Null Hypothesis, p-Value, Statistical Significance, Type 1 Error and Type 2 Error
TLDRThe video script offers a comprehensive guide to key concepts in biostatistics and epidemiology for the USMLE Step 1 Medical Board Exam. It explains the Null Hypothesis, which assumes no difference between study groups, and the Alternative Hypothesis, which posits a difference. The guide delves into Type I and Type II Errors, the significance of p-values and alpha levels, and the concept of statistical power. It emphasizes that a low p-value suggests evidence against the null hypothesis, while statistical significance doesn't always equate to clinical significance. The script also clarifies misconceptions about p-values and stresses the importance of study design and external data. It concludes with a brief overview of statistical hypothesis testing, mentioning specific tests like the t-Test, ANOVA, and chi-squared test for different types of data comparisons.
Takeaways
- π **Null Hypothesis (Hβ)**: The initial assumption that there is no difference between groups being studied.
- π **Alternative Hypothesis (Hβ)**: Opposite of the null hypothesis, suggesting a difference does exist between groups.
- π« **Type I Error**: Occurs when the null hypothesis is incorrectly rejected, leading to a false positive result.
- π **Type II Error**: Happens when the null hypothesis is not rejected when it should be, resulting in a false negative.
- π― **Power of a Test**: The probability of correctly rejecting the null hypothesis when there is a true effect, aiming for a high power (e.g., 80%).
- π **Increasing Power**: Can be achieved by increasing sample size, effect size, or precision of results.
- π² **p-Value**: The probability of observing the data (or more extreme) assuming the null hypothesis is true, indicating the strength of evidence against the null hypothesis.
- β **Statistical Significance**: Typically set at a 5% level of significance (alpha), meaning a p-value less than 0.05 suggests a significant result.
- π§ **Clinical vs. Statistical Significance**: Statistical significance does not always equate to a meaningful clinical impact.
- π **Sample Size Importance**: A small sample size can lead to incorrect conclusions, even when statistical significance is found.
- π **Contextualizing Results**: Statistical results must be interpreted within the context of study design and existing research.
- π **Hypothesis Testing for USMLE**: Knowledge of when to use different statistical tests (t-Test, ANOVA, chi-squared test) is more important than the mechanics for the exam.
Q & A
What is the null hypothesis in a medical study?
-The null hypothesis in a medical study states that there is no difference or relationship between the groups being studied with respect to the outcome of interest. For instance, when comparing a placebo group with a group receiving new medication, the null hypothesis posits that there would be no significant differences in outcomes such as blood sugar levels or medical complications between the two groups.
Why is the alternative hypothesis significant in medical research?
-The alternative hypothesis is significant because it suggests that there is a difference between the groups being studied, indicating a potential relationship between the treatment or risk factor and the health outcome. Researchers aim to prove the alternative hypothesis as it can lead to discoveries that improve patient outcomes or identify important risk factors for diseases.
What does rejecting the null hypothesis imply in statistical testing?
-Rejecting the null hypothesis implies that the data collected provides sufficient evidence to consider the null hypothesis unlikely. This rejection supports the alternative hypothesis, suggesting that there is a statistically significant difference between the groups being studied, although it does not prove the alternative hypothesis definitively.
What is a Type I error in the context of hypothesis testing?
-A Type I error occurs when the null hypothesis is incorrectly rejected when it is actually true. It is often considered a 'false positive' result, indicating a difference between groups when no such difference actually exists. The probability of committing a Type I error is denoted by alpha.
How does the concept of power relate to Type II errors?
-Power in statistical testing is defined as the probability of correctly rejecting the null hypothesis when it is false, thus it is the complement of a Type II error's probability (beta). High power means there is a lower chance of failing to detect a true effect when one actually exists, thereby reducing the risk of Type II errors.
What determines the statistical power of a study?
-Statistical power is influenced by several factors including the sample size, effect size, and the precision of measurements. Larger sample sizes and larger effect sizes increase the power of a study, as do more precise measurements (lower variability). High power is crucial to avoid Type II errors and ensure reliable study conclusions.
Why can't the p-value prove the alternative hypothesis?
-The p-value measures the probability of obtaining the observed results, or more extreme results, assuming the null hypothesis is true. It does not measure the probability of the null hypothesis being false nor does it prove the alternative hypothesis. A small p-value only indicates that the data are unlikely under the null hypothesis, suggesting the possibility of rejecting it.
What is the significance of the alpha level in hypothesis testing?
-The alpha level, commonly set at 0.05, represents the threshold probability at which the null hypothesis is rejected. It effectively sets the maximum allowed probability of committing a Type I error. If a p-value is less than the alpha level, the results are considered statistically significant, indicating strong evidence against the null hypothesis.
How does the design of a study affect its outcomes?
-The design of a study affects its outcomes by defining its structure, methodology, and how data are collected and analyzed. A well-designed study minimizes biases and errors, enhances the validity of the results, and ensures that the findings are reliable and can be generalized to a wider population.
What is the difference between statistical significance and clinical significance?
-Statistical significance refers to the likelihood that the observed differences between groups in a study are not due to random chance, as determined by the p-value. Clinical significance, however, deals with whether the differences, though statistically significant, are large enough to have a real-world, practical impact on patient care or outcomes.
Outlines
π Introduction to Biostatistics for USMLE Step 1
The video introduces the viewer to the Stomp on Step 1 series, which is aimed at future physicians preparing for the USMLE Step 1 Medical Board Exam. Brian McDaniel is the guide for this educational journey. The focus is on key biostatistics concepts such as the Null Hypothesis (H0), Alternative Hypothesis (Ha or H1), Type I and Type II Errors, p-Value, alpha, beta, power, and Statistical Significance. The video aims to cover these topics efficiently, breaking them down into understandable segments. The Null Hypothesis, which assumes no difference between study groups, is discussed in detail, along with the process of rejecting or failing to reject this hypothesis based on evidence. The Alternative Hypothesis, which assumes a difference, is also explained. The video emphasizes the importance of statistical significance in research and the concepts of Type 1 and Type 2 Errors, their probabilities (alpha and beta), and the calculation of study power.
π Understanding Power, p-value, and Significance Levels
This paragraph delves into the concept of Power in a study, which is the probability of finding a true difference between groups if one exists. It explains that high power is desirable, with a common threshold being 80%. Power calculations are often performed before starting a study to ensure the study design can detect a true effect if it exists. Factors that increase power include larger sample sizes, larger effect sizes, and increased precision of results. The paragraph also explains the p-value as the probability of obtaining a result at least as extreme as the observed one, assuming the null hypothesis is true. It clarifies that a low p-value indicates strong evidence against the null hypothesis, while a high p-value suggests the data are more consistent with the null hypothesis. The significance level, or alpha, is introduced as the predetermined threshold for determining statistical significance, with common levels being 5% or 1%. The video emphasizes that a p-value less than the alpha level indicates a statistically significant result.
π The Nuances of Statistical Significance and Hypothesis Testing
The video clarifies that a statistically significant result does not necessarily equate to clinical significance, which is the practical importance of a finding. It also dispels the common misconception that a p-value can be used to determine if observed differences are due to chance. The video stresses that the p-value is a measure of agreement or disagreement with the null hypothesis and that additional factors, such as study design and external data, must be considered to draw broader conclusions. It also discusses the limitations of relying solely on p-values, using the example of a coin flip to illustrate how small sample sizes can lead to misleading results. The paragraph concludes with a brief overview of statistical hypothesis testing for the USMLE Step 1, mentioning the use of t-Tests, ANOVA, and chi-squared tests in different scenarios.
π Acknowledging Support and Encouraging Further Learning
In the final paragraph, the speaker expresses gratitude to Brittany Hale and Dave Carlson for their donations, which helped fund the video. He encourages viewers to comment if they found the video useful and provides a direct link to the next video in the series about confidence intervals. The speaker wishes the viewers good luck with their studies, emphasizing the importance of understanding the covered topics for the USMLE Step 1 Medical Board Exam.
Mindmap
Keywords
π‘Null Hypothesis
π‘Alternative Hypothesis
π‘Type I Error
π‘Type II Error
π‘p-Value
π‘Alpha
π‘Beta
π‘Power
π‘Statistical Significance
π‘Clinical Significance
π‘Chi-Squared Test
Highlights
Stomp on Step 1 is a free video series designed to help future physicians study biostatistics and epidemiology more efficiently for the USMLE Step 1 Medical Board Exam.
The series is guided by Brian McDaniel, who explains complex statistical concepts in an accessible manner.
The Null Hypothesis (H0) is introduced as a statement of no difference between groups being studied.
The Alternative Hypothesis (Ha or H1) is the opposite of the Null Hypothesis, suggesting a difference between groups.
Researchers aim to reject the Null Hypothesis to support the Alternative Hypothesis, indicating a significant finding.
Type I Error occurs when the Null Hypothesis is incorrectly rejected, leading to a false positive result.
Type II Error happens when the Null Hypothesis is not rejected when it should be, resulting in a false negative.
The probability of making a Type I Error is denoted by alpha, while Type II Error probability is called beta.
Statistical Power is the probability of correctly rejecting the Null Hypothesis when there is a true effect, aiming for a high power (β₯80%).
Sample size, effect size, and precision directly influence the power of a study.
The p-value measures the probability of observing the data given the Null Hypothesis is true and indicates the strength of evidence against the Null Hypothesis.
A low p-value (typically <0.05) suggests strong evidence against the Null Hypothesis, while a high p-value indicates insufficient evidence to reject it.
Statistical significance (p-value < alpha) does not equate to clinical significance, which considers the practical impact of findings.
The p-value should not be used in isolation to determine if observed differences are due to chance; study design and external evidence are crucial.
Common statistical tests for different scenarios are t-Test for two groups, ANOVA for three or more groups, and chi-squared test for categorical variables.
Understanding the limitations of p-values and the importance of study design is essential for interpreting research findings accurately.
The video concludes with a reminder that statistical hypothesis testing is a part of the USMLE Step 1 exam, emphasizing the need to know when to apply different tests.
Transcripts
Browse More Related Video
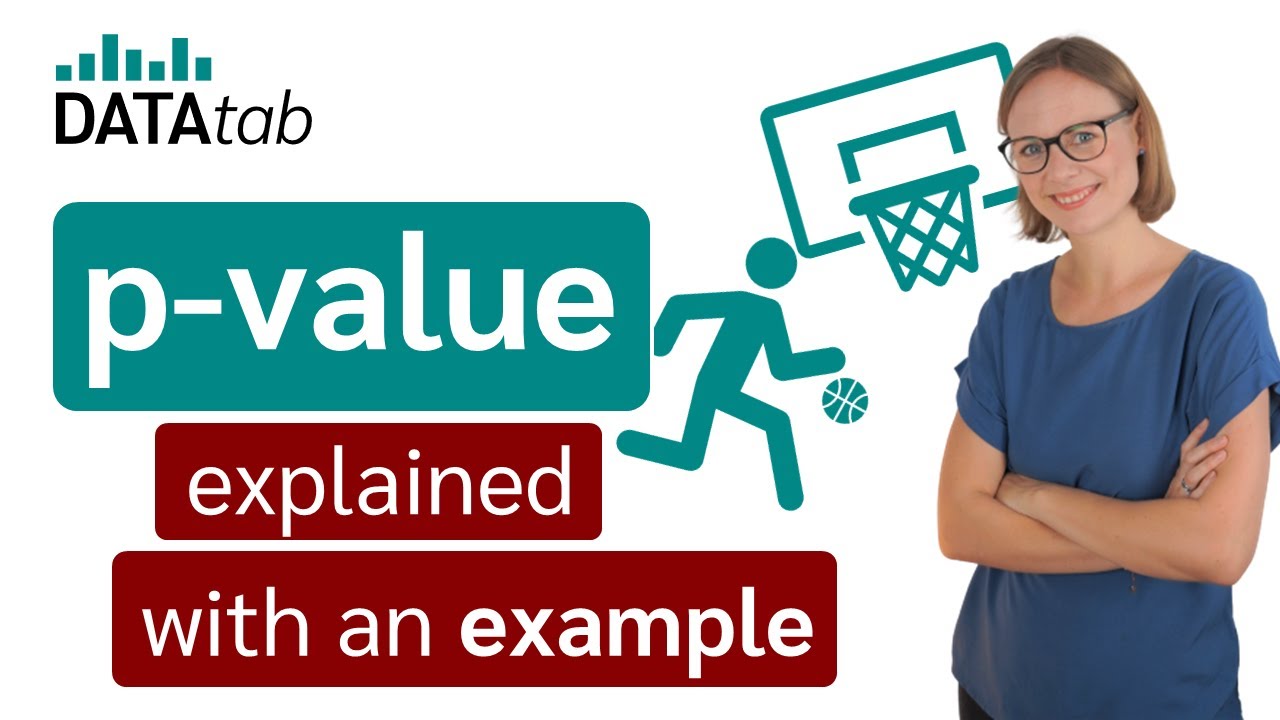
p-value - easily explained with an example
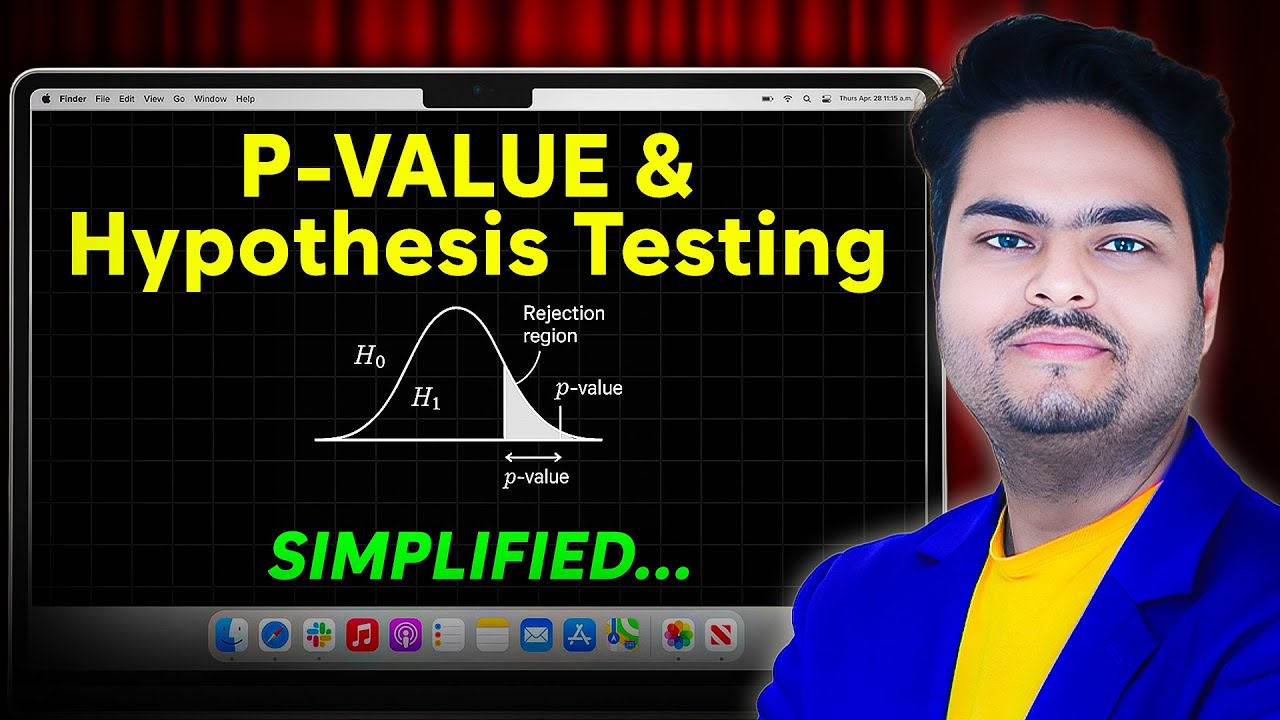
P Value and Hypothesis Testing Simplified|P-value and Hypothesis testing concepts in Statistics
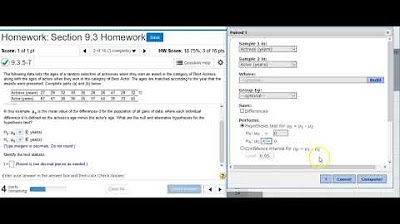
Using StatCrunch to perform hypothesis testing on two matched pair means of acting award ages
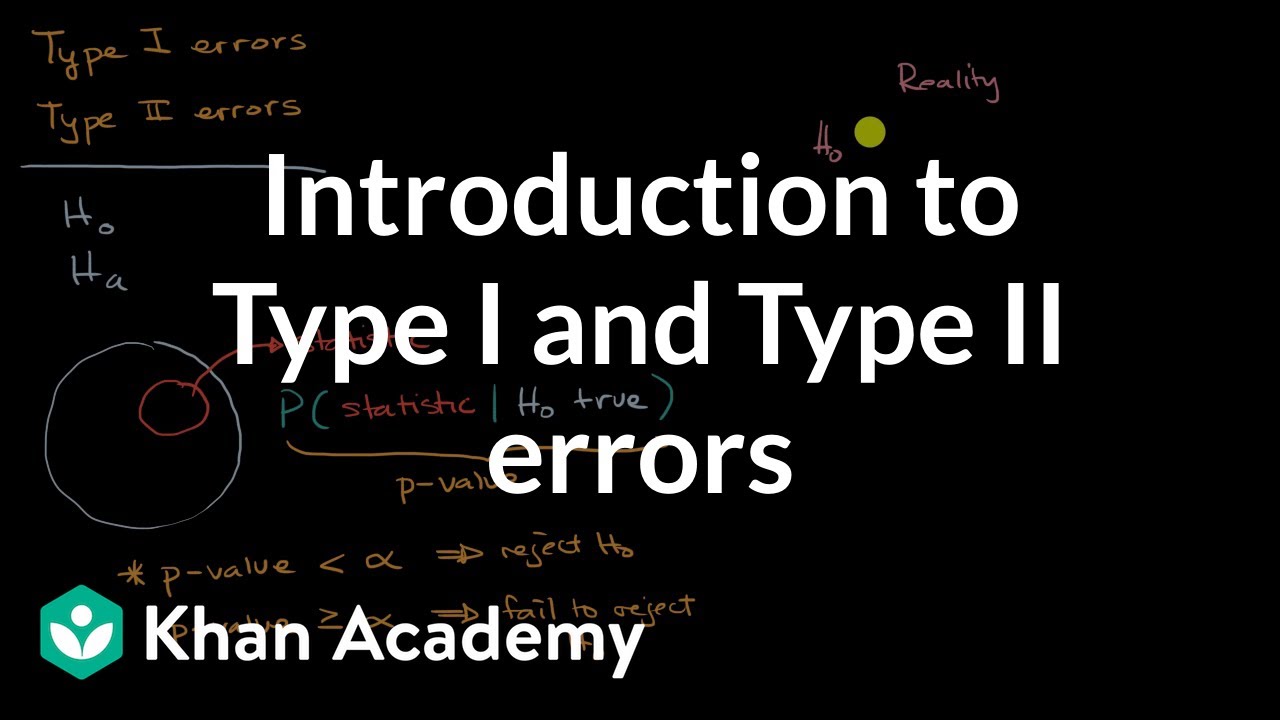
Introduction to Type I and Type II errors | AP Statistics | Khan Academy
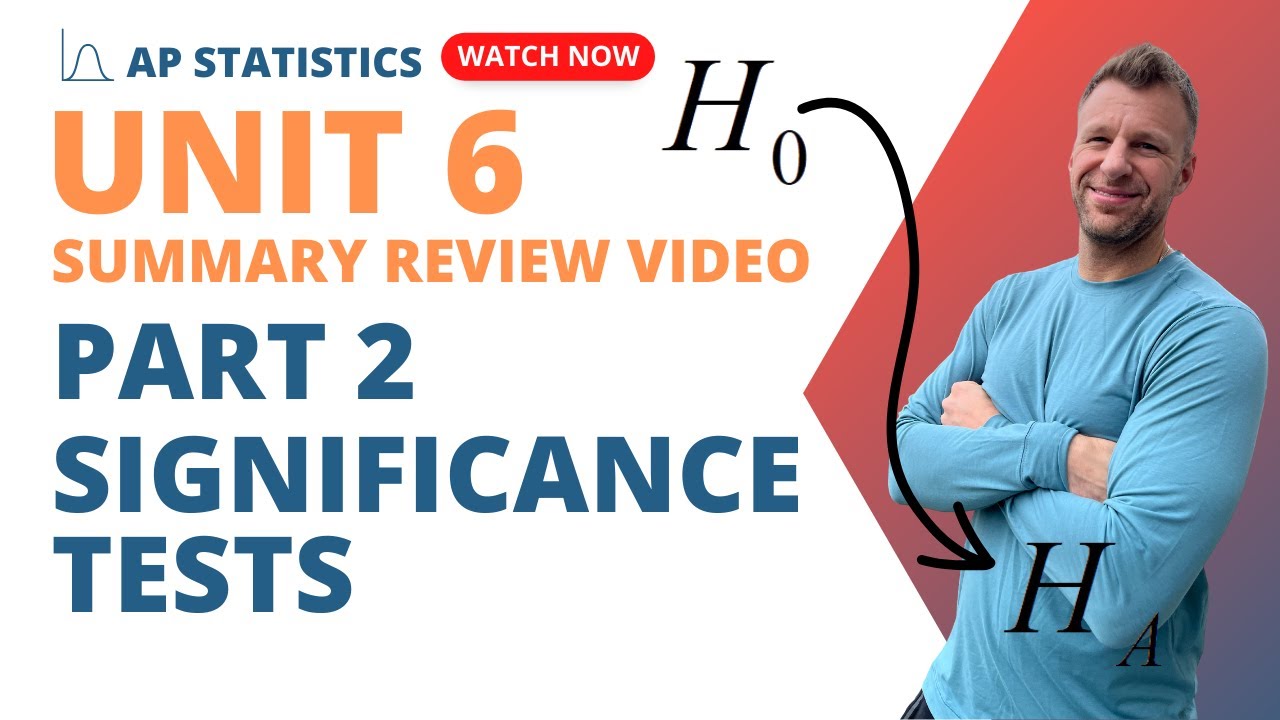
AP Statistics Unit 6 Summary Review Inference for Proportions Part 2 Significance Tests
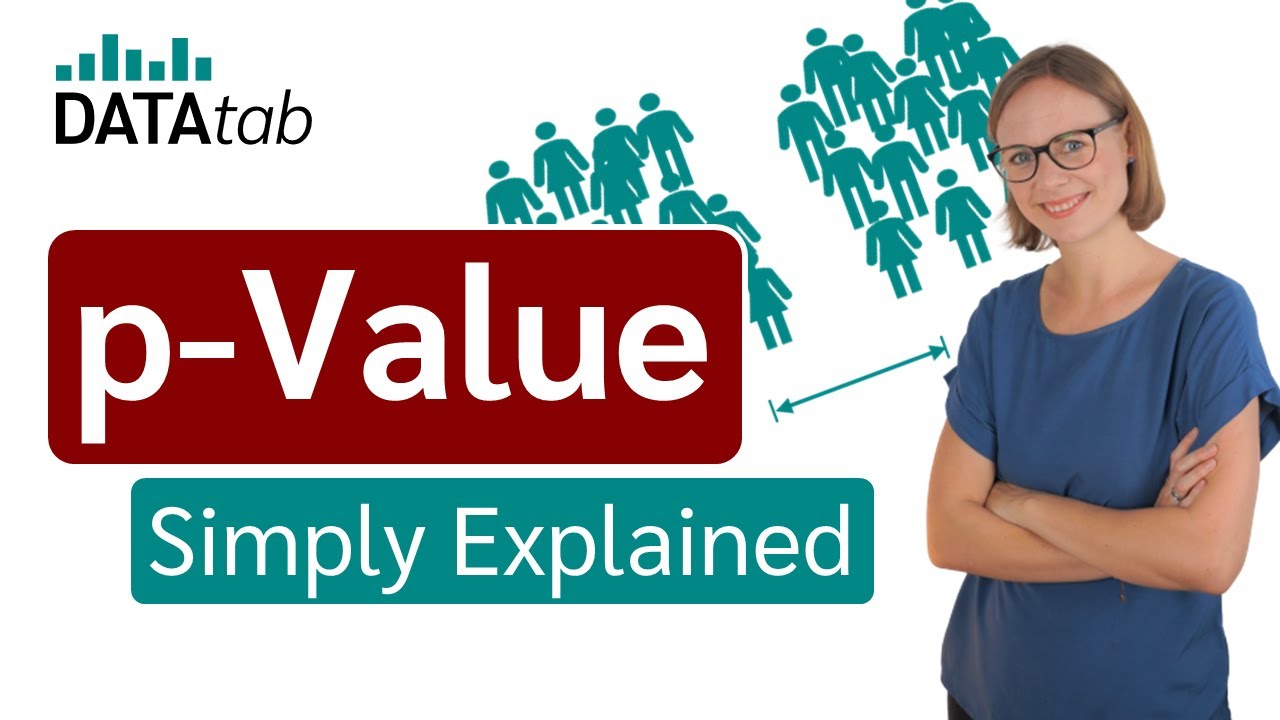
p-Value (Statistics made simple)
5.0 / 5 (0 votes)
Thanks for rating: