The Gradient of a Function (Calculus 3)
TLDRThis video from Houston Math Prep delves into the concept of gradients, explaining their significance in multi-variable calculus. The gradient, represented by the del operator, is a vector that indicates the direction of the greatest rate of increase for a scalar function. The tutorial covers how to calculate the gradient for functions of two and three variables, using examples to illustrate the process. It also discusses the magnitude of the gradient, which reflects the steepness of the increase, and touches on finding directions of greatest increase, decrease, and no change on a function's surface.
Takeaways
- π The video is an educational resource from Houston Math Prep, focusing on explaining the concept of gradients in multi-variable calculus.
- π The gradient, denoted by 'nabla' or 'del', is a vector that points in the direction of the greatest increase of a scalar function at a given point.
- π The gradient operation is applied to scalar functions, but the result is a vector function, indicating the direction of steepest ascent on a surface.
- π For a function of two variables, the gradient is calculated using partial derivatives with respect to each variable, resulting in a two-component vector.
- π The gradient can also be extended to functions of three variables, resulting in a three-component vector, indicating direction in 3D space.
- π The magnitude of the gradient vector represents the rate of increase at a point, with larger magnitudes indicating steeper ascents.
- π The direction of the gradient is always perpendicular to the level curves of the function and points towards the direction of the greatest increase.
- π§ The gradient at a specific point is found by substituting the coordinates of that point into the gradient vector function.
- π The gradient vector can be normalized to a unit vector to provide a direction without regard to the rate of increase.
- π The orthogonal direction to the gradient, where there is no increase or decrease, can be found by considering vectors perpendicular to the gradient.
- π The script also mentions an upcoming video on directional derivatives, which will explore how to find rates of change in any given direction using the gradient.
Q & A
What is the gradient in the context of multi-variable calculus?
-The gradient is a vector function that represents the direction of the greatest increase of a scalar function at any given point in multi-variable calculus. It is denoted by the symbol 'nabla' or 'del' and is calculated as the partial derivatives of the function with respect to each variable.
How is the gradient of a scalar function represented mathematically?
-The gradient of a scalar function is represented as the vector function with components being the partial derivatives of the function with respect to each variable. For a function of two variables, it is represented as del f = (βf/βx, βf/βy).
What does the gradient vector at a point on a function indicate?
-The gradient vector at a point on a function indicates the direction of the steepest ascent at that point. It points towards the direction where the function increases the most rapidly.
How does the gradient change when considering a function of three variables instead of two?
-When considering a function of three variables, the gradient remains a vector function but now has three components, with the third component being the partial derivative of the function with respect to the third variable, z.
What is the gradient of the function f(x, y) = x^2 + y^2?
-The gradient of the function f(x, y) = x^2 + y^2 is del f = (2x, 2y), which means the gradient vector at any point (x, y) is proportional to the position vector (x, y).
How do you find the gradient of a function at a specific point?
-To find the gradient of a function at a specific point, you evaluate the gradient vector function at that point by substituting the coordinates of the point into the components of the gradient vector.
What is the relationship between the gradient and the level curves of a function?
-The gradient is always perpendicular to the level curves of a function. It points in the direction of the greatest increase, which is normal to the level curve at any point.
How does the magnitude of the gradient vector relate to the rate of increase of a function?
-The magnitude of the gradient vector represents the rate of the greatest increase of the function. A larger magnitude indicates a steeper ascent, while a smaller magnitude indicates a gentler slope.
What is the gradient of the function f(x, y) = sqrt(36 - x^2 - y^2) at the point (-3, 2)?
-The gradient of the function at the point (-3, 2) is calculated by substituting x and y into the gradient vector, resulting in a vector with components (3/β23, -2/β23) after simplification.
How can you find a direction of no change (i.e., a direction tangent to the level curve) at a given point on a function?
-To find a direction of no change, you need a vector that is orthogonal to the gradient at that point. This means the dot product of the gradient vector and the direction vector should be zero. A common approach is to find a vector that, when dotted with the gradient, yields zero, and then normalize this vector to get a unit vector in that direction.
Outlines
π Introduction to Gradients in Multivariable Calculus
This paragraph introduces the concept of gradients in the context of multivariable calculus. It explains that the gradient of a scalar function, represented by the del or nabla symbol, indicates the direction of the greatest increase at any given point on the function. The gradient is computed as a vector function with components derived from partial derivatives with respect to each variable. The paragraph provides an example of a function of x and y, calculates the gradient, and evaluates it at specific points to demonstrate how the gradient changes direction depending on the location on the function's surface.
π Calculating Gradients and Their Magnitude
This section delves deeper into calculating the gradient of a function and interpreting its magnitude. It explains the process of finding the gradient for a given function, which involves taking partial derivatives with respect to each variable. The paragraph also discusses evaluating the gradient at a specific point, illustrating how to find the direction and rate of the greatest increase or decrease. It uses an example involving the square root of a quadratic expression to demonstrate these concepts and shows how the gradient vector always points towards the origin for that particular function, indicating the direction of steepest ascent.
π§ Direction of Greatest Increase and Decrease
The third paragraph focuses on determining the direction and rate of the greatest increase and decrease for a given function at a specific point. It explains how the gradient vector points in the direction of the steepest ascent and how its magnitude represents the rate of this increase. The paragraph provides a method to find the unit vector in the direction of the gradient for clarity and uses another function to demonstrate the process of finding the gradient, its evaluation at a point, and the calculation of the magnitude to determine the rate of increase. It also discusses the concept of the direction of greatest decrease, which is the opposite direction of the gradient.
π Finding Directions of No Change and Orthogonality
The final paragraph discusses the concept of finding a direction of no change on a function's surface, which is orthogonal to the gradient. It explains that moving in this direction would not result in any increase or decrease in the function's value, effectively staying on the same level curve. The paragraph provides a method to find such a direction by ensuring the dot product between the gradient and another vector is zero, indicating orthogonality. It uses a specific function to demonstrate how to find this direction, normalize it to a unit vector, and discusses the implications of moving in an orthogonal direction to the gradient.
Mindmap
Keywords
π‘Gradient
π‘Scalar Function
π‘Partial Derivative
π‘Nabla
π‘Vector Function
π‘Direction of Greatest Increase
π‘Level Curves
π‘Magnitude
π‘Unit Vector
π‘Orthogonal
π‘Directional Derivative
Highlights
The video explains the concept of gradients in the context of multi-variable calculus.
Gradients indicate the direction of greatest increase for a scalar function at a given point.
The gradient is represented by the del or nabla symbol and is a vector function.
For a function of two variables, the gradient has two components: partial derivatives with respect to x and y.
In a 3D space, the gradient of a function has three components, including the partial derivative with respect to z.
The first example demonstrates calculating the gradient of a function f(x, y) = x^2 + y^2.
The gradient at a specific point is found by substituting the point's coordinates into the gradient vector function.
The gradient's direction at a point can vary, indicating different directions of greatest increase.
The magnitude of the gradient vector represents the steepest rate of increase at a point.
The second example involves a function f(x, y) = sqrt(36 - x^2 - y^2) and its gradient calculation.
The gradient of the second example function always points toward the origin, indicating the direction of steepest ascent.
The gradient's magnitude near the origin is smaller, indicating a less steep uphill climb.
The third example explores the function f(x, y) = x^2y - xy^2 and its gradient at the point (1, -1).
The gradient's direction and rate of greatest increase are derived from the gradient vector at a specific point.
The direction of greatest decrease is the opposite of the gradient's direction of greatest increase.
A direction of no change is found by identifying a vector orthogonal to the gradient, resulting in a dot product of zero.
The video concludes with a preview of the next topic: directional derivatives and their application using gradients.
Transcripts
Browse More Related Video

Directional Derivatives (Calculus 3)
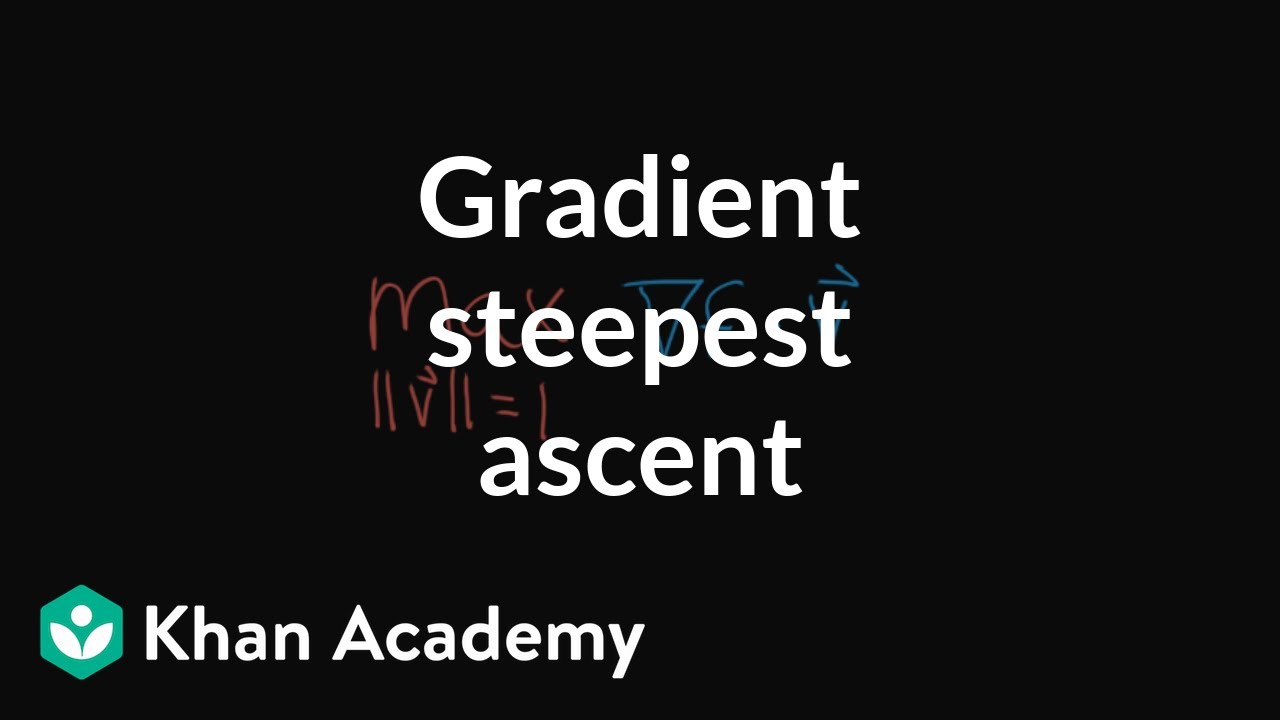
Why the gradient is the direction of steepest ascent
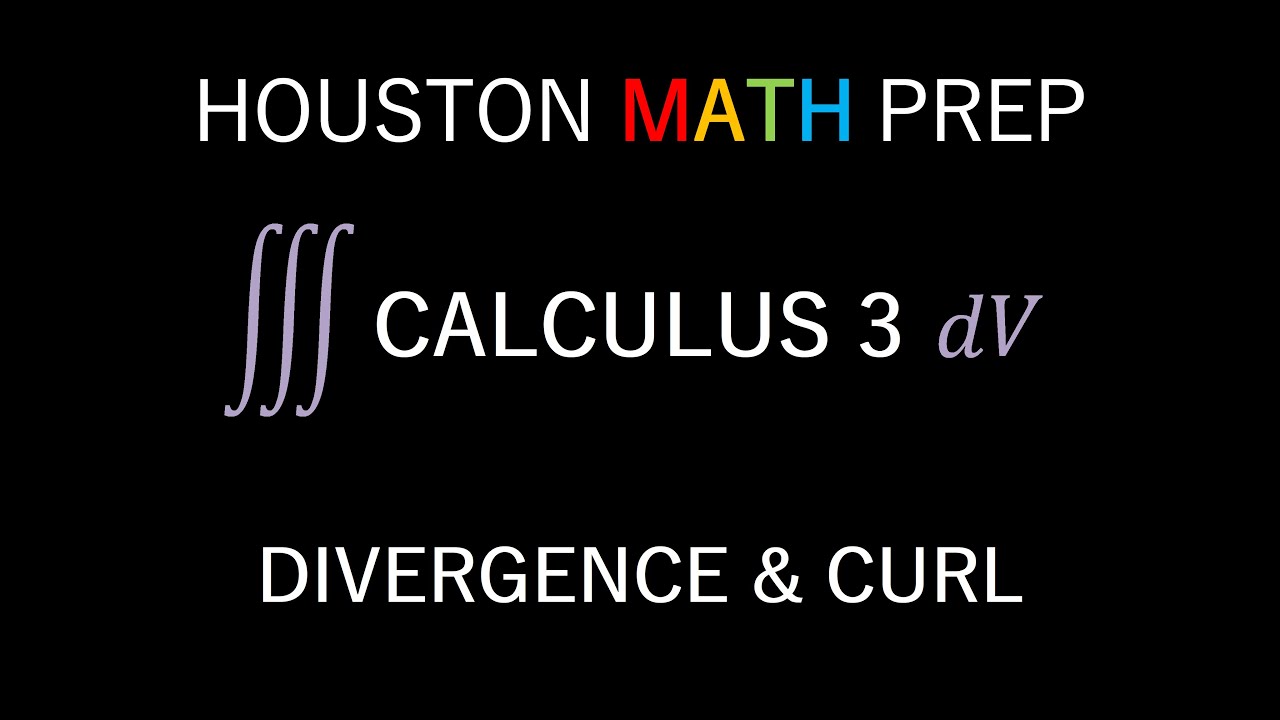
Divergence and Curl (Vector Fields)

Gradient 1 | Partial derivatives, gradient, divergence, curl | Multivariable Calculus | Khan Academy

Tensor Calculus 2: The Two Conflicting Definitions of the Gradient
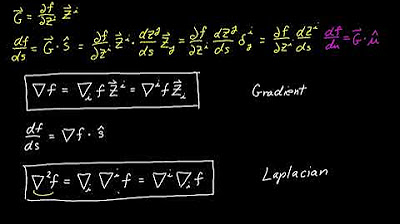
Video 39 - Gradient
5.0 / 5 (0 votes)
Thanks for rating: