Bias Detection (in Meta-Analyses)
TLDRThis video script delves into the critical issue of bias in scientific research and literature. It emphasizes the importance of recognizing and preventing bias, intentional or unintentional, throughout the research cycle. The script discusses various forms of bias, including research misconduct, statistical reporting errors, and publication bias. It also introduces tools and techniques for detecting bias, such as the Grim test, the trim and fill method, and p-curve analysis. However, it acknowledges the limitations of these tools, noting that while they can indicate the presence of bias, they cannot accurately correct for it. The script concludes by highlighting the pervasiveness of bias in scientific literature and the necessity for researchers and evaluators to remain vigilant.
Takeaways
- π΅οΈ Bias can be introduced at various stages in the research cycle, and it's crucial to be aware of its potential presence.
- π Tools are available to help detect bias in scientific literature, which is important for both researchers and those evaluating published findings.
- π Research misconduct, such as falsifying data or results, can have significant impacts, as seen in the retracted study linking the MMR vaccine to autism.
- π€ Statistical reporting errors, both unintentional and intentional, can lead to inaccurate representations of research findings.
- π οΈ A statistical tool developed by Michelle Knight and colleagues can help prevent errors in manuscripts by checking for incorrect p-values and other statistical inconsistencies.
- π Inconsistencies in published results, like reported means that are statistically impossible given the sample size, can indicate bias.
- π§ Hypothesizing after results are known can lead to inflated type 1 error rates, as researchers may generate hypotheses based on statistically significant findings.
- π Publication bias is a major concern, as research published in literature may not accurately represent the full population of completed studies.
- π Funnel plots can be used to visually detect bias in meta-analyses by comparing the distribution of study effect sizes and standard errors.
- π The trim and fill technique is a method proposed to detect and 'correct' for publication bias, though it may not provide accurate unbiased effect size estimates.
- π Meta-regression and p-curve analysis are additional techniques for detecting bias by examining the distribution of p-values and the relationship between effect sizes and standard errors.
Q & A
What is the importance of being aware of bias in scientific literature?
-Being aware of bias in scientific literature is crucial because it can affect the validity and reliability of research findings. It helps researchers prevent introducing bias in their own work and allows them to critically evaluate published findings.
What does Deborah Mayo suggest as a first question when confronted with statistical news flash of the day?
-Deborah Mayo suggests asking whether the results are due to selective reporting, cherry-picking, or any number of other similar biases as the first question when confronted with statistical news flash of the day.
What is an example of research misconduct mentioned in the script?
-An example of research misconduct mentioned in the script is a paper by Andrew Wakefield, which claimed a link between the measles, mumps, and rubella vaccine (MMR) and autism. This paper greatly impacted the public's perception and was eventually retracted in 2010.
What is a grim test and why is it significant?
-A grim test checks for inconsistencies in published results, specifically whether reported means are statistically possible given the sample sizes. It is significant because it helps detect errors and biases in reported data.
What is hypothesizing after the results are known and why can it be problematic?
-Hypothesizing after the results are known involves generating a hypothesis based on statistically significant findings rather than testing a prediction. This can be problematic as it can greatly inflate the type 1 error rate, leading to false positives.
What is publication bias and why is it a concern in scientific literature?
-Publication bias refers to the systematic unrepresentativeness of published research compared to the actual population of completed studies. It is a concern because it can skew the understanding of a field, making it seem as though positive results are more common than they actually are.
What is the trim and fill technique and what is its limitation?
-The trim and fill technique is a method used to detect and attempt to correct for publication bias by adding 'missing' studies to a meta-analysis to balance the distribution of effect sizes. However, its limitation is that it does not accurately correct bias because it makes assumptions about the missing studies, which may not be true.
What is meta-regression and how does it help in bias detection?
-Meta-regression is a technique that estimates the unbiased meta-analytic effect size by analyzing the relationship between effect sizes and study characteristics. It helps in bias detection by comparing the observed effect sizes with what would be expected in the absence of bias.
What is P-curve analysis and how does it work?
-P-curve analysis is a bias detection technique that focuses on the distribution of p-values across studies. It compares the observed p-value distribution with what would be expected under the null hypothesis or with some power, helping to identify potential bias in the literature.
Why is it important to decide when to perform a meta-analysis?
-Deciding when to perform a meta-analysis is important because the timing can introduce bias. Performing a meta-analysis after observing non-significant results can skew the findings, making them appear more significant than they are, thus affecting the reliability of the meta-analysis.
What does the script suggest about the state of scientific literature?
-The script suggests that scientific literature is not unbiased and that researchers need to be aware of this when evaluating research findings. It emphasizes the importance of using tools and techniques to detect and understand the presence of bias.
Outlines
π΅οΈββοΈ Detecting and Preventing Bias in Scientific Research
This paragraph emphasizes the importance of being aware of potential biases in scientific literature. It suggests having tools to detect bias and understanding how to prevent it in one's own research. The discussion includes the distinction between intentional and unintentional biases, such as research misconduct exemplified by the retracted Andrew Wakefield paper linking MMR vaccine to autism, and statistical reporting errors. It introduces a statistical tool developed by Michelle Knight and colleagues to help prevent such errors in APA style manuscripts. The paragraph also touches on the grim test for detecting impossible means in reported data and the issue of hypothesizing after results are known, which can lead to inflated type 1 error rates. Lastly, it addresses publication bias and how research published in literature may not accurately represent the entire body of completed studies.
π Publication Bias and Techniques for Detection
The second paragraph delves into the challenges of publication bias, where research findings in the literature may not be representative due to various reasons such as personal judgment based on results or a preference for reporting positive outcomes. It acknowledges the difficulty of correcting for publication bias, as accurately modeling the bias is not feasible. The paragraph introduces several techniques for detecting bias, including funnel plots for visualizing meta-analysis results, the trim and fill method for inferring and adjusting for missing studies, and meta-regression techniques like PET and PEAS to estimate the unbiased effect size. However, it also highlights the limitations of these methods, as they are based on certain assumptions and cannot provide definitive answers about the presence of bias.
π Advanced Bias Detection Methods and Their Limitations
This paragraph discusses advanced methods for detecting and attempting to correct bias in meta-analyses. It describes the P-curve analysis, which examines the distribution of p-values to identify potential bias, such as the tendency to report only significant results or to perform covariate analysis until significance is achieved. The paragraph also addresses the limitations of bias correction techniques, noting that they can only provide indications of bias under specific models and assumptions. The discussion serves as a caution that while these tools can raise red flags for potential bias, they cannot guarantee the complete absence of bias if not detected.
π The Reality of Bias in Scientific Literature
The final paragraph acknowledges the unfortunate reality that scientific literature is not free from bias. It stresses the importance of considering this when evaluating research findings. The paragraph serves as a reminder that while tools and techniques can help detect and mitigate bias, complete elimination of bias is challenging, and researchers and readers of scientific literature must remain vigilant and critical in their approach.
Mindmap
Keywords
π‘Bias
π‘Selective Reporting
π‘Research Misconduct
π‘Statistical Reporting Errors
π‘P-Value Manipulation
π‘Grim Test
π‘Hypothesizing After Results are Known
π‘Publication Bias
π‘Trim and Fill Technique
π‘Funnel Plot
π‘P-Curve Analysis
π‘Meta-Regression
Highlights
The importance of being aware of potential bias in scientific literature and the need for tools to detect it.
Bias can be introduced intentionally or unintentionally throughout the research process.
Advice from Deborah Mayo on questioning the validity of statistical news due to potential bias.
Research misconduct, such as altering results, leading to inaccurate representations of reality.
The retraction of Andrew Wakefield's paper linking MMR vaccine to autism due to misconduct.
Unintentional statistical reporting errors and their potential impact on research.
The development of a statistical tool to prevent errors in manuscript reporting, similar to a spell check.
Inconsistencies in published results, such as reported means not being possible given sample sizes.
The Grim Test for identifying statistically impossible means in published research.
The issue of hypothesizing after results are known, leading to inflated type 1 error rates.
The impact of publication bias on the representation of completed studies in the literature.
The difficulty in correcting for publication bias due to the inability to accurately model it.
Older bias detection techniques like fail-safe N that are no longer considered reliable.
The use of funnel plots to visually detect bias in meta-analysis.
The trim and fill technique for attempting to correct publication bias, despite its limitations.
Meta-regression as a method for estimating unbiased effect sizes in meta-analysis.
The P-curve analysis for detecting bias based on the distribution of p-values.
The limitations of bias detection techniques and the importance of considering their assumptions.
The potential bias introduced by the timing and decision-making process of conducting a meta-analysis.
The acknowledgment that scientific literature is not unbiased and the need for critical evaluation.
Transcripts
Browse More Related Video

Techniques for random sampling and avoiding bias | Study design | AP Statistics | Khan Academy
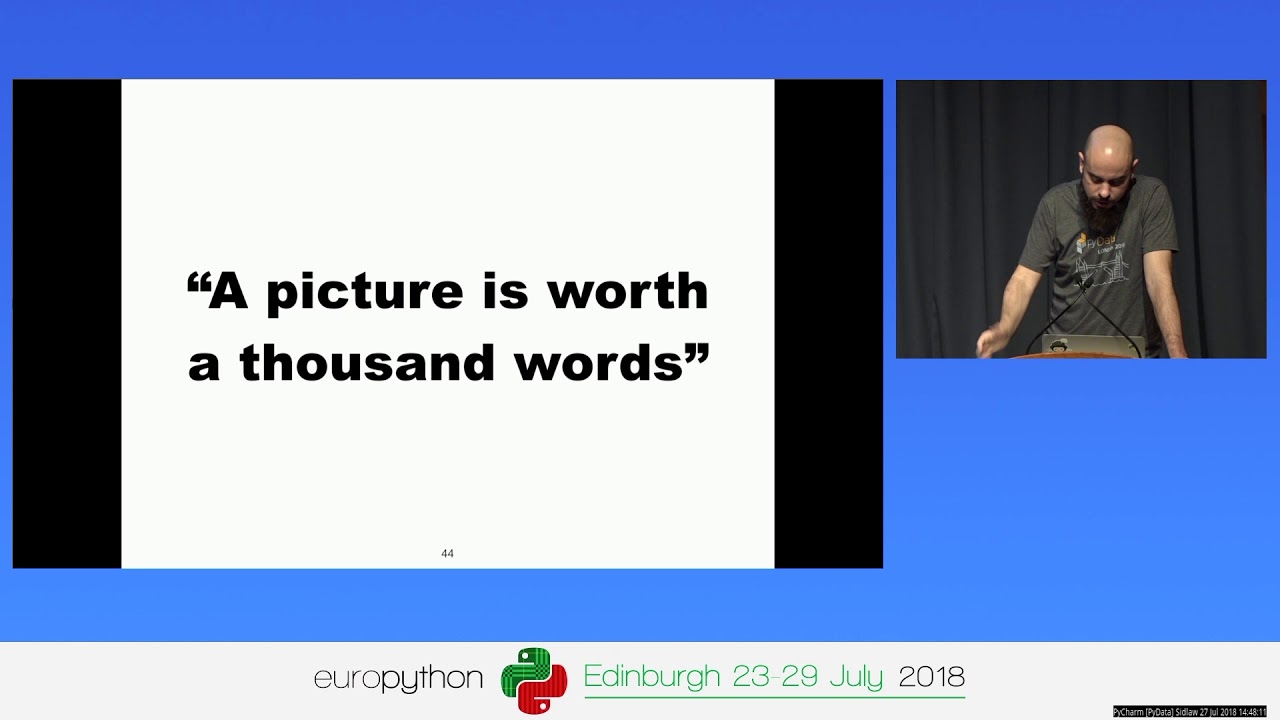
Marco Bonzanini - Lies, damned lies, and statistics
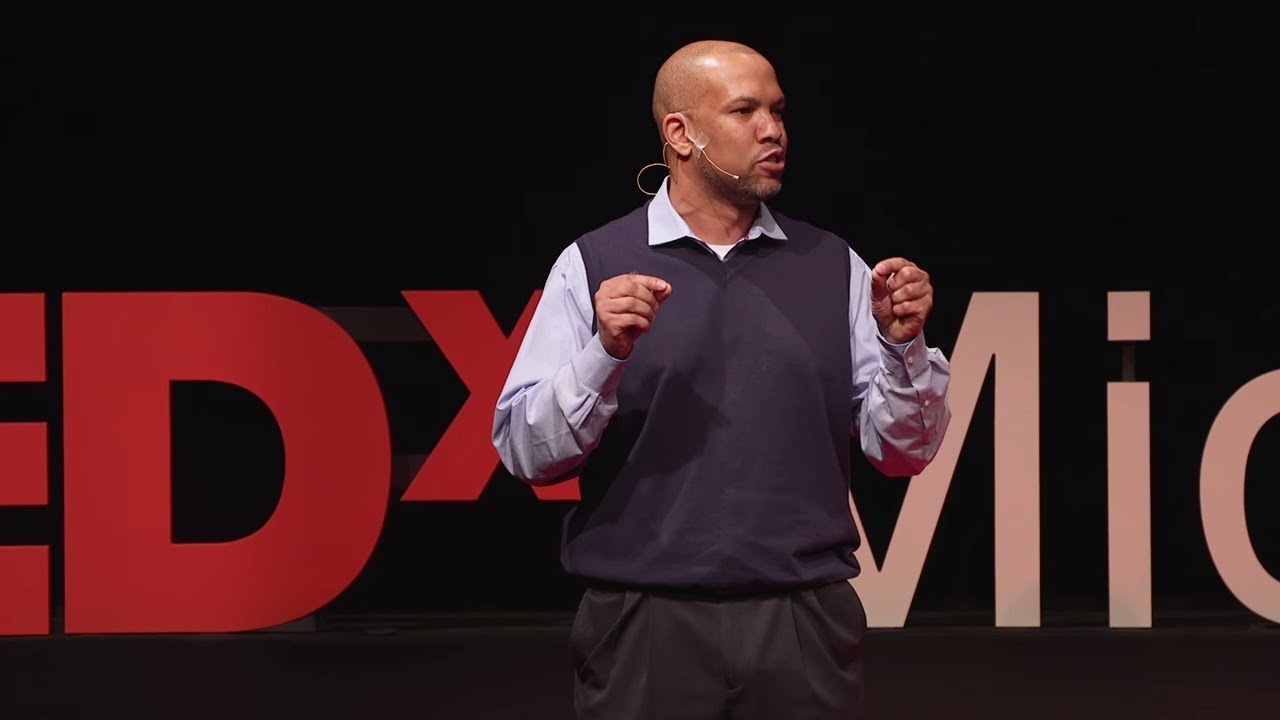
We all have implicit biases. So what can we do about it? | Dushaw Hockett | TEDxMidAtlanticSalon
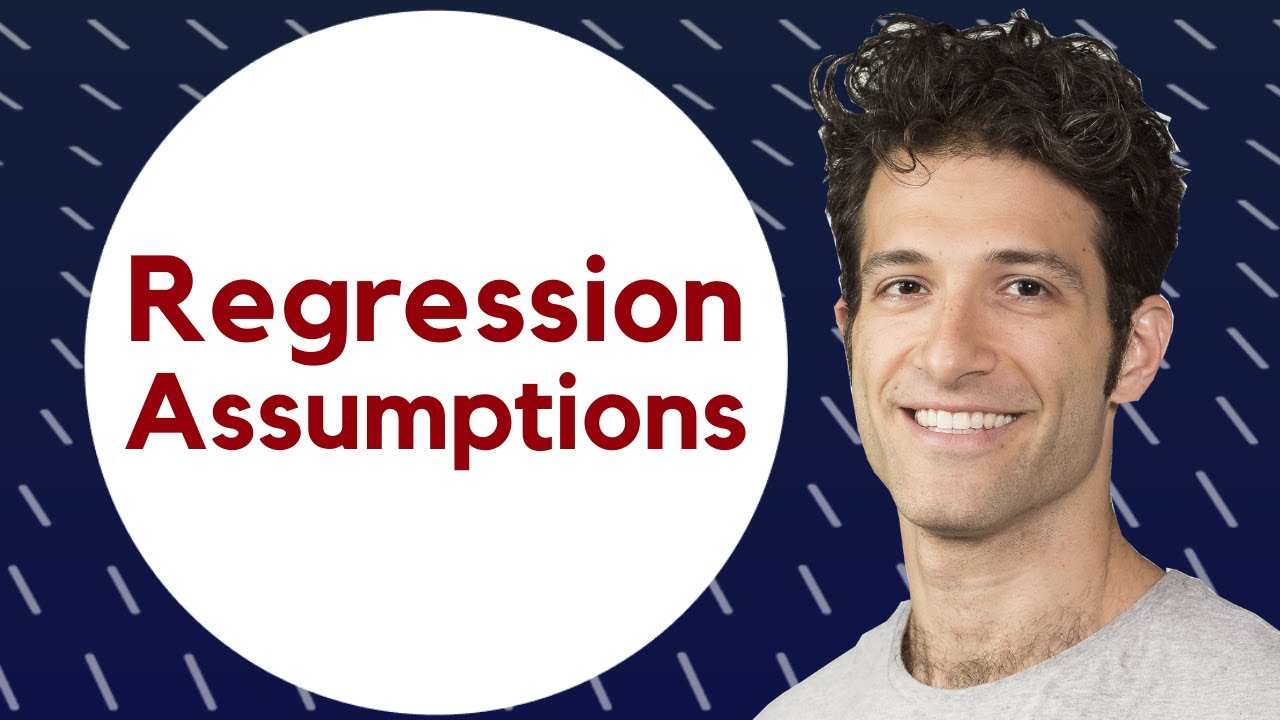
Regression assumptions explained!

AP Psychology | Myersβ Unit 2
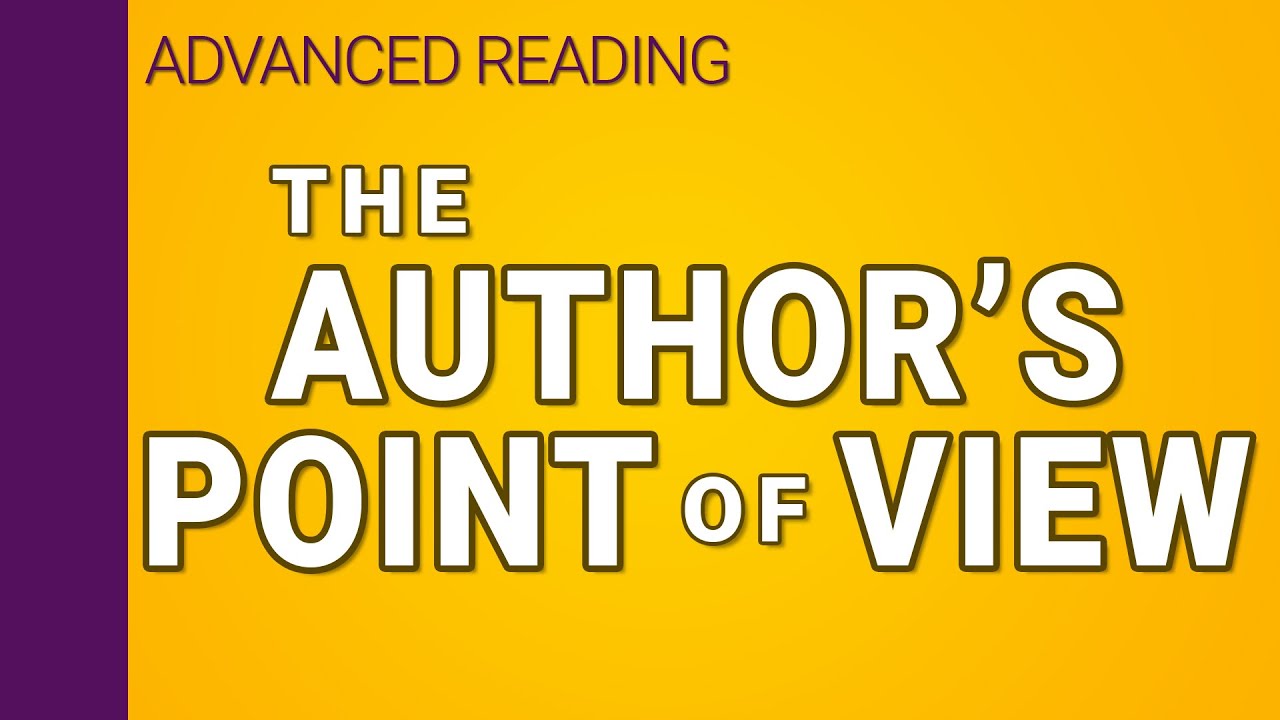
The author's point of view in writing (2/3) | Interpreting Series
5.0 / 5 (0 votes)
Thanks for rating: