Top 10 Tips for AP Statistics Unit 3 Collecting Data
TLDRIn this video, SAT expert Michael Princhak presents the top 10 essential concepts from AP Statistics Unit 3, focusing on data collection. He emphasizes the importance of understanding the goal of collecting data, which is to estimate a population parameter through a sample statistic. Princhak explains the differences between observational and experimental studies, the various sampling techniques, and the crucial role of randomization in reducing bias and ensuring representative samples. He also delves into the principles of good experimental design, including treatments, randomization, replication, and direct control. The video concludes with advice on generalizing results from samples and experiments, highlighting the significance of random selection and assignment in making inferences about the larger population.
Takeaways
- π **Goal of Collecting Data**: The primary aim is to estimate a population parameter using a sample statistic, accounting for sampling variability.
- π **Types of Studies**: Distinguish between observational and experimental studies, noting that the latter allows for cause-and-effect conclusions.
- π **Sampling Techniques**: Be familiar with simple random, stratified random, cluster, and systematic random sampling methods.
- π― **Selecting a Sample**: Understand how to perform a simple random sample by numbering, random selection, and matching subjects to numbers.
- π’ **Stratification Purpose**: Stratify to ensure representation of important variables in the population for a more accurate sample statistic.
- π« **Avoiding Bias**: Be aware of selection and survey bias to ensure a representative sample that reflects the population.
- π§© **Principles of Experiment Design**: Know the four pillars of good experimental design: treatments, randomization, replication, and direct control.
- π **Importance of Randomization**: Use randomness to create diversity and representation in samples and experiments, which helps estimate population parameters accurately.
- π§ **Experimental Designs**: Recognize the three main experimental designs: completely randomized, randomized block, and matched pair design.
- βοΈ **Generalizing Results**: Know when and to whom you can generalize the results of a study, based on the sample or experimental setup.
- π **Study Tools**: Utilize practice questions, study guides, and review materials to prepare for AP Statistics exams effectively.
Q & A
What is the ultimate goal of collecting data in statistics?
-The ultimate goal of collecting data in statistics is to estimate a parameter from a population. This parameter is something that is true or summarizes something about the population. If a census is not feasible, a sample is taken, and a statistic is derived from this sample to approximate the population parameter.
What is the difference between an observational study and an experimental study?
-In an observational study, researchers simply observe and gather information without imposing any treatments on the subjects. In contrast, an experimental study involves imposing treatments on subjects to determine their effect on a response variable. Observational studies can only suggest associations between variables, while experiments can provide evidence for cause-and-effect relationships.
What are the four main sampling techniques mentioned in the script?
-The four main sampling techniques mentioned are simple random sample, stratified random sample, cluster random sample, and systematic random sample. Each technique has its own method of selecting samples to ensure representativeness and reduce bias.
Why is it important to perform a simple random sample?
-Performing a simple random sample is important because it helps to ensure that every member of the population has an equal chance of being selected. This reduces selection bias and increases the likelihood that the sample will be representative of the population.
What is stratification and why is it used in sampling?
-Stratification is the process of dividing a population into subgroups, or strata, based on specific characteristics before sampling. It is used to ensure that these subgroups are represented in the sample, which helps to make the sample more representative of the population and reduces variation between the sample statistic and the population parameter.
What are the two main types of bias that can affect a sample statistic?
-The two main types of bias are selection bias and survey bias. Selection bias occurs when the process of selecting the sample is not random or representative, while survey bias can occur due to issues with the survey itself, such as question wording, response bias, or non-response bias.
What are the four pillars of good experimental design?
-The four pillars of good experimental design are treatments, randomization, replication, and direct control. Treatments involve having two or more different treatments for comparison. Randomization ensures that subjects are assigned to treatments randomly to control for confounding variables. Replication involves repeating the experiment or having a sufficient number of subjects to ensure reliability. Direct control involves managing variables that could affect the outcome, such as ensuring all plants receive the same amount of water and sunlight.
Why is randomness important in selecting a sample and assigning subjects to treatments in an experiment?
-Randomness is important because it helps to create a mix that is representative of the population. It ensures diversity and representation, which are key to reducing variation and increasing the likelihood that the sample or experimental groups reflect the broader population.
What are the three different experimental designs?
-The three different experimental designs are completely randomized design, randomized block design, and matched pair design. The completely randomized design randomly assigns subjects to treatment groups. The randomized block design ensures representation of important variables by grouping subjects before random assignment. The matched pair design pairs similar subjects and then randomly assigns one of each pair to a different treatment.
When can you generalize the results of a study to a larger population?
-You can generalize the results of a study to a larger population if the subjects were randomly selected from that population and then randomly assigned to treatment groups. This double layer of randomness allows for the inference of cause-and-effect relationships and the generalization of results to the broader population.
What is the importance of understanding who you can infer or generalize your results to in a study?
-Understanding who you can infer or generalize results to is important because it determines the scope and applicability of your findings. If the sample or experimental subjects were not randomly selected from a specific population, the results may only be applicable to a similar group rather than the entire population.
Outlines
π Understanding Data Collection Goals and Variability
The first paragraph emphasizes the importance of knowing the goal of collecting data, which is to estimate a population parameter using a sample statistic. It discusses the challenge of achieving a perfect match due to sampling variability and the need to reduce this variation by ensuring unbiased, random, and representative samples. The example of estimating the proportion of people with brown hair in a population is used to illustrate this concept.
π Distinguishing Between Observational and Experimental Studies
This section differentiates between observational and experimental studies. In observational studies, researchers only observe and gather data without influencing the outcome. In contrast, experimental studies involve imposing treatments to measure their effects on a response variable. It also touches on the limitations of observational studies in establishing causation, whereas well-designed experiments can provide stronger evidence for cause-and-effect relationships.
π Knowledge of Sampling Techniques
The third paragraph covers various sampling techniques, including simple random sampling, stratified random sampling, cluster sampling, and systematic random sampling. It stresses the importance of recognizing these methods, as they are crucial for ensuring that the sample is representative of the population. Each technique is briefly explained, highlighting when and why each might be chosen.
π― Selecting a Simple Random Sample
The fourth tip focuses on the process of selecting a simple random sample, which is a fundamental technique used across different sampling methods and experimental designs. The steps involve assigning unique numbers to every subject, using a random number generator or table, and ensuring no repeats to select the sample. This process is essential for maintaining randomness and representativeness in a sample.
π§ͺ Stratification for Improved Sample Representation
Stratification is the process of dividing a population into subgroups, or strata, based on a relevant variable to ensure representation of each group in the sample. This paragraph explains that stratification helps to reduce variation between the sample and the population parameters, making the sample more reflective of the population's diversity. It also advises on stratifying based on variables that are pertinent to the research question.
π« Avoiding Bias in Data Collection
Bias can significantly impact the accuracy of sample statistics. This section outlines two main types of bias: selection bias, which occurs during the sampling process, and survey bias, which relates to the survey's design or the way questions are asked. It also covers specific forms of survey bias, including response bias and non-response bias, and the importance of obtaining responses from a diverse sample to ensure the sample's representativeness.
ποΈ Fundamentals of Good Experimental Design
The seventh paragraph outlines the four key elements of a well-designed experiment: treatments, randomization, replication, and direct control. Treatments involve comparing two or more conditions. Randomization ensures that treatments are assigned to subjects in a way that controls for unmeasured variables. Replication, both within and across experiments, strengthens the validity of results. Direct control involves managing variables that could affect the outcome.
π The Importance of Randomization in Experiments
Randomization is highlighted as a critical component in both sampling and experimental design due to its ability to create a mix that reflects the diversity and representation of the population. It helps in ensuring that any differences observed between groups in an experiment can be attributed to the treatments rather than other variables.
π§ Different Types of Experimental Designs
This section introduces three experimental designs: completely randomized, randomized block, and matched pair design. Each design is suited to different scenarios and helps in controlling for various variables that could affect the outcome. The completely randomized design randomly assigns treatments to subjects, the randomized block design ensures representation of a critical variable across treatment groups, and the matched pair design pairs similar subjects to control for individual differences.
π Generalizing Results from Samples and Experiments
The final paragraph discusses the conditions under which results from a sample or an experiment can be generalized to a larger population. It emphasizes the necessity of random selection in both the sampling process and the assignment of subjects to treatment groups in an experiment. The double layer of randomness allows for the inference of cause-and-effect relationships and the generalization of results to the broader population from which the sample was drawn.
Mindmap
Keywords
π‘Collecting Data
π‘Sampling Variability
π‘Observational Study
π‘Experimental Study
π‘Sampling Techniques
π‘Stratification
π‘Bias
π‘Experimental Design
π‘Randomization
π‘Replication
π‘Generalization
Highlights
The ultimate goal of collecting data is to estimate a population parameter using a sample statistic.
Reducing sampling variability is crucial for the sample statistic to closely approximate the population parameter.
Differentiating between observational and experimental studies is key, with the latter allowing for cause-and-effect conclusions.
Understanding the four sampling techniques: simple random sample, stratified random sample, cluster random sample, and systematic random sample.
The importance of randomization in sampling to ensure a mix that represents the population.
Stratification ensures representation of important variables within the sample, aiding in the accuracy of the sample statistic.
Bias, including selection and survey bias, can significantly affect the accuracy of a sample statistic.
The four pillars of good experimental design: treatments, randomization, replication, and direct control.
Randomization in experiments helps control for extraneous variables and ensures group similarity.
Three types of experimental designs: completely randomized, randomized block, and matched pair.
Randomized selection and assignment in experiments allow for cause-and-effect inferences and generalization to larger populations.
The necessity of understanding to whom results can be generalized, which depends on the randomness of sample selection and treatment assignment.
The importance of using a random number table or generator for unbiased simple random sampling.
Stratified sampling ensures diversity within the sample by first categorizing the population into strata.
Cluster sampling involves selecting entire groups or clusters as the sample, assuming each cluster is a mini-population.
Systematic sampling can introduce bias if not done correctly, as it involves selecting every nth element from a list.
The potential for non-response bias when a portion of the sample chosen does not respond, which may skew results.
Transcripts
Browse More Related Video
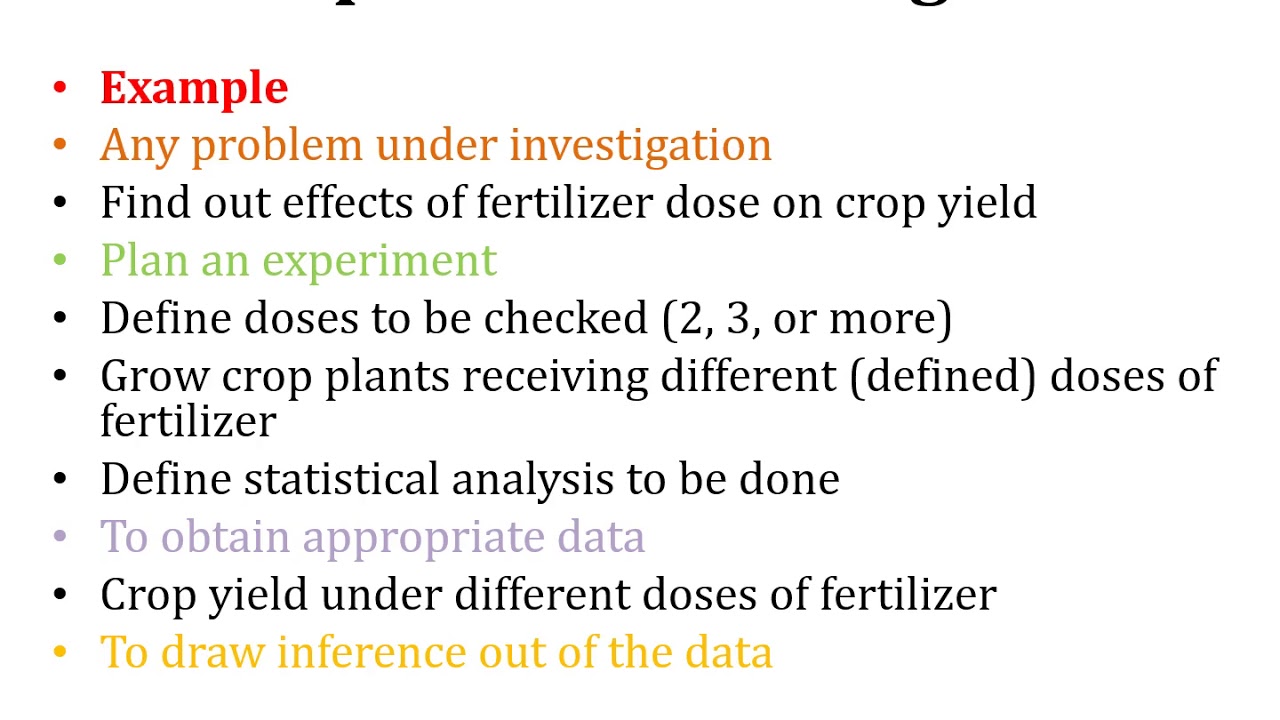
Introduction to Experimental Designs; Principles; Randomization; Replication; Local Control
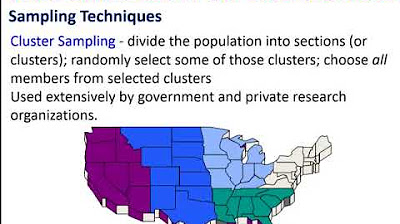
Elementary Statistics - Chapter 1 Introduction to Statistics Part 2
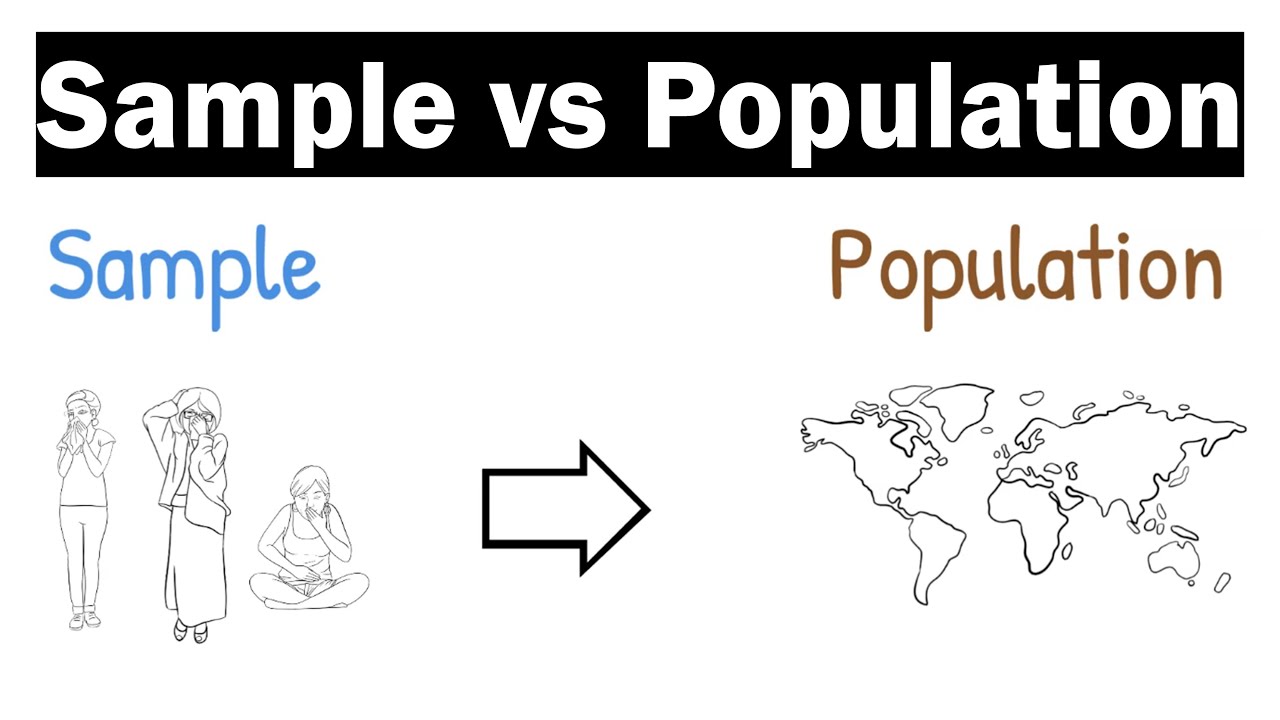
Sample vs Population - Clearly Explained

Introductory Statistics Lecture 1 Introduction and Chapter 1 Part 1
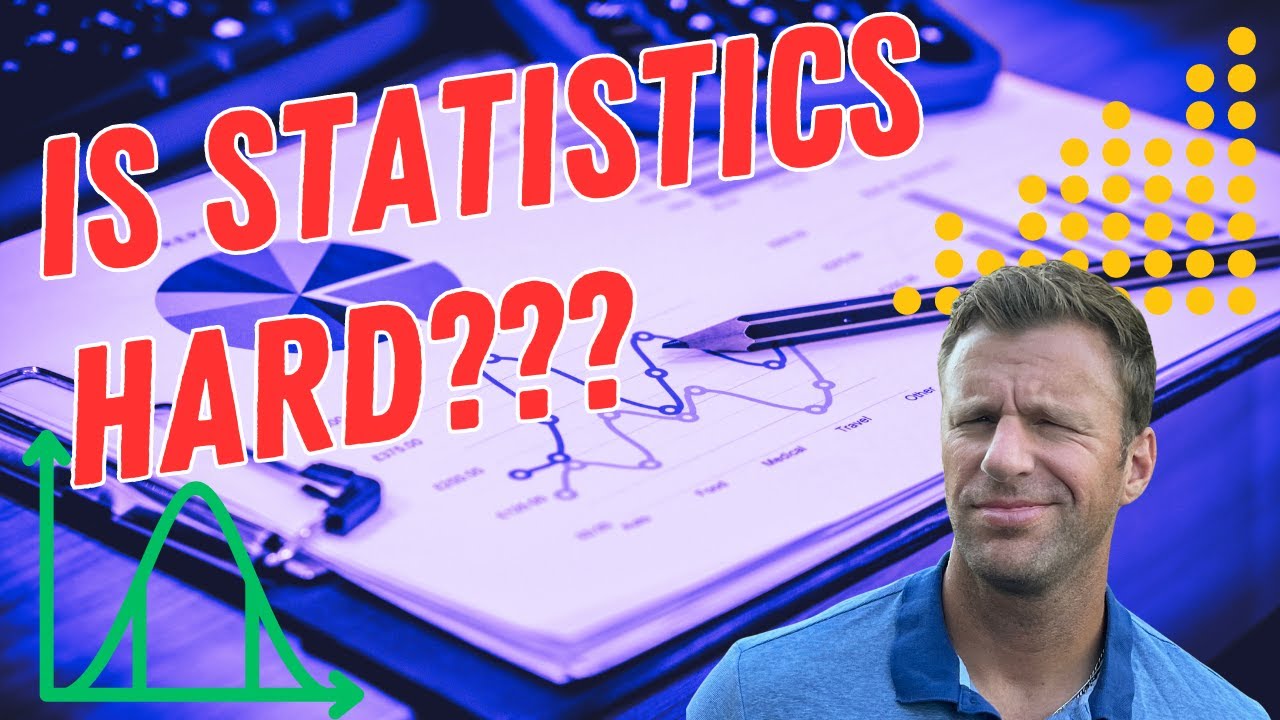
Is STATISTICS hard?
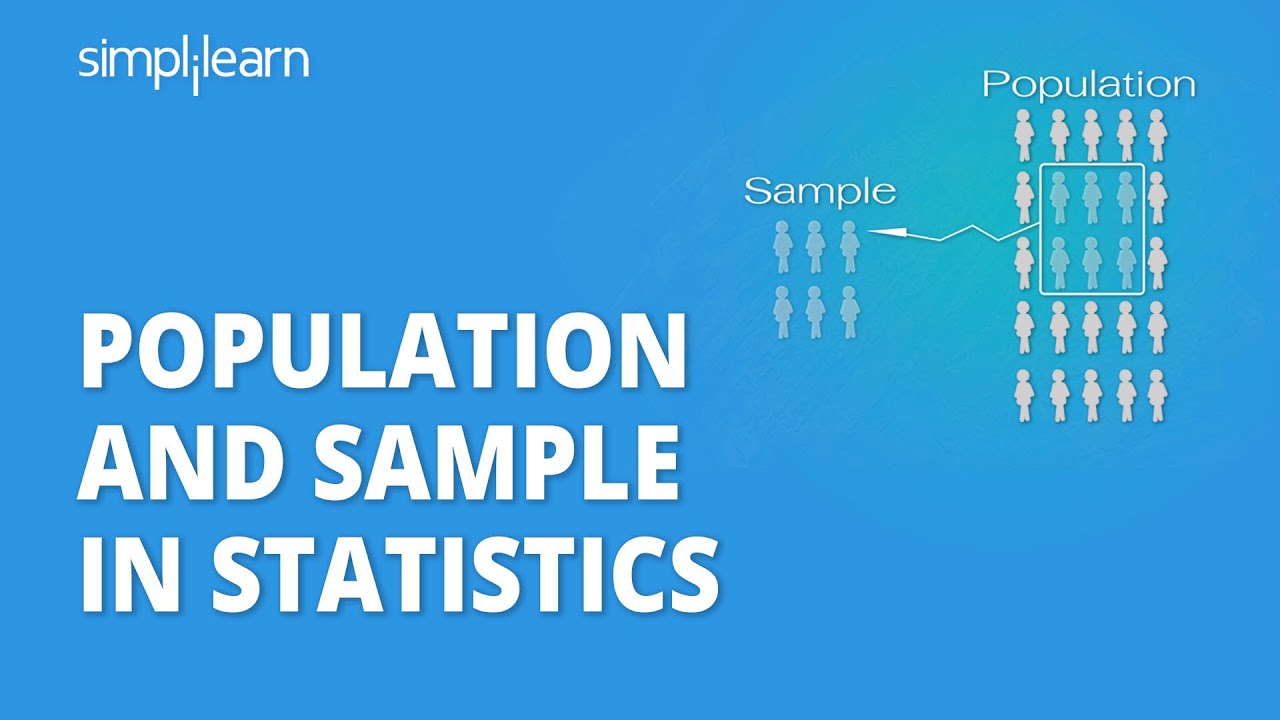
Population And Sample In Statistics Example | Population vs Sample In Statistics | Simplilearn
5.0 / 5 (0 votes)
Thanks for rating: