Marco Bonzanini - Lies, damned lies, and statistics
TLDRThe speaker discusses the use, misuse, and abuse of statistics in everyday life, emphasizing the importance of critical thinking when interpreting statistical data. They debunk common misconceptions, such as the confusion between correlation and causation, and introduce concepts like lurking variables and the Simpson's paradox. The talk also addresses various biases, including sampling bias and survivorship bias, and the potential for misleading data visualization. The speaker cautions about the misuse of statistical significance and p-values, and the dangers of data dredging. The presentation concludes with a call to always question the context and motivations behind statistical findings, advocating for a more informed and discerning approach to data analysis.
Takeaways
- π **Understanding Statistics**: The speaker emphasizes the importance of comprehending statistics to be a well-informed citizen, not just for those with advanced degrees in math.
- π **Humor in Data**: The presentation starts with a humorous example about the population density of Vatican City and the concept of one Pope at a time.
- π **Correlation vs. Causation**: A key point made is the need to differentiate between correlation, which indicates a relationship, and causation, which implies a direct cause and effect.
- π **Lurking Variables**: The concept of lurking variables is introduced to explain how a third, unseen variable can influence the correlation between two observed variables.
- π **Simpson's Paradox**: The paradox is explained as a situation where data can be analyzed differently, leading to seemingly contradictory conclusions when grouped in various ways.
- π€ **Sampling Bias**: The audience is reminded of the potential for bias in sampling, which can lead to incorrect conclusions when a sample doesn't represent the larger population.
- π **Survivorship Bias**: The issue of survivorship bias is highlighted, where focusing only on 'winners' can lead to an incomplete understanding of a situation.
- π **Data Visualization**: The power of data visualization is discussed, showing how it can be used to communicate complex ideas simply and effectively.
- π **Manipulation in Visualization**: The potential for manipulation in data visualization is also covered, warning against graphs that may distort the truth by starting axes at unconventional points.
- βοΈ **Statistical Significance**: The distinction between statistical significance and practical importance is made clear, with the former not necessarily implying the latter.
- π£ **Data Dredging**: The practice of data dredging, or looking for patterns in data until something significant is found, is cautioned against due to the risk of false conclusions.
- π€ **P-Values and Data Fishing**: The concept of p-values is introduced, and the dangers of data fishing, where one might manipulate data to achieve a desired p-value, are discussed.
Q & A
What is the main focus of the talk?
-The main focus of the talk is the use, misuse, and abuse of statistics in everyday life. It aims to educate people on how not to be misled by statistical data and to be more discerning when interpreting statistical information.
What is the correlation mentioned in the talk?
-Correlation, as discussed in the talk, refers to a relationship or connection between two variables or events. It can be measured in terms of strength and is often visualized through a linear correlation, where one variable increases or decreases as the other does.
What is the difference between correlation and causation?
-Correlation indicates a relationship between two variables, but it does not imply that one variable causes the other to change. Causation implies a direct cause-and-effect relationship, which is a stronger claim than correlation.
What is a lurking variable?
-A lurking variable is a factor that is not immediately visible but influences the relationship between two other variables. It can help explain why seemingly correlated variables appear to have a connection that might not be directly causal.
Why is the Simpsons paradox mentioned in the context of data analysis?
-The Simpsons paradox is mentioned to illustrate how aggregated data can sometimes be misleading. It occurs when data is grouped differently, and the overall trend appears to reverse when looking at individual groups, highlighting the importance of considering data distribution.
What is sampling bias?
-Sampling bias is a systematic error that occurs when a subset of individuals is selected for a study in a way that is not representative of the larger population. This can lead to incorrect conclusions being drawn from the study.
What is survivorship bias?
-Survivorship bias is a type of sampling bias where only the 'winners' or survivors are considered, and the 'losers' or those who did not survive are ignored. This can lead to an overestimation of success rates and outcomes.
How can data visualization be misleading?
-Data visualization can be misleading if it is manipulated, such as by starting the axis at a value other than zero to exaggerate differences, or by omitting important context, which can lead to a misinterpretation of the data.
What is statistical significance?
-Statistical significance refers to the likelihood that the results of a study are not due to chance. A common threshold for statistical significance is a p-value of less than 0.05, indicating that there is a less than 5% chance that the results occurred by random chance.
What is data dredging?
-Data dredging, also known as data fishing, involves searching through data for patterns or relationships without a prior hypothesis. This can lead to false discoveries because the same data is used to both find and confirm the patterns.
Why is it important to question the context and funding of a study?
-Questioning the context and funding of a study is important because it helps to identify potential biases and vested interests that could influence the results. Understanding the bigger picture can help discern whether the presented data is being used accurately and ethically.
Outlines
π Understanding Statistics and Their Misuse
The speaker begins by humorously addressing the three types of lies: lies, damned lies, and statistics. They clarify a statistical fact about the population density of Popes in Vatican City, using it as a segue into a broader discussion about the everyday use and potential misinterpretation of statistics. The talk aims to educate on how to be critical consumers of statistical information without requiring advanced mathematical knowledge. The speaker also gauges the audience's familiarity with Python, highlighting its positive correlation with well-being.
π Correlation vs. Causation and Lurking Variables
The speaker delves into the concept of correlation, emphasizing that it does not imply causation. They explain the difference between positive and negative linear correlation through examples, such as ice cream sales and drowning incidents. The importance of considering lurking variables that might explain the observed correlation is discussed, using the example of temperature affecting both ice cream sales and swimming activities.
π Data Visualization and Simpson's Paradox
The speaker discusses the power of data visualization in conveying complex concepts and its role in data analytics. They introduce the Simpson's paradox with an example from the University of California's admission rates, highlighting how aggregated data can sometimes be misleading. The paradox is explained by showing how men and women tend to apply to different departments with varying admission rates, which can skew the interpretation of the data when not analyzed at a granular level.
π€ Sampling Bias and Its Impact on Data Interpretation
The speaker addresses the issue of sampling bias, using the historical example of the 'Dewey Defeats Truman' headline based on a biased survey. They also mention survivorship bias, a common pitfall when focusing only on successful cases while ignoring failures. The speaker underscores the importance of representative sampling to avoid skewed results and discusses how data visualization can be used to either clarify or obfuscate the truth, depending on the intent.
π Manipulation of Data Visualization and Statistical Significance
The speaker critiques the manipulation of data visualization for misleading purposes, using examples from politics and media. They discuss how the choice of axis scales can alter perceptions of data. The concept of statistical significance and its misinterpretation in everyday language is also explored, with a clarification that statistical significance does not equate to importance or usefulness. The misuse of p-values and the issue of data dredging are highlighted as common statistical pitfalls.
π§ Questioning Data and Avoiding Bias
The speaker concludes by emphasizing the importance of questioning the data presented to us, especially when it aligns with a particular agenda. They caution against the blind acceptance of media headlines and the need for a deeper understanding of the context and methodology behind statistical studies. The speaker encourages critical thinking and a healthy skepticism towards data, advocating for a more informed approach to interpreting statistical information.
Mindmap
Keywords
π‘Statistics
π‘Correlation
π‘Lurking Variable
π‘Simpson's Paradox
π‘Sampling Bias
π‘Survivorship Bias
π‘Data Visualization
π‘Statistical Significance
π‘P-values
π‘Data Dredging
π‘Bias in Media Reporting
Highlights
The speaker humorously introduces the topic of statistics by discussing the 'lies, damned lies, and statistics' adage.
The importance of understanding statistics in everyday life is emphasized, even without advanced degrees in math.
The concept of correlation is introduced, explaining its informal definition and its role in statistical analysis.
The difference between correlation and causation is clarified using the example of ice cream sales and drowning incidents.
The idea of a lurking variable, which can affect the perceived correlation between two variables, is discussed.
The speaker presents examples of spurious correlations, such as the number of movies with Nicolas Cage and drowning incidents.
The Simpsons paradox is introduced, demonstrating how data can be misinterpreted when analyzed in different ways.
Sampling bias is explained using the example of a survey that led to the incorrect headline 'Dewey Defeats Truman'.
The potential for bias in data visualization is highlighted, showing how charts can be manipulated to present a misleading narrative.
The concept of statistical significance is discussed, emphasizing that it does not equate to the importance of results.
P-values are explained as a measure of the probability of observing results under the null hypothesis, not a measure of certainty.
Data dredging, or the practice of searching for patterns in data until something significant is found, is critiqued for its potential to mislead.
The speaker advises on critical thinking when interpreting statistical data, questioning the context and funding behind studies.
The importance of not blindly trusting media headlines and seeking a deeper understanding of the data presented is stressed.
The speaker invites questions from the audience, promoting an interactive discussion on the topic of statistics and their misuse.
A question from the audience about the downside of data dredging is addressed, emphasizing the importance of not validating hypotheses on the same data used to find patterns.
The philosophical nature of discerning truth from lies in statistics is touched upon, suggesting that a deeper conversation is needed.
The speaker concludes by encouraging the audience to be vigilant and question the data they encounter, promoting a healthy skepticism.
Transcripts
Browse More Related Video
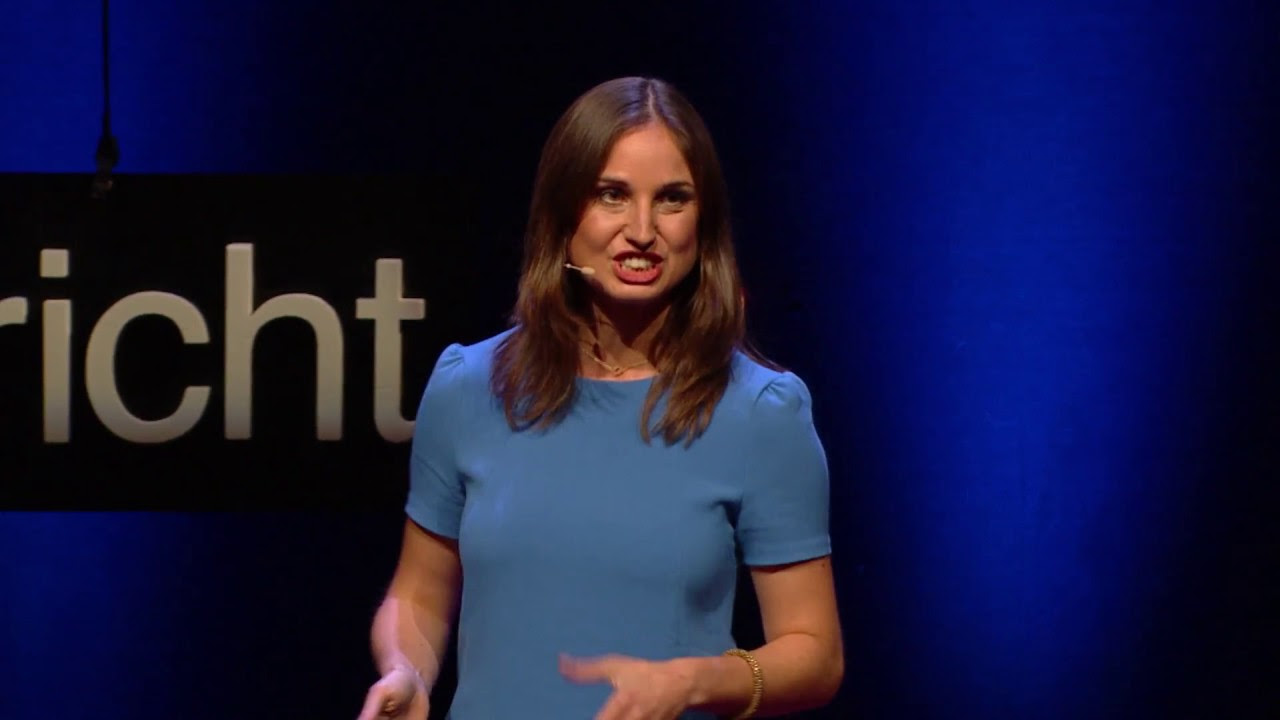
How to defend yourself against misleading statistics in the news | Sanne Blauw | TEDxMaastricht
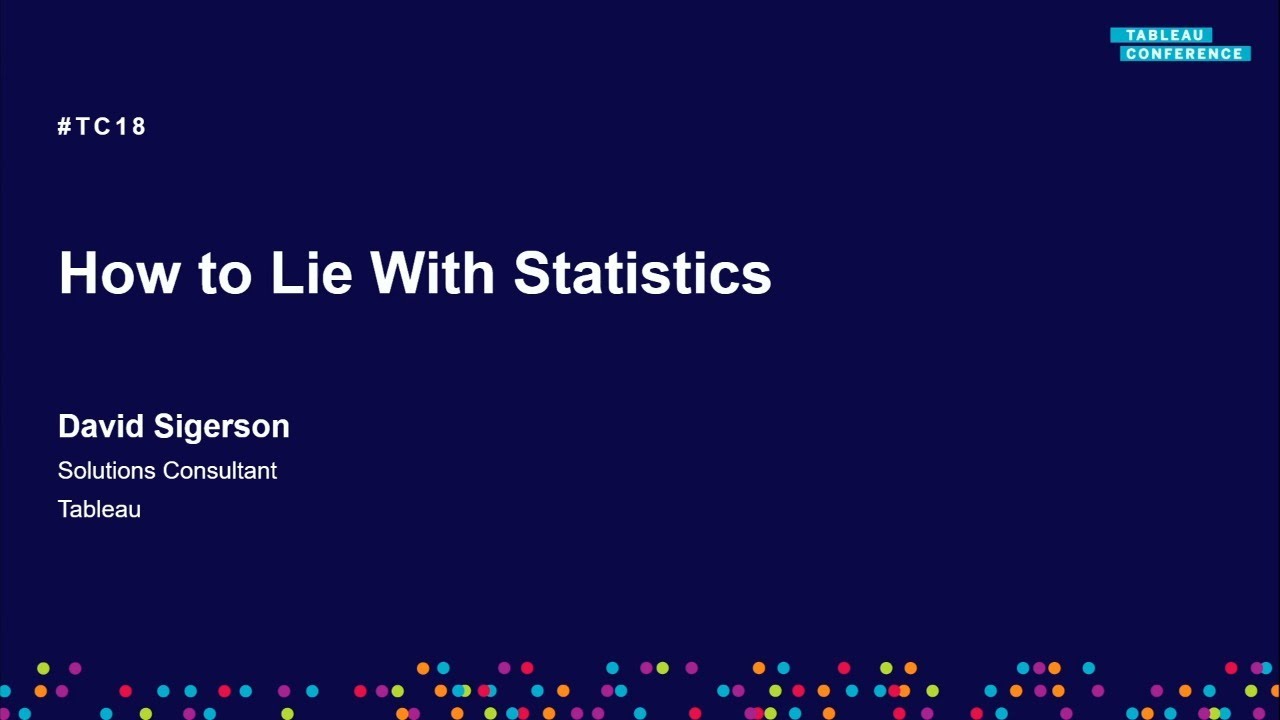
How to lie with statistics

Lies, Damned Lies, and Statistics: The misapplication of statistics in everyday life.
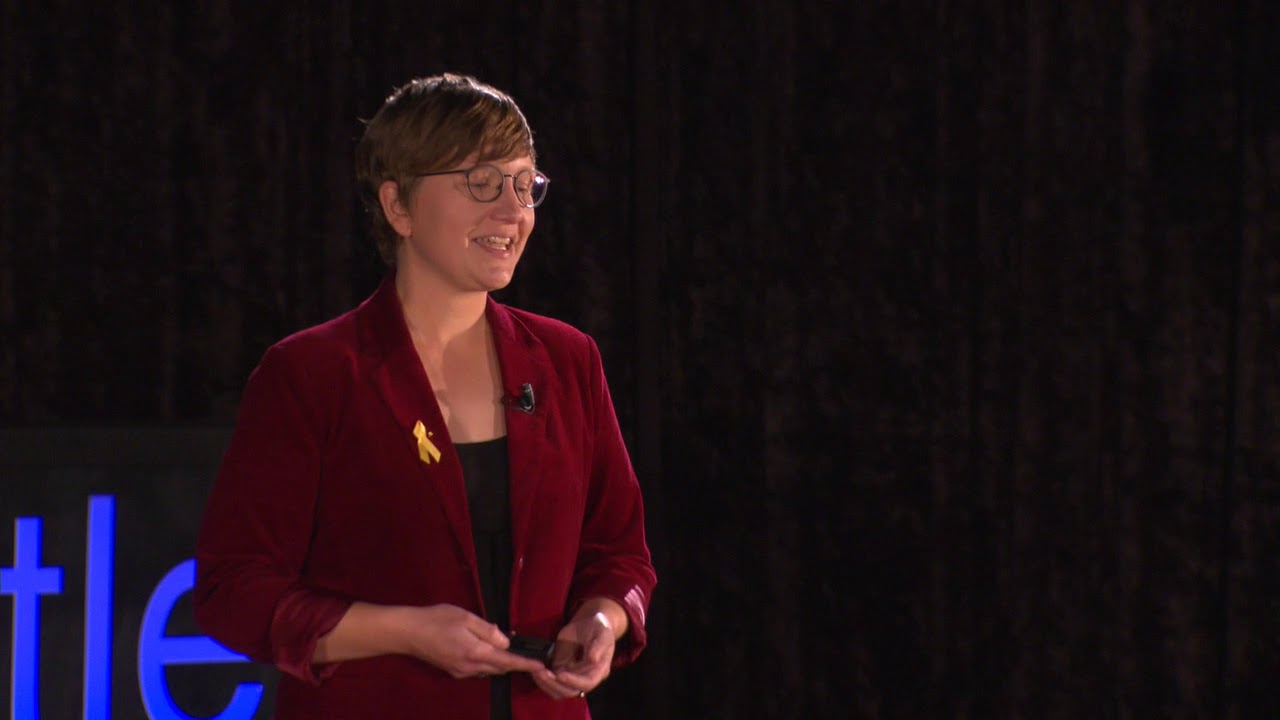
The Antidote to Lies, Damned Lies and Statistics | Emily Bird | TEDxFremantle
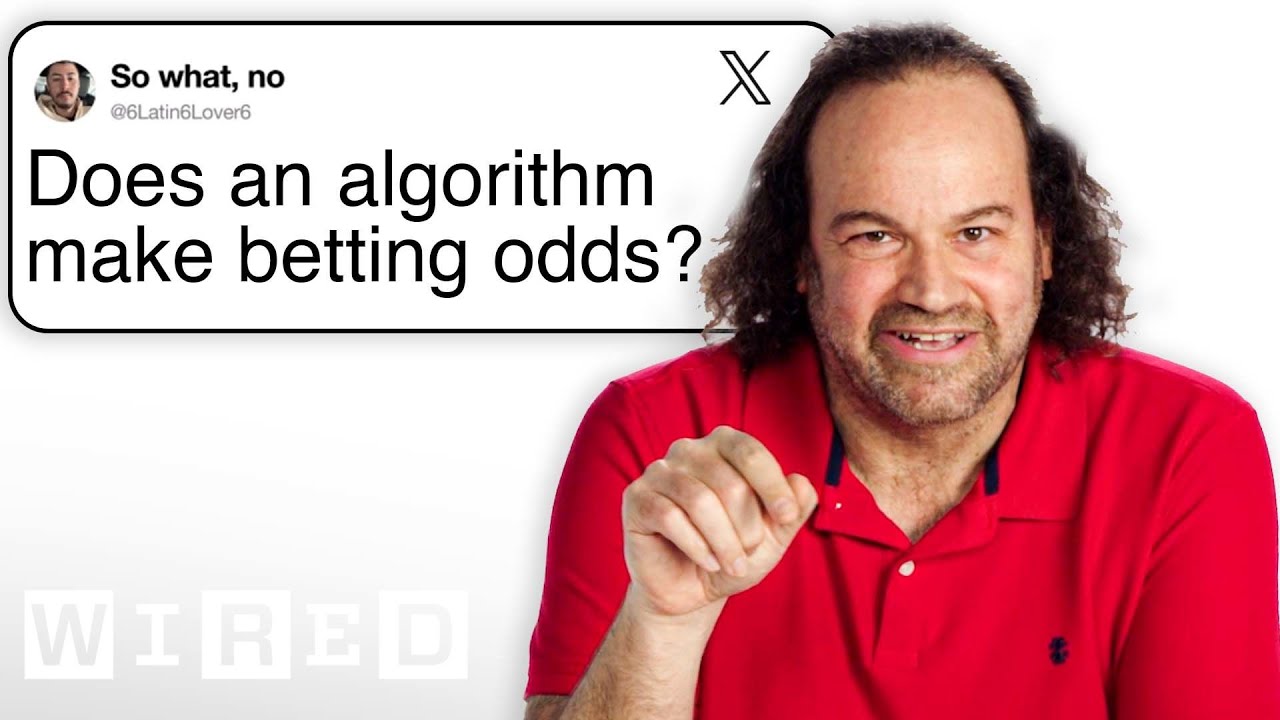
Statistician Answers Stats Questions From Twitter | Tech Support | WIRED

Elementary Statistics Chapter 1
5.0 / 5 (0 votes)
Thanks for rating: