Crack Metric/Business Case/Product Sense Problems for Data Scientists | Data Science Interviews
TLDREmma's video offers a comprehensive guide to tackling product sense problems in data science interviews. She shares frameworks for diagnosing issues, measuring success, and deciding whether to launch a product. With insights from her own experiences at Airbnb, Lyft, and Twitter, Emma emphasizes the importance of structure, comprehensiveness, and feasibility in responses. Her tips for interview preparation include researching the company, clarifying questions, and demonstrating a systematic approach to problem-solving.
Takeaways
- π Emma discusses strategies for tackling product sense problems in data science interviews, which are often challenging for candidates without product experience.
- π‘ She emphasizes the importance of having a structured approach to answer these questions, which can be developed through practice and study.
- π Emma shares frameworks that have helped her secure job offers from companies like Airbnb, Lyft, and Twitter, and have also been proven effective for her friends.
- π Product sense problems are asked to evaluate a candidate's ability to define metrics and solve product-related issues, which are critical skills for a data scientist.
- π The first category of problems involves diagnosing issues when a key metric shifts negatively, requiring a systematic approach to identify root causes.
- π The second category focuses on measuring the success or health of a product or feature, where candidates should define relevant metrics and a guardrail metric.
- π The third category, 'launch or not,' involves testing product ideas or deciding whether to launch a feature, requiring a clear experimental design and recommendation based on results.
- π Emma suggests starting with clarifying questions to understand the product's function and goal before defining success metrics.
- π She highlights the importance of context in choosing metrics, as a metric suitable for one scenario may not be appropriate for another.
- βοΈ During interviews, candidates should clarify the question, listen to the interviewer's feedback, and structure their thought process visibly, such as through bullet points.
- π Researching the company and its products before the interview can lead to deeper insights and better interview conversations.
Q & A
What is the main topic of the video that Emma is discussing?
-The main topic is about how to crack product sense problems for data science interviews, which are often challenging for candidates without direct product experience.
Why are product sense problems asked during data science interviews?
-Product sense problems are asked to evaluate a candidate's ability to define the right metrics to measure product success, diagnose and solve real product problems, and assess their problem-solving skills.
What are the three most commonly asked categories of product sense questions according to Emma?
-The three categories are diagnosing a problem, measuring success, and deciding whether to launch a product or feature.
What does Emma suggest for someone who lacks experience in product-related work to do in order to prepare for product sense questions?
-Emma suggests gaining knowledge through reading, listening, thinking, and summarizing, which can help develop the skill of answering product sense questions even without prior experience.
What are the three key elements that constitute a good answer to product sense questions according to the script?
-A good answer should have a structured approach, be comprehensive in covering all important aspects of the problem, and be feasible, meaning the solution should be practical and realistically implementable.
Can you provide an example of a product sense problem related to diagnosing a problem as mentioned in the script?
-An example given is a 20% decrease in the creation of Facebook user groups, where the candidate is asked to figure out the root cause of this issue.
What is Emma's framework for answering product sense questions about diagnosing a problem?
-Emma's framework involves six steps: clarifying the scenario and metric, analyzing the time aspect of the change, considering internal and external factors, checking for changes in related products or features, segmenting by user demographics and behavior, decomposing the metric for further analysis, and summarizing the approach.
How should one approach measuring the success of a product or feature according to the script?
-One should start by asking clarifying questions to understand the product's function and goal, then define relevant metrics, including a guardrail metric that should not degrade, and ensure the metrics fit the context of the product or feature.
What is the significance of guardrail metrics in the context of product sense questions?
-Guardrail metrics are important because they are indicators that should not worsen while pursuing the success of a new product or feature, helping to maintain a balanced view of product health.
How does Emma suggest approaching questions about whether to launch a product or feature?
-Emma suggests designing an experiment to test the product or feature, considering how to split users into control and treatment groups, determining the duration of the experiment, and making recommendations based on the results, linking them to the initial goal and business impact.
What are some tips Emma provides for acing a product sense interview?
-Emma advises researching the company and its products beforehand, clarifying the question before answering, listening to the interviewer's feedback, and structuring the thought process clearly during the interview.
Outlines
π Introduction to Product Sense in Data Science Interviews
Emma introduces the video's focus on tackling product sense problems in data science interviews, a common challenge for those without direct product experience. She promises to share frameworks that have helped her and others secure jobs at top companies like Airbnb, Lyft, and Twitter. The video will cover understanding product sense problems, addressing three common question categories, and tips for acing the interview. Emma emphasizes that product sense is a skill that can be developed through practice and learning.
π Systematic Approach to Diagnosing Product Problems
The second paragraph delves into the first category of product sense questions: diagnosing problems. Emma outlines a six-step framework to systematically identify the root cause of negative metric shifts. This includes clarifying scenario definitions, analyzing time aspects of changes, considering internal and external factors, examining changes in related products, segmenting by user demographics, and decomposing metrics for in-depth analysis. She advises summarizing the approach and suggesting potential causes and solutions to impress interviewers.
π Measuring Success: Defining the Right Metrics
In the third paragraph, Emma discusses the second category: measuring success. She advises starting with clarifying questions to understand the product's function and goal. Then, she recommends defining no more than three key metrics, including a guardrail metric to ensure no degradation in other areas. The importance of context-appropriate metrics is highlighted, using a job recommendation algorithm example to illustrate the point.
π Launch Decisions: Testing Product Ideas and Impact Analysis
Emma addresses the third category of questions in the fourth paragraph, which involves deciding whether to launch a product or feature. She suggests designing experiments, including random userεη», and determining the duration of the test. The focus is on linking results to the initial goal and business impact, considering both short-term and long-term effects. She also discusses how to handle complicating results and the importance of translating them into user and business impact.
π Interview Tips for Demonstrating Product Sense
In the final paragraph, Emma shares tips for acing product sense interviews. She emphasizes the importance of researching the company and its products, clarifying questions, listening to the interviewer, and structuring responses with bullet points. The goal is to showcase a clear and systematic approach to problem-solving, which can lead to a successful interview outcome.
Mindmap
Keywords
π‘Product Sense
π‘Data Science Interviews
π‘Frameworks
π‘Diagnose a Problem
π‘Measure Success
π‘Launch or Not
π‘Metrics
π‘Problem-Solving Skills
π‘Structured Approach
π‘Interview Preparation
π‘Guardrail Metrics
Highlights
Emma shares frameworks for answering product sense questions in data science interviews, which helped her get job offers from Airbnb, Lyft, and Twitter.
Product sense problems are often difficult for candidates without experience in product roles, but can be developed through practice.
A good answer to a product sense question should be structured, comprehensive, and feasible.
Product sense questions assess a candidate's ability to define metrics, diagnose problems, and solve real product issues.
Emma's frameworks have been proven effective, helping friends get their dream data science jobs.
The first category of product sense questions is diagnosing a problem, where candidates must identify the root cause of a negative metric shift.
Emma's framework for diagnosing problems includes clarifying the scenario, analyzing time aspects, internal factors, external factors, related products, user demographics, and decomposing the metric.
The second category is measuring success, where candidates must define appropriate metrics for a product or feature.
When measuring success, it's important to provide no more than three key metrics, including one guardrail metric that should not degrade.
Context is crucial when defining success metrics, as what works in one scenario may not be suitable in another.
The third category, 'launch or not', involves testing product ideas or deciding whether to launch a feature based on experimental results.
For 'launch or not' questions, candidates should design experiments, make recommendations based on results, and consider both short-term and long-term impacts.
Emma provides tips for acing product sense interviews, including researching the company, clarifying questions, listening to the interviewer, and structuring thought processes.
Candidates should familiarize themselves with a company's products and consider how to improve them and measure their success before interviews.
During interviews, it's important to clarify the high-level goal, listen to feedback, and structure responses to show a systematic approach.
Emma's video offers a comprehensive guide to mastering product sense problems in data science interviews, with frameworks and tips for success.
Transcripts
Browse More Related Video
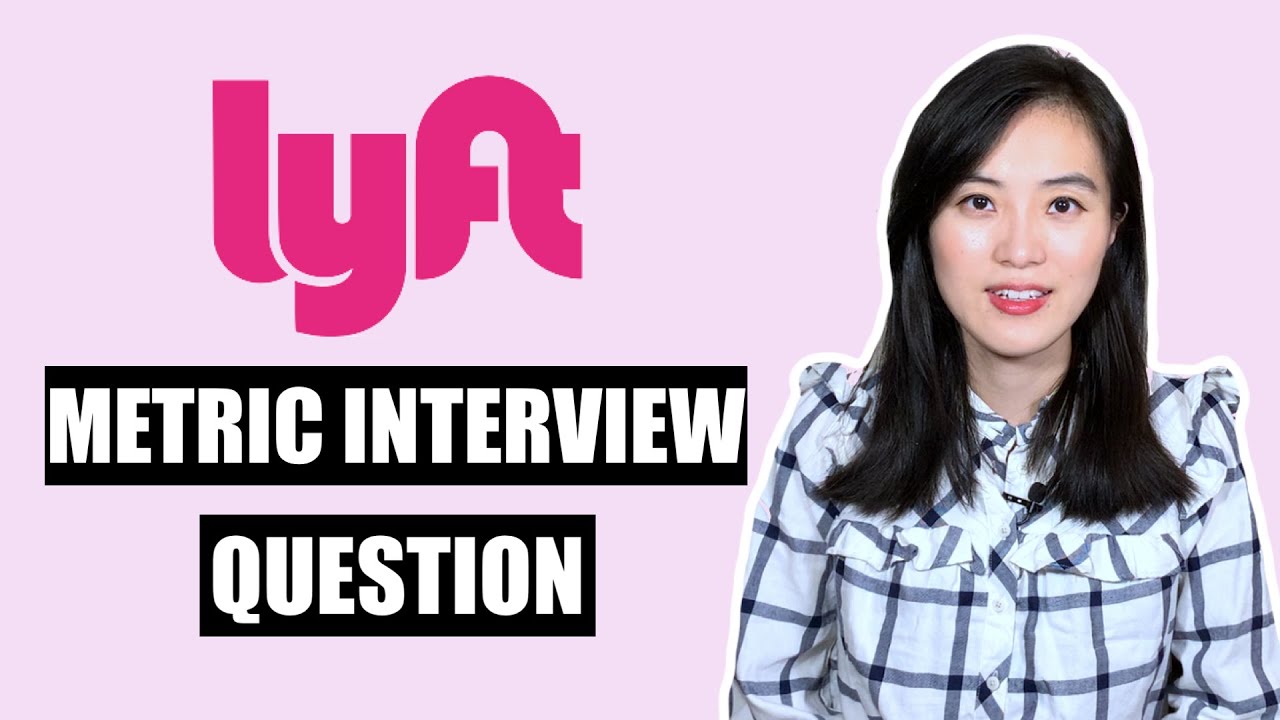
Lyft/Uber Metric Interview Question and Answer: Tips for Data Science Interview Success!
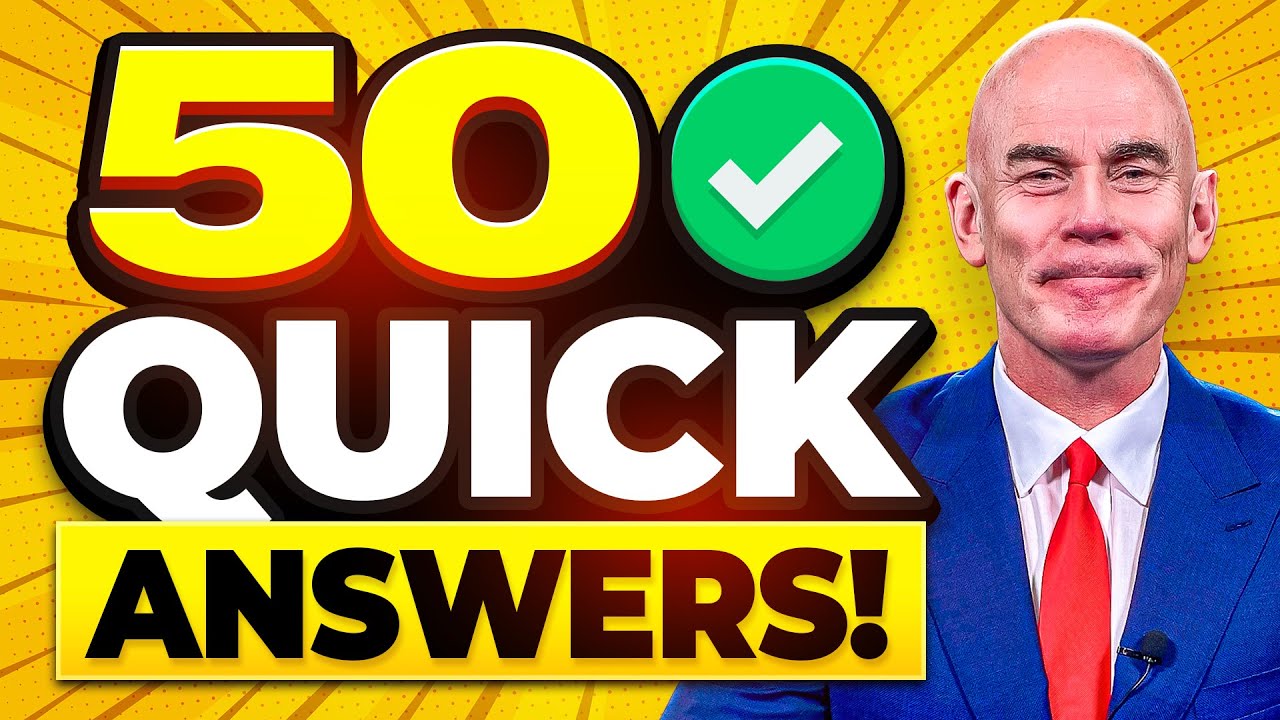
50 'QUICK ANSWERS' to TOUGH INTERVIEW QUESTIONS!
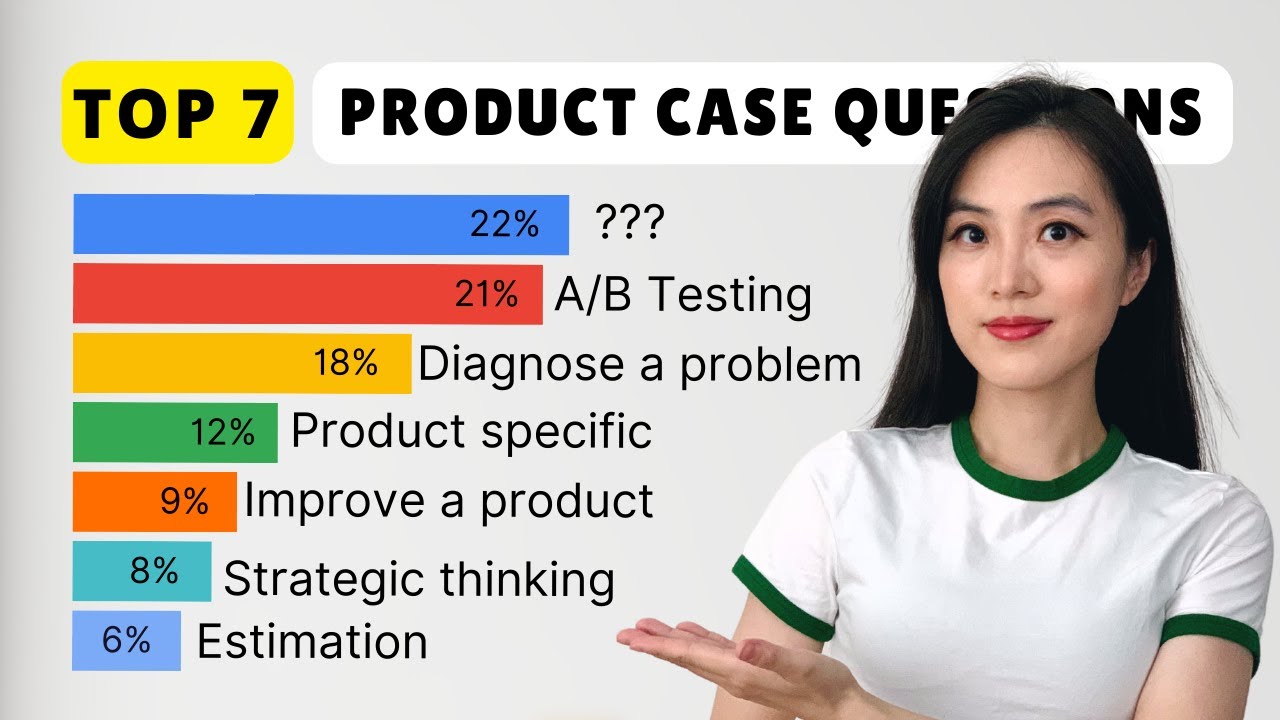
Ace Product/Business Case Interview Questions: A Data-driven Approach for Data Scientists
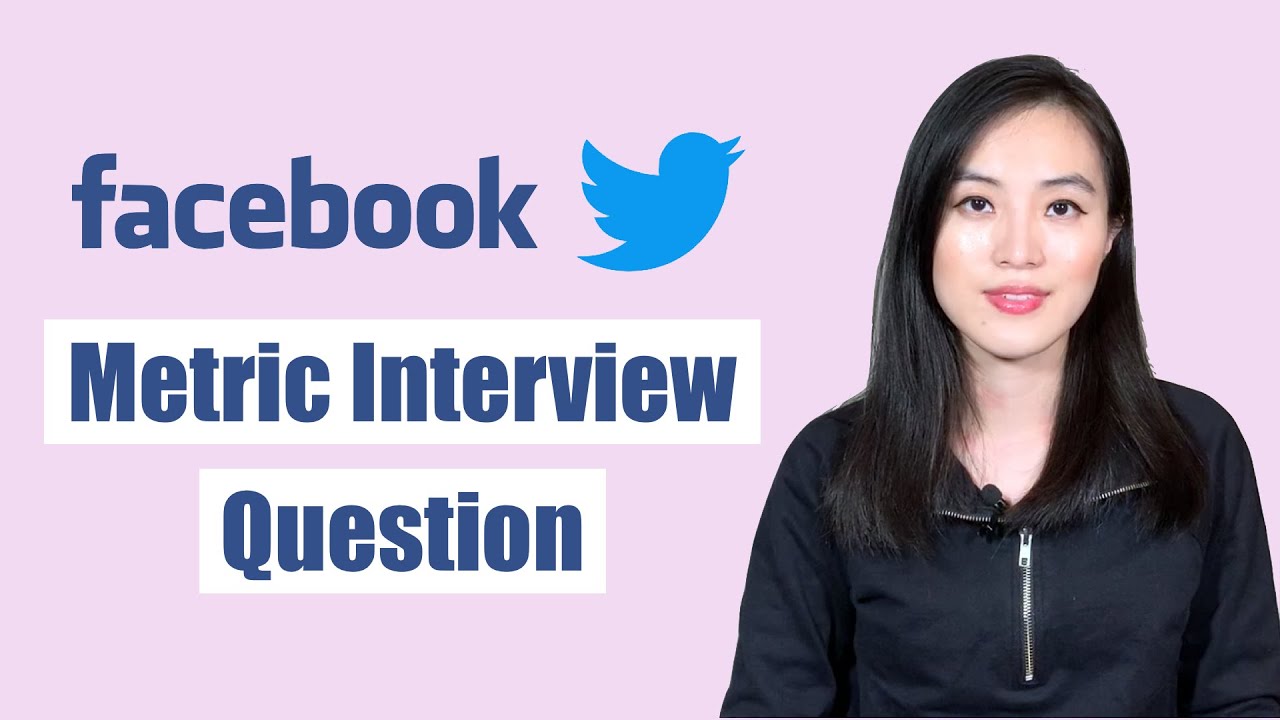
Cracking Facebook (Meta) Product Case Interviews: Tips for Data Science Interview Success!
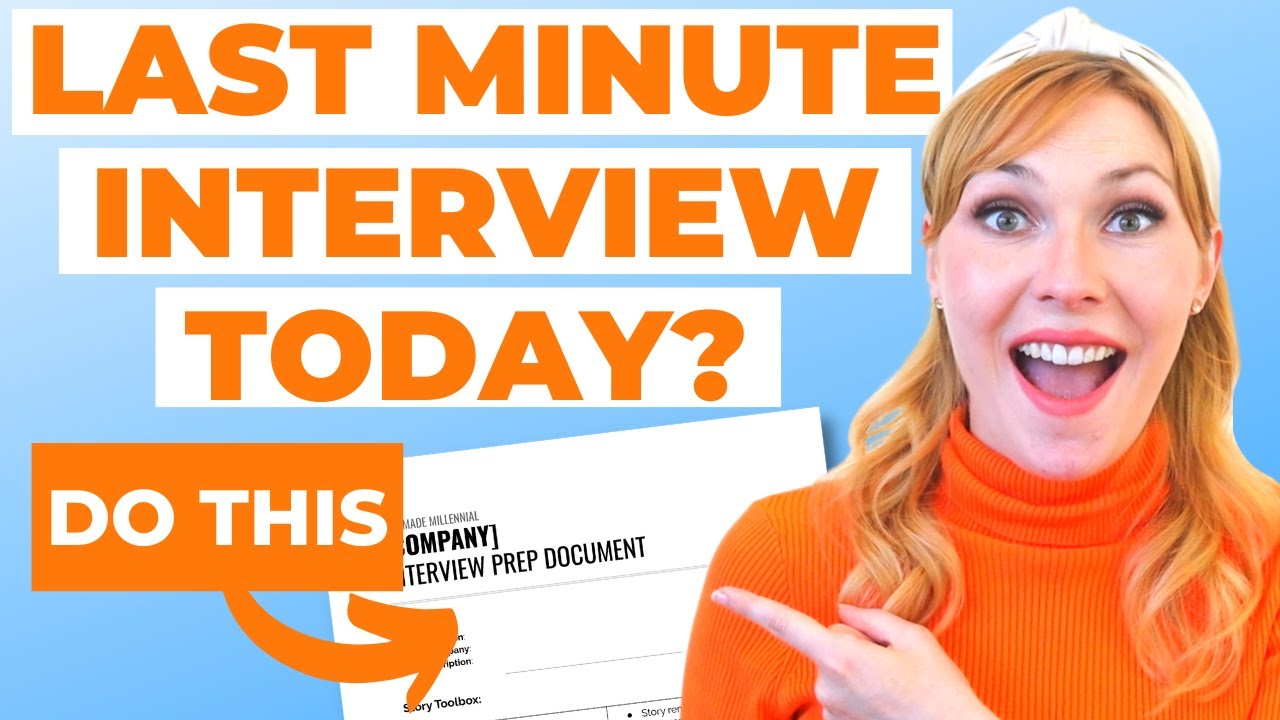
RUSHED Interview Prep - How to Prepare for a Job Interview at the LAST Minute!
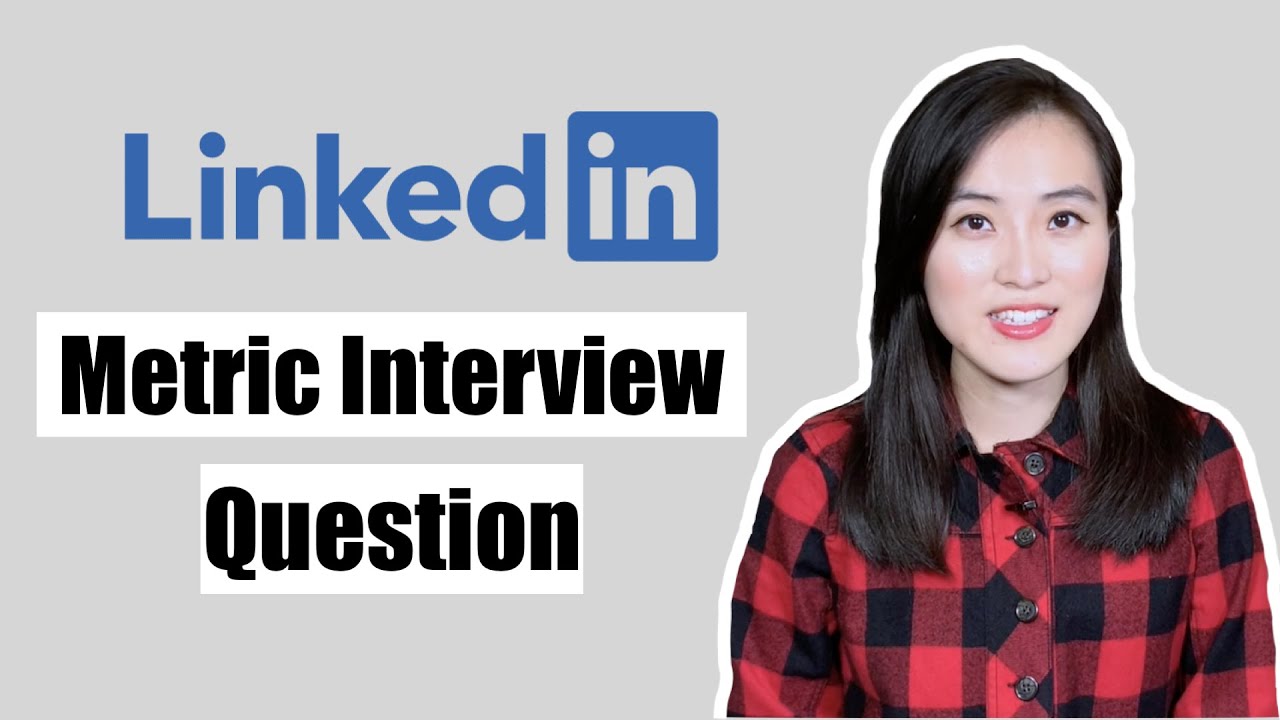
LinkedIn Metric Interview Question and Answer: Tips for Data Science Interview Success!
5.0 / 5 (0 votes)
Thanks for rating: