Lecture 4: Conditional Probability | Statistics 110
TLDRThe video script is a comprehensive lecture on probability theory, focusing on the concepts of inclusion-exclusion, independence, and conditional probability. The speaker begins by revisiting a matching problem involving a deck of cards, illustrating the inclusion-exclusion principle. They then delve into the definition of independent events, emphasizing the distinction between independence and disjointness, and provide a counterexample to highlight the importance of understanding the concept of independence fully. The lecturer also discusses the famous Newton-Pepys problem, a gambling question involving dice, which was solved by Isaac Newton and demonstrates the application of probability theory in solving real-world problems. The script concludes with an introduction to conditional probability, including Bayes' rule, and its significance in updating beliefs based on new evidence. The lecture is enriched with intuitive explanations, such as the 'pebble world' analogy, and the importance of the subject in various fields, including science and philosophy.
Takeaways
- ๐ The De Montmort matching problem is a classic example of the inclusion-exclusion principle in probability theory.
- ๐ The probability of the union of events can be calculated using the inclusion-exclusion principle, which sums individual probabilities and adjusts for overlaps.
- โ๏ธ Symmetry plays a crucial role in simplifying the calculation of probabilities, especially when all orders of a deck of cards are equally likely.
- ๐ข The concept of independence in probability means that the occurrence of one event does not affect the probability of another event.
- โ Disjoint events are different from independent events; disjoint events cannot occur at the same time, whereas independent events have no influence on each other's probability.
- ๐ฒ The Newton-Pepys problem from 1693 involves calculating the probability of rolling certain numbers with dice and is an example of how probability can be applied to gambling questions.
- ๐ค Intuition can be misleading when solving probability problems; formal calculation is necessary to arrive at the correct answer.
- ๐ The probability of no matches in a deck of cards converges to 1/e as the number of cards goes to infinity, which is a counterintuitive result for many.
- ๐ค The use of factorials in the bottom of a probability fraction often suggests the application of Taylor series, particularly the series for e^x.
- ๐งฉ Conditional probability is about updating our beliefs or probabilities in light of new evidence.
- ๐ค Independence implies that the probability of two events A and B occurring together is the product of their individual probabilities, P(A) * P(B).
- ๐ Bayes' rule is a fundamental theorem in probability that provides a way to find the probability of an event based on conditional probabilities and is foundational in Bayesian statistics.
Q & A
What is the De Montmort problem discussed in the script?
-The De Montmort problem is a classic example of a probability puzzle involving a matching problem where cards are flipped and the goal is to match the position of the card in the deck to its numerical value. The problem showcases the use of inclusion-exclusion principle to calculate the probability of at least one match occurring during the game.
What does inclusion-exclusion principle imply in the context of the De Montmort problem?
-In the De Montmort problem, the inclusion-exclusion principle is used to calculate the probability of the union of several events (matches occurring at specific positions in the deck). The principle works by alternatingly adding and subtracting probabilities of intersections of these events to account for overlaps and ensure each scenario is counted only once.
What does it mean when two events are independent in probability theory?
-In probability theory, two events are considered independent if the occurrence of one does not affect the probability of the occurrence of the other. Mathematically, this is expressed as the probability of the intersection of the events being equal to the product of their individual probabilities.
What is pairwise independence and why is it not sufficient for complete independence among three events?
-Pairwise independence refers to every pair of events being independent of each other. However, this does not imply that the three events are completely independent because it does not guarantee that the probability of all three events occurring together is the product of their individual probabilities. An additional condition needs to be met for complete independence among three events.
How does the Taylor series relate to approximating the probability in the De Montmort problem?
-In the De Montmort problem, the probability of no match (or all matches) resembles terms of a Taylor series for exponential functions. Recognizing this pattern allows for the use of the Taylor series for 'e to the x' to approximate the probability of no matches, which interestingly converges to about 1/e.
What was the Newton-Pepys problem and how was it resolved?
-The Newton-Pepys problem involved Samuel Pepys asking Isaac Newton to solve a gambling problem related to dice. The question was to determine which event is more likely: getting at least one six with six dice, at least two sixes with twelve dice, or at least three sixes with eighteen dice. Newton used probability calculations to prove that the first event was the most likely.
What is the significance of conditional probability in statistics?
-Conditional probability is fundamental in statistics as it deals with the probability of an event occurring given the occurrence of another event. It is crucial for updating beliefs in light of new evidence, making it a cornerstone for fields such as Bayesian statistics, which heavily relies on updating probabilities as new data becomes available.
How is Bayes' Rule derived and what are its implications?
-Bayes' Rule is derived by manipulating the formula for conditional probability. It relates the conditional probabilities of two events by dividing the probability of one event occurring given the other by the probability of the second event. This rule is crucial for applications in Bayesian statistics, influencing how probabilities are updated with new information.
Why is the 1/e approximation surprising in the context of the De Montmort problem?
-The 1/e approximation is surprising because it emerges from a purely combinatorial setup involving permutations of cards, which seems discrete and unrelated to the continuous nature implied by the number e. This convergence to approximately 1/e, regardless of the size of n, is counterintuitive but demonstrates the profound connections in probability theory.
What does the speaker suggest about guessing probabilities when uncertain?
-The speaker humorously suggests that if one is completely uncertain in a probabilistic scenario, guessing 1/e might be a surprisingly good strategy. This is because 1/e appears frequently in different probability problems, reflecting its deep underlying significance in the field.
Outlines
๐ Recap and De Montmort Matching Problem
The speaker begins by recapping the De Montmort matching problem from the previous session, emphasizing the use of inclusion-exclusion principle. The problem involves a deck of 'n' cards labeled from 1 to 'n', where the game is won if a named card matches the card drawn. The discussion includes the probability of winning, defining events Aj for the jth card matching, and the use of symmetry and inclusion-exclusion to calculate the probability of the union of these events.
๐ Inclusion-Exclusion and Probability Calculations
The speaker delves into the inclusion-exclusion principle, explaining how it's applied to calculate the probability of the union of events. The formula for the probability of the union is given as an alternating sum involving factorials. The speaker corrects a previous oversight regarding the 'plus 1' in the last term of the inclusion-exclusion formula. A sanity check is performed to validate the formula, and the concept of the complement of the union is introduced to find the probability of no matches, leading to an approximation of 1/e for large 'n'.
๐ค Defining Independence and Its Confusion with Disjointness
The speaker introduces the concept of independence between events, providing a formal definition where the probability of the intersection of two events A and B equals the product of their individual probabilities. The difference between independence and disjointness is clarified, with disjoint events being mutually exclusive. The criteria for three events to be independent are outlined, emphasizing that pairwise independence is not sufficient and the need for a multiplicative rule involving all three events.
๐ฒ Newton-Pepys Problem and Dice Probabilities
The speaker presents the Newton-Pepys problem, a historical gambling query from 1693, where the likelihood of rolling at least one six with six dice, at least two sixes with twelve dice, and at least three sixes with eighteen dice is compared. The speaker engages the audience in a poll to gauge their intuitions before revealing that the event of getting at least one six with six dice is the most likely, contrary to what many might have guessed.
๐งฎ Solving the Newton-Pepys Problem Using Probability
The speaker outlines the process for solving the Newton-Pepys problem using probability theory. The approach involves calculating the probability of the complement of the events (i.e., getting no sixes) and then subtracting these from 1 to find the desired probabilities. The calculations are performed step-by-step for each of the three scenarios (A, B, and C), using independence and multiplication of probabilities for the intersection of independent events.
๐ Binomial Probability and the Newton-Pepys Solution
The calculation for the probability of getting exactly k sixes when rolling 18 dice is explained, introducing the concept of binomial probability. The speaker shows how to compute this using combinations (18 choose k) and probabilities for each die (1/6 for a six and 5/6 for a non-six). The results of the calculations reveal that event A (at least one six with six dice) is the most likely, aligning with Newton's findings.
๐ค Conditional Probability and Its Role in Statistics
The speaker shifts the focus to conditional probability, describing it as the central concept for updating beliefs based on new evidence. The definition of conditional probability P(A|B) is given as the probability of A and B occurring together divided by the probability of B. The importance of this concept in science, philosophy, and everyday reasoning is emphasized, and the speaker outlines the intention to explore the mathematical and statistical ideas behind it.
๐ Intuition Behind Conditional Probability
Two intuitive approaches to understanding conditional probability are presented: the pebble world and the frequentist world. In the pebble world, the sample space is represented by pebbles, and the process involves eliminating pebbles not in event B and renormalizing the remaining 'universe' to find P(A|B). The frequentist world perspective involves repeating the experiment many times and observing the fraction of times A occurs given that B has occurred. The speaker assures that these intuitions will become more clear and helpful with further reflection.
๐ข Theorems of Conditional Probability
Several theorems related to conditional probability are derived. The first theorem shows that the probability of the intersection of A and B can be calculated as P(A|B) * P(B) or P(B|A) * P(A). The second theorem generalizes this to the intersection of multiple events, highlighting the importance of the order in which events are conditioned. The third theorem, known as Bayes' rule, provides a relationship between P(A|B) and P(B|A), emphasizing its simplicity and profound implications. The speaker concludes the session with these theorems, noting their utility and the deep controversies they have sparked in statistical theory.
Mindmap
Keywords
๐กInclusion-Exclusion
๐กIndependence
๐กConditional Probability
๐กSymmetry
๐กComplement
๐กTaylor Series
๐กNewton-Pepys Problem
๐กBinomial Probability
๐กBayes' Rule
๐กDisjoint Events
๐กPebble World
Highlights
Introduction to the De Montmort matching problem, an example of inclusion-exclusion.
Explanation of independence in probability and how events are considered independent.
Discussion on conditional probability and its significance throughout the course.
Detailed analysis of the De Montmort problem using inclusion-exclusion to calculate probabilities.
Illustration of probability calculations through the symmetry of problems and how they simplify complex probability evaluations.
Introduction of the concept that the probability of no match in a matching problem is an application of Taylor series, particularly e to the power of x.
Unexpected result of the no match probability approaching 1/e, demonstrating a surprising application of mathematical constants in discrete problems.
Definition of independence using the probability of intersections and the simple multiplication of probabilities.
Contrasting independence with disjoint events, emphasizing their fundamental differences.
Definition and implications of conditional probability, pivotal in updating beliefs based on new evidence.
Introduction of Bayes' rule and its foundational role in Bayesian statistics, highlighting the simplicity of its algebra but the depth of its implications.
Explanation of pairwise independence and the necessity of considering joint independence in multiple events.
Newton-Pepys problem discussion, illustrating historical context and practical application of probability theory in gambling.
Practical application of independence in solving probability problems involving dice, showcasing problem-solving strategies.
Exploration of how continuous updating of beliefs and probabilities is central to the practice of science and rational decision making.
Transcripts
Browse More Related Video
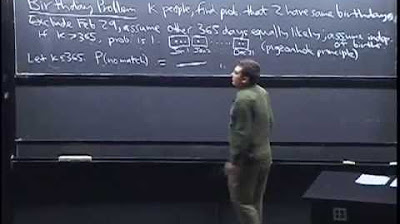
Lecture 3: Birthday Problem, Properties of Probability | Statistics 110
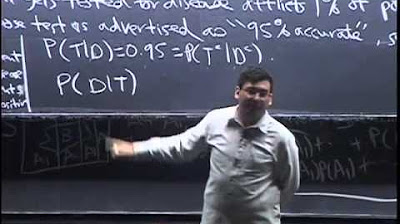
Lecture 5: Conditioning Continued, Law of Total Probability | Statistics 110

8. Alan Hรกjek: The Extremely Improbable
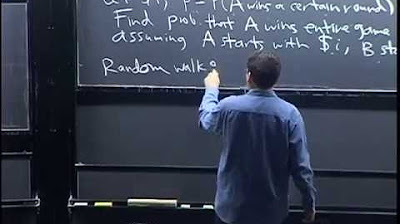
Lecture 7: Gambler's Ruin and Random Variables | Statistics 110
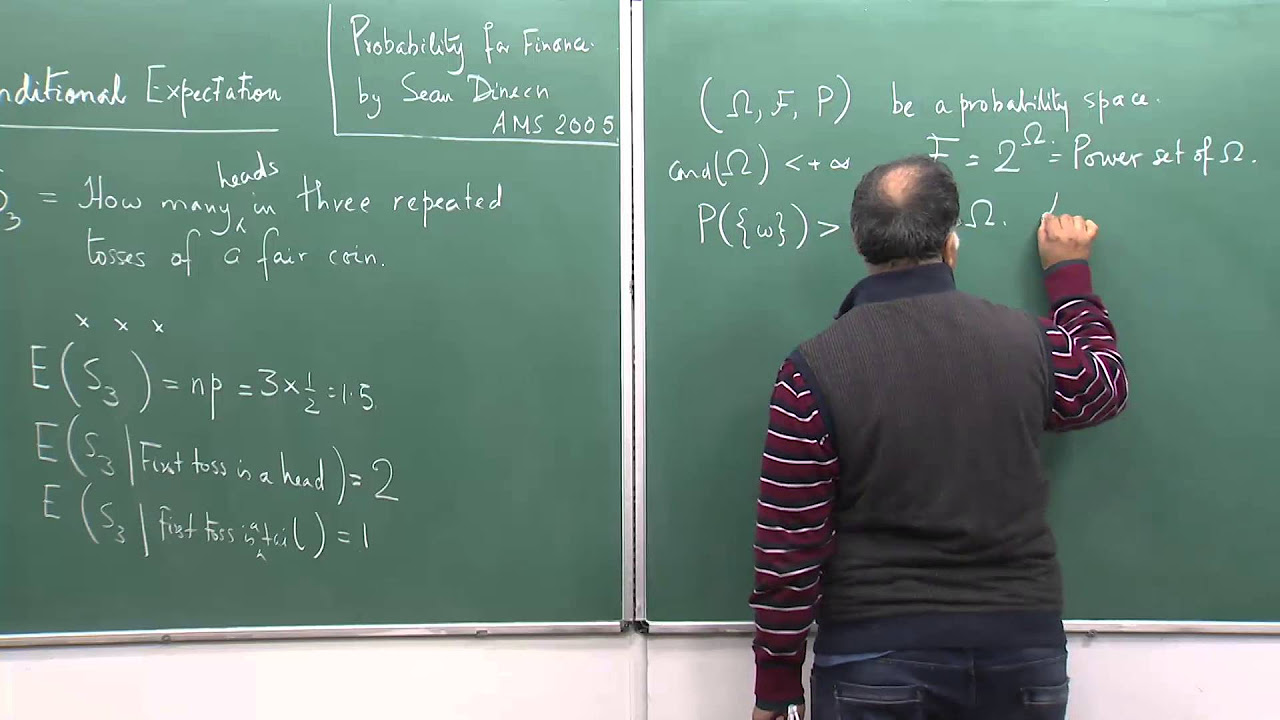
Conditional Expectation-I

Lecture 2: Interesting problems in probablity
5.0 / 5 (0 votes)
Thanks for rating: