Measures of Variability (Range, Standard Deviation, Variance)
TLDRThe video script delves into the concept of measures of variability, a crucial aspect of descriptive statistics that complements measures of central tendency. It emphasizes the importance of understanding data distribution by illustrating the limitations of mean as a sole measure. The video introduces range, standard deviation, and variance as key measures of variability, explaining their significance in interpreting data sets. It also highlights how standard deviation provides valuable insights in normally distributed data, such as IQ scores, by indicating what percentage of data falls within specific ranges from the mean. The script sets the stage for future lessons on calculating these statistical measures.
Takeaways
- π Measures of variability are essential in descriptive statistics to capture the dispersion of data points in addition to central tendency.
- π The mean alone can be misleading; it's important to consider both central tendency and variability for a complete data analysis.
- π Two datasets with the same mean can have vastly different variability, which can significantly impact interpretations and decisions based on the data.
- π’ The range is a simple measure of variability calculated as the highest score minus the lowest score in a dataset.
- π The range can quickly provide a basic understanding of data spread, but it might not fully represent the data's distribution.
- π In practical scenarios, like pharmaceutical research, understanding variability can help make more informed decisions between options with similar means but different consistency.
- π Standard deviation is a measure that describes the average amount of deviation from the mean, providing insight into the spread of data points.
- π In a normal distribution, standard deviations help identify common and uncommon data points, such as how many people fall within certain ranges from the mean.
- π’ Variance is the square of the standard deviation, representing the average squared deviation from the mean.
- π Symbols differ for population and sample standard deviations (Ξ£ for population, s for sample), and the same applies to variance (Σ² for population, sΒ² for sample).
- π― The next step is learning how to calculate these measures of variability, which will be covered in subsequent educational content.
Q & A
What is the main topic of the video?
-The main topic of the video is measures of variability, a form of descriptive statistics that helps describe the differences among data points in a dataset.
Why is it important to understand measures of variability in addition to measures of central tendency?
-Understanding measures of variability is important because it provides a more complete picture of the data, capturing aspects such as how spread out or clustered together the points are, which a measure of central tendency like the mean cannot alone.
What are the two examples used in the video to illustrate the need for measures of variability?
-The two examples are: 1) Comparing two datasets with the same mean but different spreads, and 2) Deciding between two medications for depression based on their effectiveness and consistency of improvement.
What are the three measures of variability discussed in the video?
-The three measures of variability discussed are the range, standard deviation, and variance.
How is the range calculated and what is its limitation?
-The range is calculated by subtracting the lowest score (L) from the highest score (H) in a dataset. Its limitation is that it can sometimes miss or not fully represent the information in the dataset, as it only considers the extreme values.
What does standard deviation describe and why is it useful?
-Standard deviation describes the standard or typical amount that scores deviate from the mean. It is useful because it provides additional information about a dataset, such as the proportion of data points within a certain range from the mean, especially in a normally distributed set of data.
What percentage of data falls within one, two, and three standard deviations from the mean in a normal distribution?
-In a normal distribution, approximately 68% of data falls within one standard deviation, 95% within two standard deviations, and 99.7% within three standard deviations from the mean.
How is variance related to standard deviation?
-Variance is related to standard deviation as it is the square of the standard deviation. It represents the average squared deviation from the mean.
What are the symbols used to represent population standard deviation and sample standard deviation?
-The symbol for population standard deviation is Sigma (Ξ£), while the symbol for sample standard deviation is s.
How is the variance for a population denoted and what is the difference for a sample?
-The variance for a population is denoted as Sigma squared (Ξ£^2), and for a sample, it is denoted as s^2.
What is the term used for the sum of squares in the formulas for standard deviation and variance?
-The term used for the sum of squares in the formulas for standard deviation and variance is 'sums of squares', often abbreviated as SS.
Outlines
π Understanding Measures of Variability
This paragraph introduces the concept of measures of variability, a type of descriptive statistics that complements measures of central tendency. It emphasizes the importance of understanding variability in data through two examples. The first example compares two datasets with the same mean but different spreads, illustrating how the mean alone can be misleading. The second example discusses decision-making in a pharmaceutical context, highlighting the value of knowing variability in addition to the mean. The paragraph outlines three measures of variability to be discussed: range, standard deviation, and variance, starting with the range for its simplicity and quick calculation.
π Exploring Standard Deviation and Variance
This paragraph delves into the significance of standard deviation in understanding data distribution, particularly in a normal curve. It explains how standard deviation quantifies the average amount of deviation from the mean, providing insights into what is common and uncommon within a dataset. The paragraph uses the example of IQ scores to demonstrate how knowing the standard deviation can inform us about the proportion of data falling within certain ranges. It also introduces variance as the square of the standard deviation, which represents the average squared deviation from the mean. The paragraph concludes with a brief mention of the formulas for calculating standard deviation and variance for both population and sample data.
Mindmap
Keywords
π‘Measures of Variability
π‘Descriptive Statistics
π‘Mean
π‘Range
π‘Standard Deviation
π‘Variance
π‘Pharmaceutical Company
π‘Normal Distribution
π‘IQ Scores
π‘Sum of Squares
Highlights
Measures of variability are essential in descriptive statistics to complement measures of central tendency.
Two datasets with the same mean can have vastly different situations due to different levels of variability.
The range is a simple measure of variability that calculates the difference between the highest and lowest scores in a dataset.
The range can be quickly calculated but may not fully capture the distribution of a dataset, potentially missing important information.
Standard deviation describes the typical amount that scores deviate from the mean, providing a more detailed understanding of data distribution.
In a normal distribution, standard deviations help identify what is common and uncommon, with 68% of data falling within one standard deviation and 95% within two.
Variance is the square of the standard deviation, representing the average squared deviation from the mean.
Population standard deviation is denoted by Sigma, while sample standard deviation is denoted by s.
For population variance, it is represented as Sigma squared, and for sample variance, it is represented as s squared.
The video emphasizes the importance of understanding both the mean and measures of variability to get a comprehensive picture of a dataset.
In practical applications, such as pharmaceuticals, knowing the variability can help make better decisions, as illustrated by the example of choosing between two medications.
The video provides a clear example of how the range alone might be misleading, as it does not account for the shape of the data distribution.
Standard deviations are particularly useful in understanding the distribution of data that follows a normal curve, such as height and weight.
The video introduces the concept that in a normal distribution, 99.7% of data falls within three standard deviations of the mean.
The video explains the significance of knowing standard deviations in interpreting data, such as IQ scores, and what different standard deviation ranges indicate about the population.
The video outlines the formulas for standard deviation and variance, highlighting the sums of squares (SS) as a key component.
The video emphasizes the difference in formulas between population and sample statistics, noting that the numerator is the sums of squares.
The video concludes by highlighting the importance of understanding both the mean and measures of variability for a complete data analysis.
Transcripts
Browse More Related Video

Range, variance and standard deviation as measures of dispersion | Khan Academy
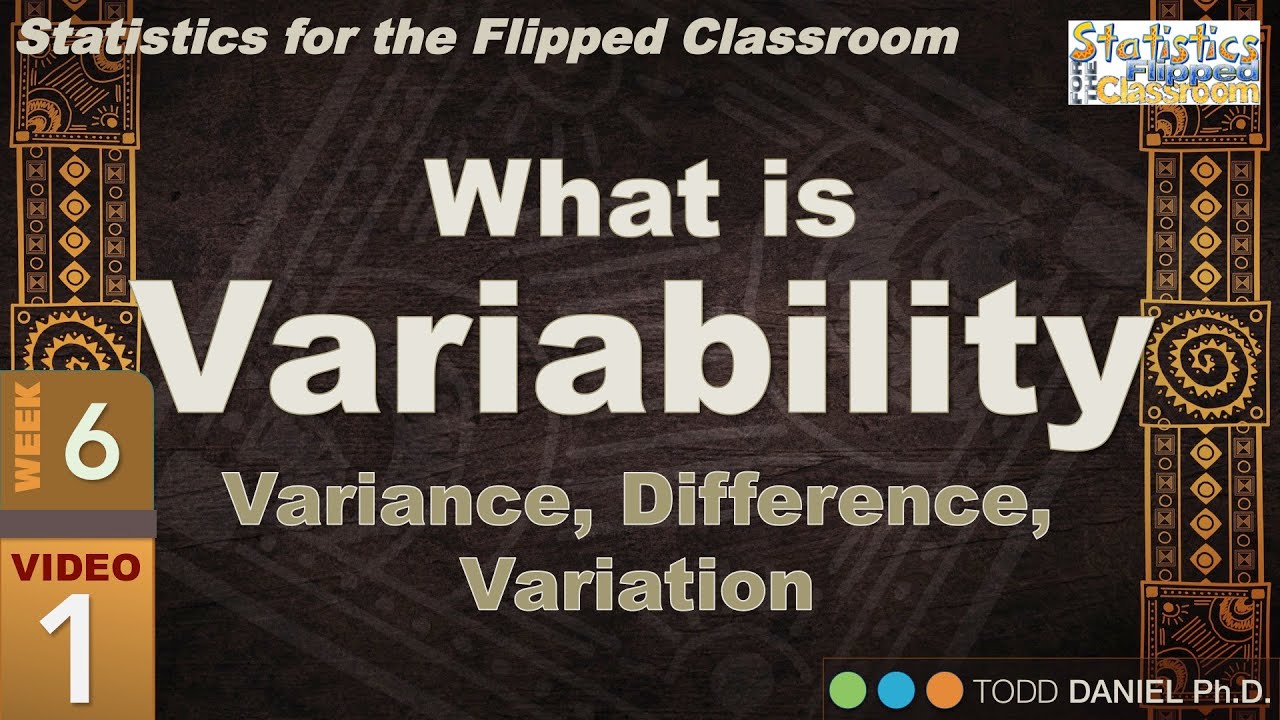
What is Variability? β An Introduction to Variance in Statistics (6-1)
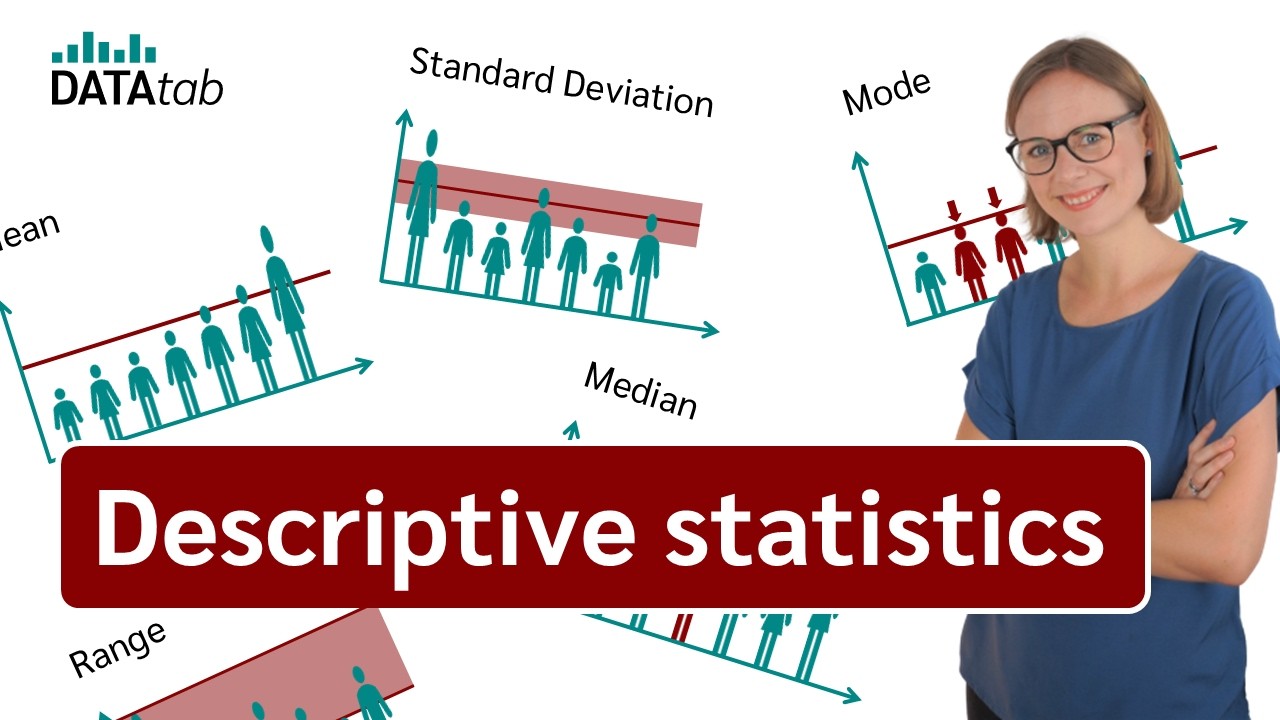
Descriptive Statistics [Simply explained]
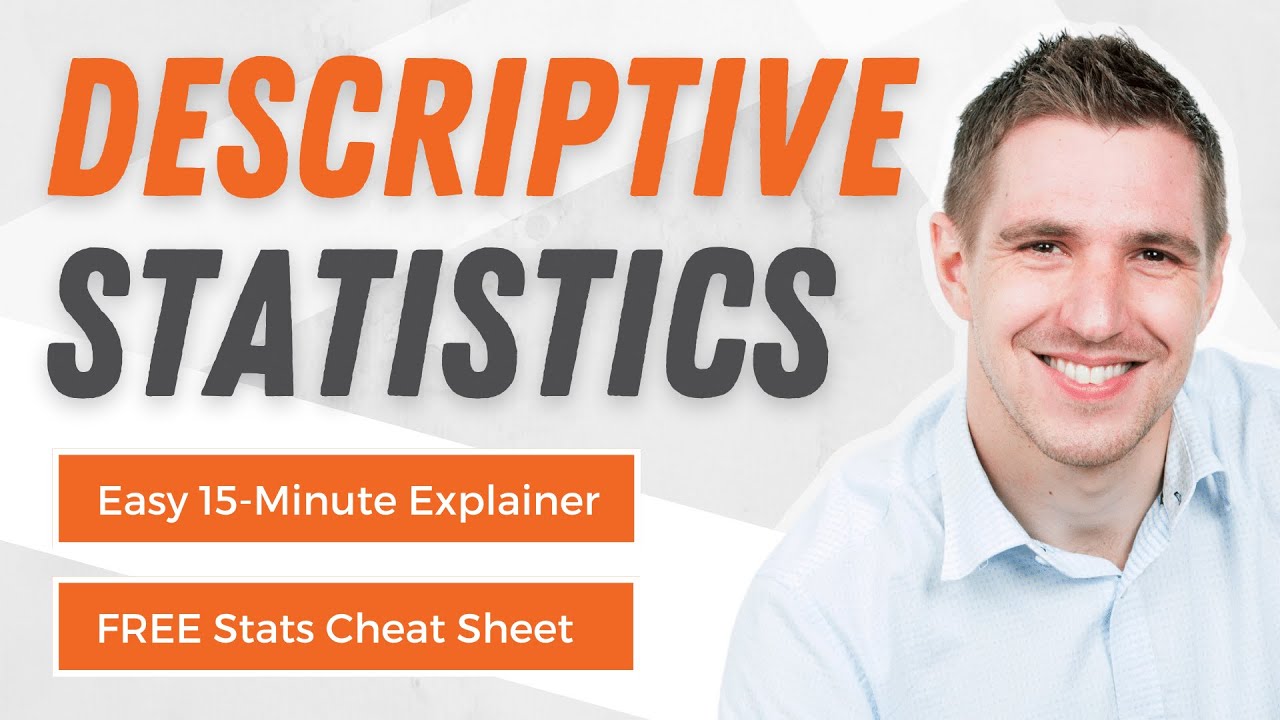
Descriptive Statistics: FULL Tutorial - Mean, Median, Mode, Variance & SD (With Examples)

Elementary Stats Lesson #3 A

What is Descriptive Statistics? A Beginner's Guide to Descriptive Statistics!
5.0 / 5 (0 votes)
Thanks for rating: