the acronym 'LINE' to remember them: Linear relationship, Independence, Normality, and Equal variance of residuals. A free cheat sheet covering frequently asked statistics interview questions is offered to help viewers prepare more effectively for interviews.
π Exploring T-Tests, Correlation, and Hypothesis Testing Errors
This paragraph continues the discussion on statistical concepts important for data science interviews, starting with t-tests. It outlines the assumptions of t-tests using the acronym 'I and E' for Independence and Normality, and Equal variance, and explains the use of t-tests to determine if two groups have different means. The paragraph then contrasts covariance and correlation coefficient, highlighting the correlation coefficient's unitless nature and its range between -1 and 1, versus covariance which is unit-dependent and can vary. Lastly, it addresses the two main types of errors in hypothesis testing: Type I (false positive) and Type II (false negative) errors, providing examples and a mnemonic to help remember the concepts. Additional resources, such as dedicated videos on t-tests and hypothesis testing, are mentioned for further learning.
Mindmap
Keywords
π‘Statistics
π‘Data Science Job Interview
π‘P-value
π‘Hypothesis Testing
π‘Linear Regression
π‘Assumptions
π‘T-test
π‘Correlation Coefficient
π‘Covariance
π‘Type I and Type II Errors
π‘Confidence
Highlights
The video aims to prepare viewers for common statistics questions in data science job interviews.
Emma from Amazon.com provides tips and strategies for job interviews and offer negotiations.
A data-driven approach is used to analyze over 300 statistics interview questions from 50 companies.
Fundamental concepts like p-value, linear regression, t-test, correlation coefficient, and types of errors are frequently asked.
P-value is the most important concept, appearing in over 10% of interview questions.
The p-value measures the likelihood of observing results as extreme as the sample, assuming the null hypothesis is true.
A p-value less than 0.05 indicates strong evidence against the null hypothesis.
The concept of p-value is applied in A/B testing to determine significant differences between groups.
Simple examples, like a productivity app scenario, are used to explain p-value to non-technical audiences.
Linear regression assumptions are remembered using the acronym LINE.
The independence of residuals and normal distribution of residuals are key assumptions for linear regression.
Equal variance assumption ensures consistent spread of residuals across different values of X.
A cheat sheet covering frequently asked statistics interview questions is available for download.
T-tests are used to determine if two groups have different means and have specific assumptions similar to linear regression.
The correlation coefficient measures the strength and direction of the linear relationship between two variables.
Covariance and correlation coefficient are distinguished by their focus on relationship direction and strength.
Type 1 error occurs when concluding a difference where there isn't one, like falsely claiming a change in button color affects conversion rates.
Type 2 error is the failure to detect a real difference, such as not recognizing a button color change's impact on conversion rates.
A mnemonic device is provided to remember type 1 and type 2 errors as 'false positive' and 'false negative'.
Further resources include videos on hypothesis testing and a playlist dedicated to statistics interview questions.
The video encourages continuous learning and curiosity to boost confidence in data science interviews.
Transcripts
Browse More Related Video
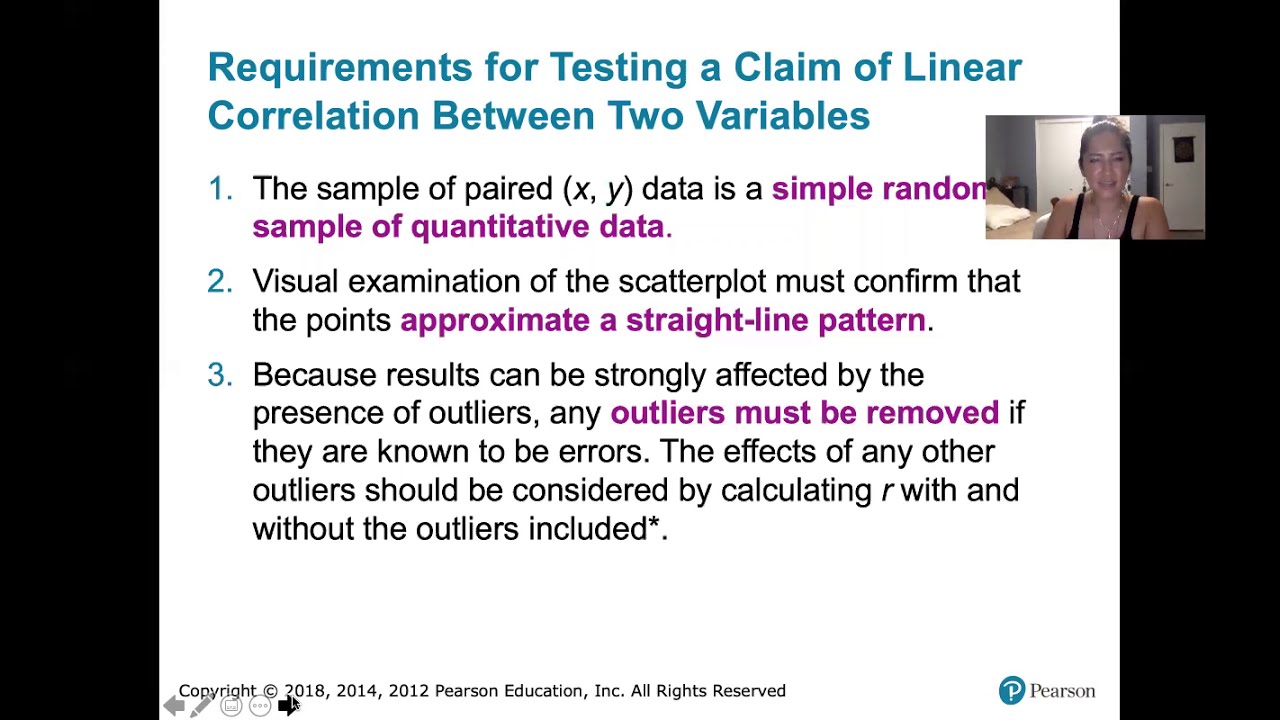
10.1.5 Correlation - Testing a Claim of Correlation Using the P-Value Method
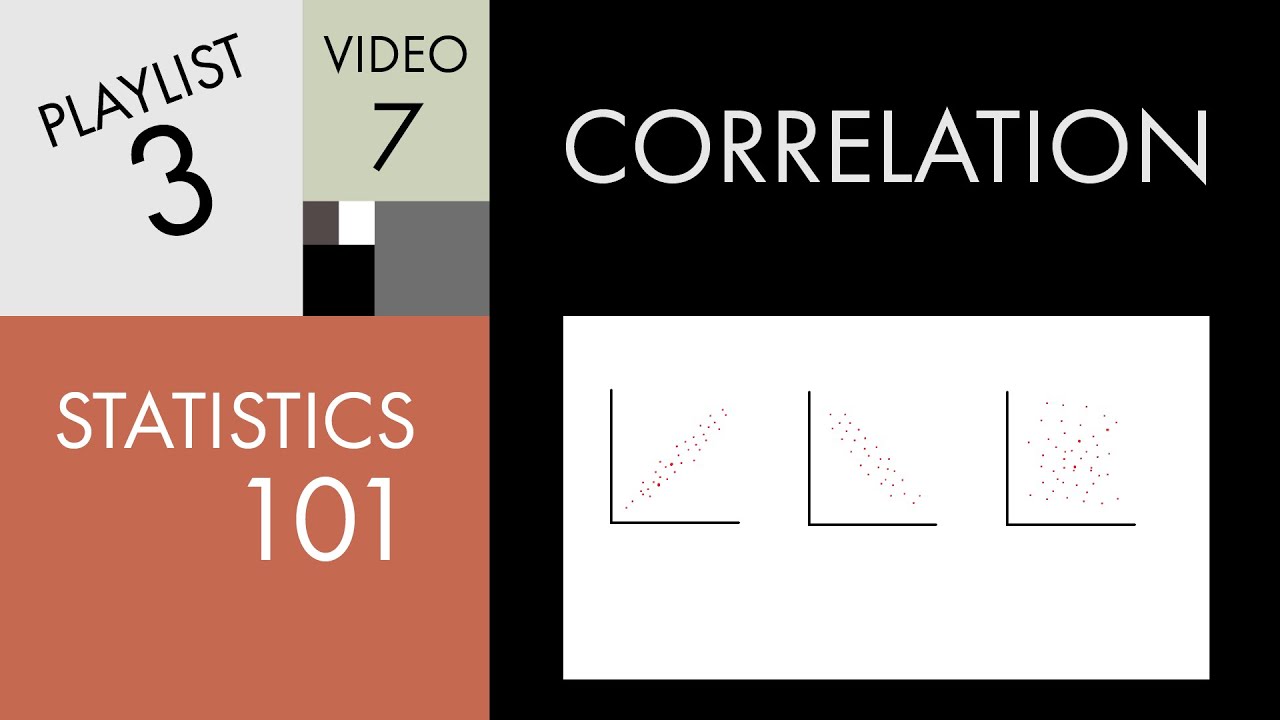
Statistics 101: Understanding Correlation
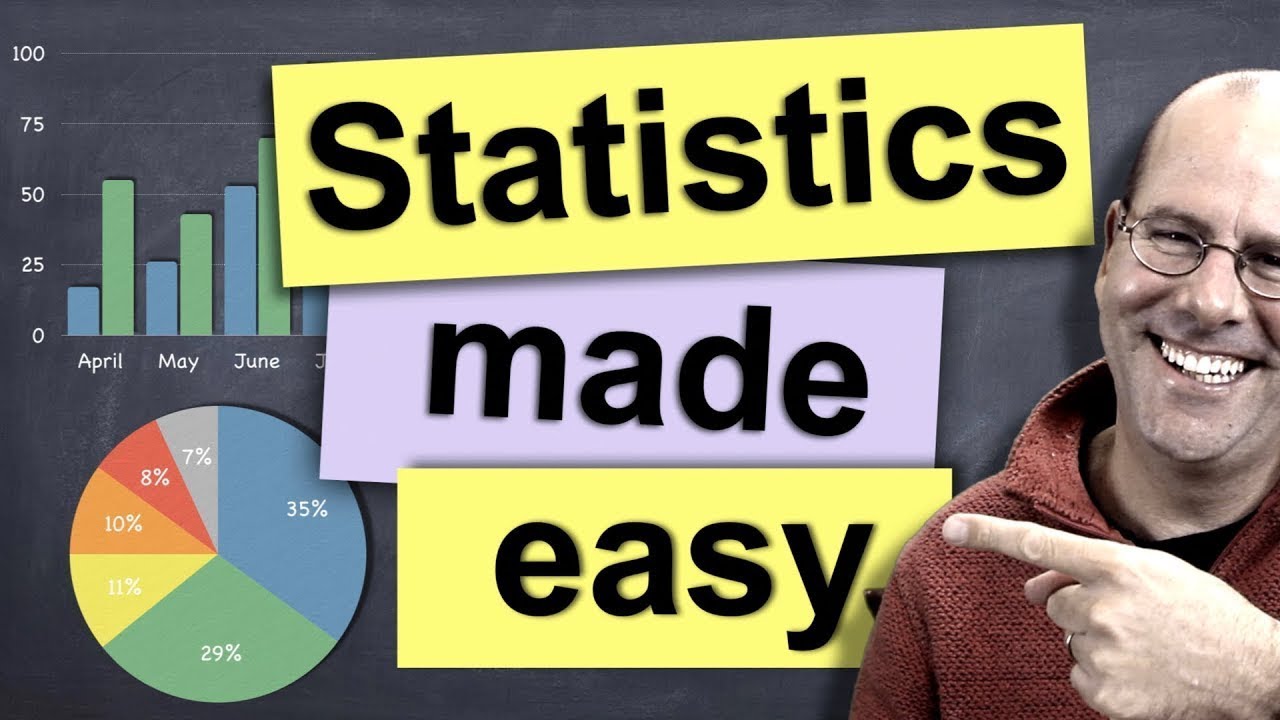
Statistics made easy ! ! ! Learn about the t-test, the chi square test, the p value and more
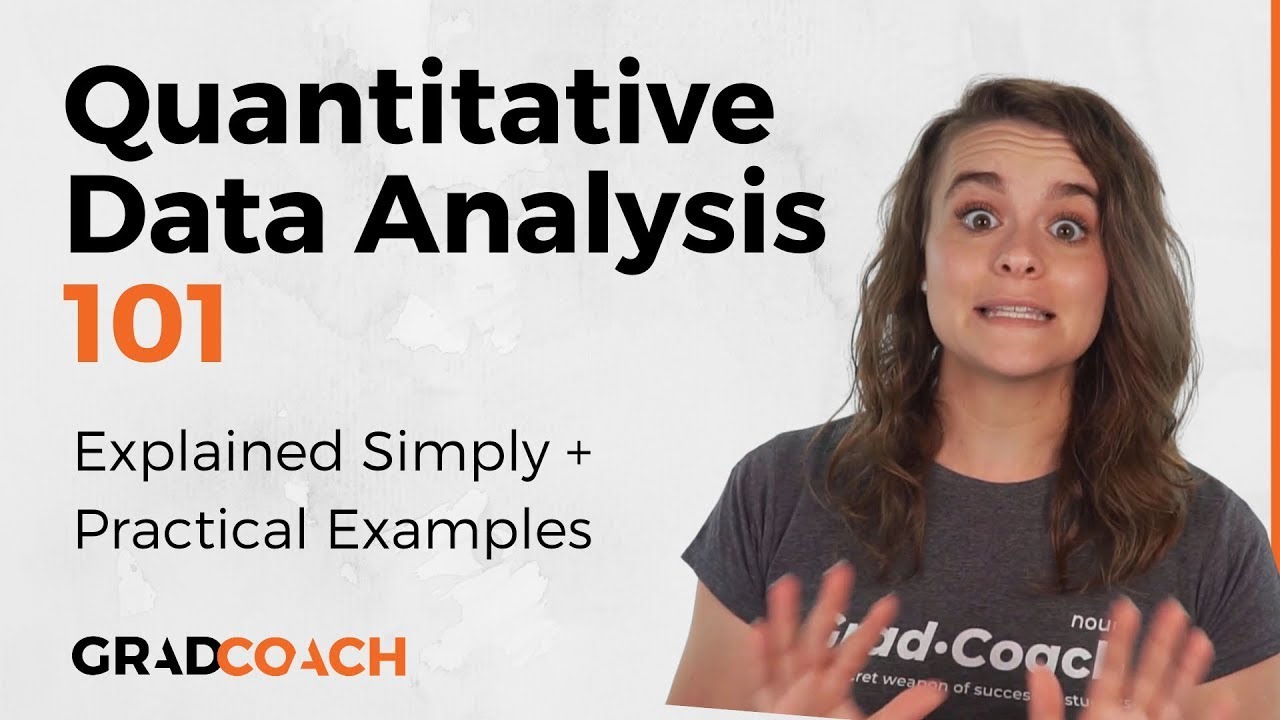
Quantitative Data Analysis 101 Tutorial: Descriptive vs Inferential Statistics (With Examples)
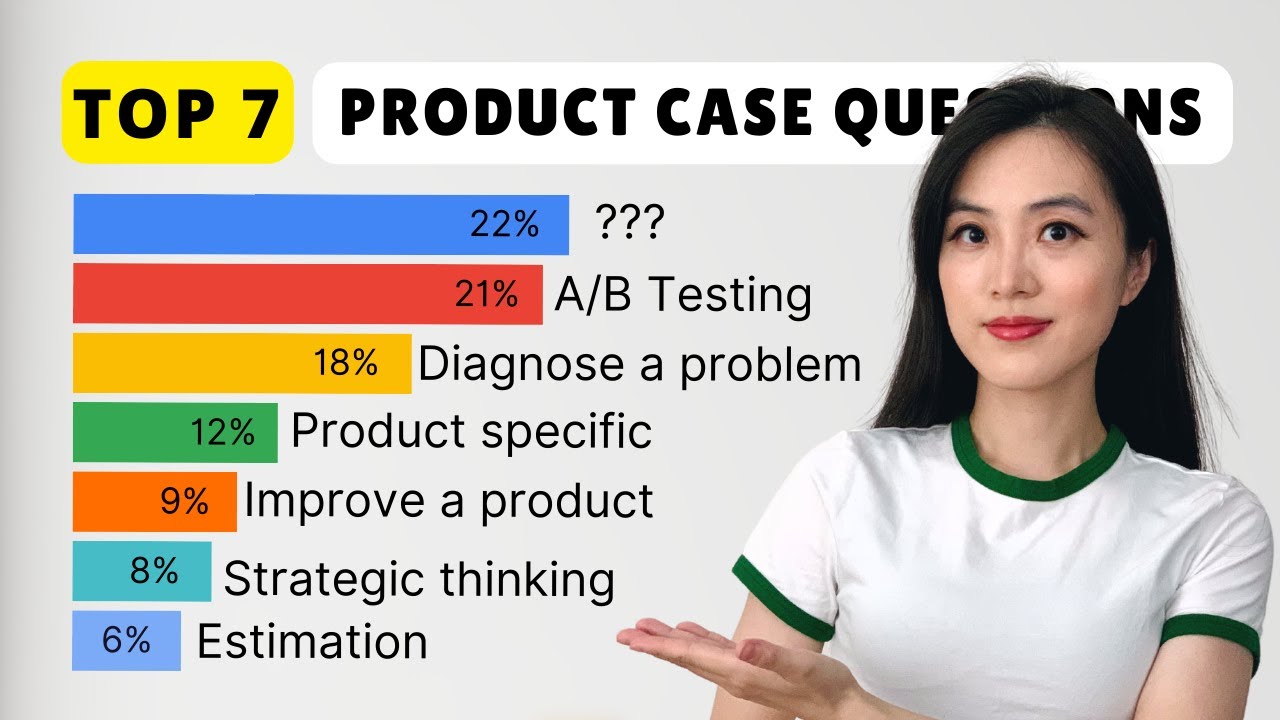
Ace Product/Business Case Interview Questions: A Data-driven Approach for Data Scientists
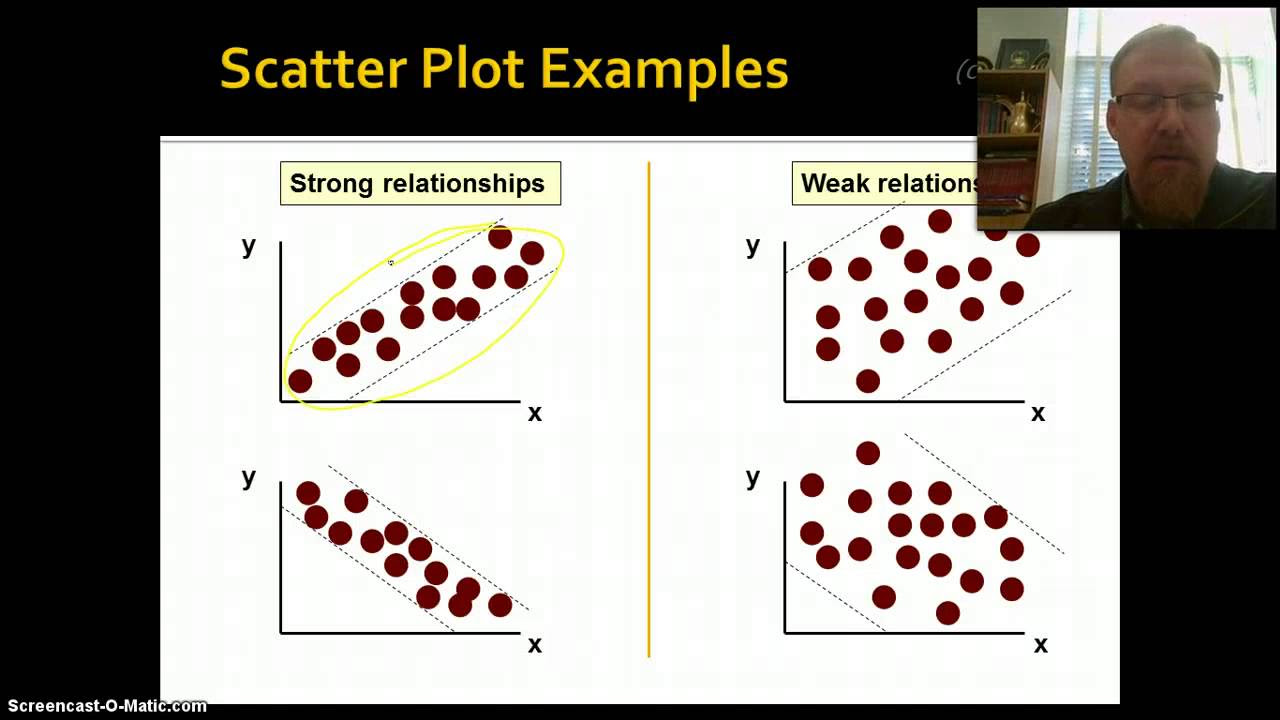
Introduction to Correlation & Regression, Part 1
5.0 / 5 (0 votes)
Thanks for rating: