How To Catch A Cheater With Math
TLDRThe video script explores the concept of frequentist hypothesis testing through a fictional game in the nation of blobs, where cheating with biased coins is suspected. It introduces the idea of designing a test to minimize false accusations of cheating while maximizing the detection of actual cheaters. The script uses coin flipping as a metaphor for statistical analysis, discussing concepts such as false positives, true positives, and the importance of understanding assumptions in data interpretation. The lesson emphasizes the need for a balance between testing sensitivity and specificity, and the potential impact of incorrect assumptions on the outcomes of tests.
Takeaways
- π² The nation of blobs plays a simple game based on flipping coins, where happiness and sadness are derived from the outcomes.
- π§ There are rumors of players using trick coins that bias towards heads, leading to an unfair advantage and a need for a method to catch these cheaters.
- π― A warmup activity involves having each blob flip their coin five times, resulting in a range of outcomes from zero to five heads.
- π The concept of frequentist hypothesis testing is introduced as a method to make decisions with limited data and to create a test for detecting cheating in the coin flipping game.
- π« It's acknowledged that due to randomness, it's impossible to be completely sure if a blob is cheating, but better approaches can be developed.
- π² The challenge is to design a test that has a low chance of wrongly accusing fair players, high chance of catching cheaters, and uses the fewest number of coin flips possible.
- π The video discusses the importance of understanding the balance between false positives and true positives, and introduces the terms negative and positive test results.
- π The binomial distribution is mentioned as a formula to calculate probabilities in more complex scenarios involving multiple coin flips.
- π The process of designing the test involves setting goals for the false positive rate and the true positive rate, and adjusting the test rules to meet these goals.
- π€ The video emphasizes the importance of being aware of assumptions made in the process and the potential for these assumptions to affect the outcomes and conclusions.
- π The concept of P-value is introduced as a measure for a single test result, helping to determine if a result is likely from a cheater or a fair player.
Q & A
What is the main objective of the blob game based on flipping coins?
-The main objective of the blob game is to flip their own coin and observe the outcomes, where heads bring happiness and tails bring sadness to the participants.
What rumor is circulating in the blob nation regarding the game?
-The rumor is that some players are using trick coins that come up heads more than half the time, which is considered unfair.
What is the purpose of the warm-up exercise where blobs flip their coins five times?
-The purpose of the warm-up exercise is to gather data on the outcomes of the coin flips, which can later be used to identify potential cheaters.
What is the significance of the 88% statistic mentioned in the script?
-The 88% statistic indicates that when a blob gets five heads out of five flips, it is only a cheater about 88% of the time, highlighting the inherent uncertainty in detecting cheating based on randomness.
What are the three key requirements for the test being designed to detect cheaters?
-The test should: 1) have a low chance of wrongly accusing a player using a fair coin, 2) have a high chance of catching a player cheating with an unfair coin, and 3) use the smallest number of coin flips possible.
What is frequentist hypothesis testing?
-Frequentist hypothesis testing is a statistical method for making decisions with limited data, which involves designing a test to differentiate between outcomes based on predefined models and thresholds.
What is the standard choice for the false positive rate in the context of this coin flipping game?
-The standard choice for the false positive rate is 5%, meaning one false accusation out of every 20 fair players.
How is the concept of 'true positive' and 'false positive' applied in the context of the blob game?
-A 'true positive' occurs when the test correctly identifies a cheater, while a 'false positive' happens when the test wrongly accuses a fair player of cheating.
What is the role of the 'P value' in the context of the test results?
-The P value represents the probability of obtaining a result as extreme or more extreme than the observed results, assuming the null hypothesis (that the player is fair) is true. It helps determine if the result is significant enough to accuse a player of cheating.
Why did the test fail to catch 80% of the cheaters in the final group of 1000 blobs?
-The test failed to catch 80% of the cheaters because the underlying assumption about the cheaters' coins coming up heads 75% of the time was incorrect. The real cheaters' coins came up heads only 60% of the time, which required a different test design to achieve the goals.
What is the main lesson learned from the incorrect assumption about the cheaters' coins?
-The main lesson is the importance of recognizing and accounting for assumptions when designing tests and interpreting results. Assumptions can lead to incorrect conclusions if they are not accurately reflected in the test design and analysis.
Outlines
π² Introducing the Blob Coin Flipping Game
The video script begins with a description of a popular game in the nation of blobs where participants flip coins and the outcome of the coin tosses dictates their emotions. It is mentioned that some players are suspected of using trick coins that are biased towards landing on heads. To address this issue, a warmup activity is suggested where blobs flip their coins five times, and the results are analyzed. The script introduces the concept of frequentist hypothesis testing as a method to determine if a player is cheating and outlines the criteria for an effective test, which includes low false accusation rates for fair players, high catch rates for cheaters, and minimal use of resources.
π§ Evaluating the Coin Flipping Test
This paragraph delves into the evaluation of the coin flipping test's performance. A set of 1000 players is simulated, with half being cheaters, to test the effectiveness of the proposed test. The results show that the test falsely accuses a small percentage of fair players, but it does not catch many cheaters. The script introduces statistical terms such as negative and positive results, true negatives, false positives, true positives, and false negatives. It also discusses the concept of the false positive rate and the importance of understanding the implications of these terms when making conclusions.
π Refining the Cheating Detection Test
The paragraph focuses on refining the test to better catch cheaters while maintaining a low false positive rate. It emphasizes the need to balance the test's sensitivity and specificity. The script explains the concept of statistical power and introduces the binomial distribution as a tool for calculating probabilities in more complex scenarios. It also discusses the limitations of the test when the effect size (the probability of a cheater's coin landing heads) is not accurately known.
π Analyzing Test Results with P-Values
This section of the script introduces the concept of P-values in the context of the coin flipping test. It explains how P-values can be used to interpret individual test results and make decisions about whether a player is cheating or not. The script also presents a hypothetical scenario where the test is applied to a new group of blobs and discusses the importance of setting the right thresholds to balance between catching cheaters and minimizing false accusations.
π± The Unexpected Outcome
The final paragraph reveals an unexpected outcome when the test is applied to a group of blobs where the assumption about the cheaters' coin was incorrect. The test fails to catch the expected percentage of cheaters due to an incorrect effect size assumption. The script highlights the importance of being aware of assumptions when designing and interpreting tests. It concludes by emphasizing that the framework used in the video is widely applicable in scientific studies and sets the stage for discussing Bayesian hypothesis testing in future content.
Mindmap
Keywords
π‘coin flipping
π‘cheaters
π‘fair coin
π‘frequentist hypothesis testing
π‘false positive
π‘false negative
π‘true positive
π‘true negative
π‘statistical power
π‘P value
π‘binomial distribution
Highlights
The nation of blobs plays a popular game based on flipping coins, where happiness and sadness are derived from the outcomes.
Rumor has it that some players use trick coins that come up heads more often, leading to an unfair advantage.
An interactive version of the game allows players to judge the blobs themselves and identify potential cheaters.
A blob getting five heads out of five flips is a cheater only about 88% of the time, highlighting the role of randomness.
Frequentist hypothesis testing is introduced as a method for making decisions with limited data.
The test aims to minimize false accusations for fair players, maximize the chance of catching cheaters, and use the fewest number of coin flips.
The probability of getting two heads in a row is 25%, calculated by multiplying the probabilities of independent events.
The standard choice for a false positive rate is 5%, or one false accusation out of every 20 fair players.
A test where a player gets five out of five heads accuses them of cheating, based on the assumption of a fair coin's probability.
The test's performance is evaluated through a large dataset of 1000 players, with predictions made before results.
True negative and false positive terms are introduced, relating to the correctness of test results in relation to reality.
The statistical power of a test, or its ability to detect a cheater, is targeted at 80%.
The effect size, representing the impact of using an unfair coin, is a crucial factor in determining test accuracy.
The binomial distribution formula is mentioned as a tool for calculating probabilities in more complex scenarios.
A test rule is developed where a blob flipping a coin 23 times and getting 16 or more heads is accused of cheating.
The P-value is introduced as a measure of the probability of a test result occurring under the assumption of innocence.
The test's effectiveness is challenged when assumptions about the cheaters' coin are incorrect.
The video concludes by emphasizing the importance of remembering assumptions and the framework's applicability to scientific studies.
Transcripts
Browse More Related Video
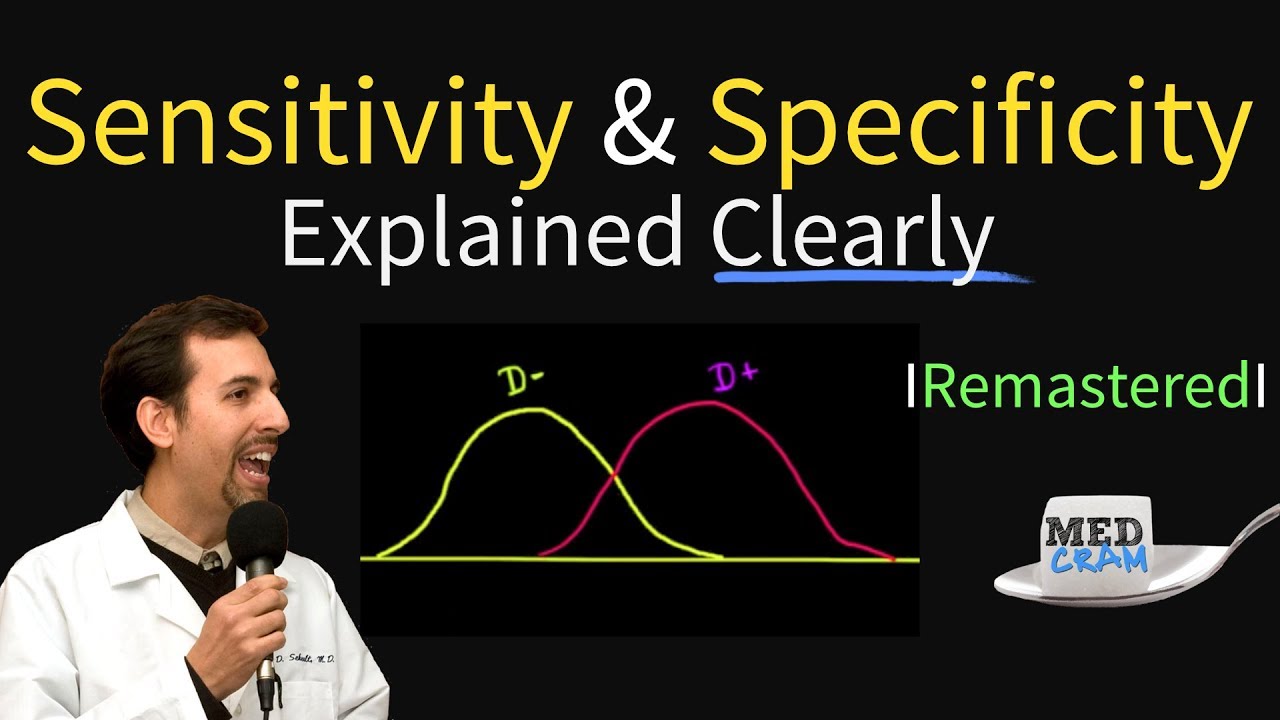
Sensitivity vs Specificity Explained (Medical Biostatistics)
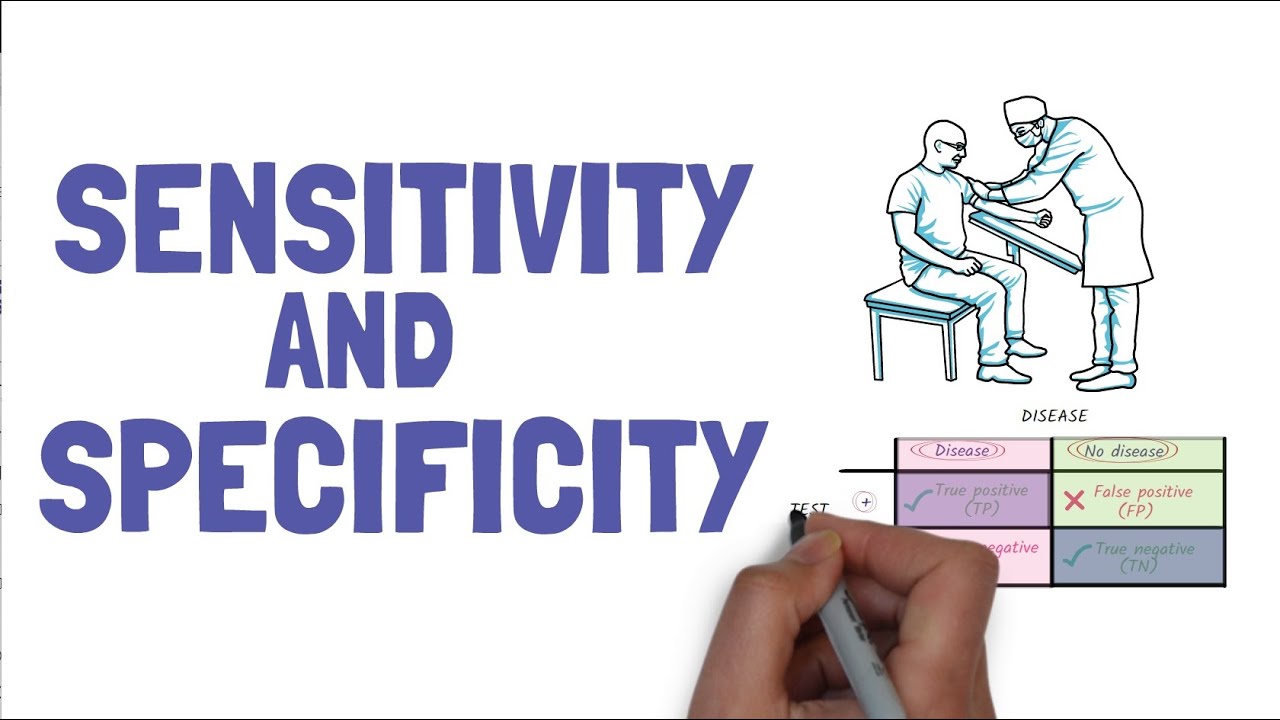
Sensitivity and Specificity simplified
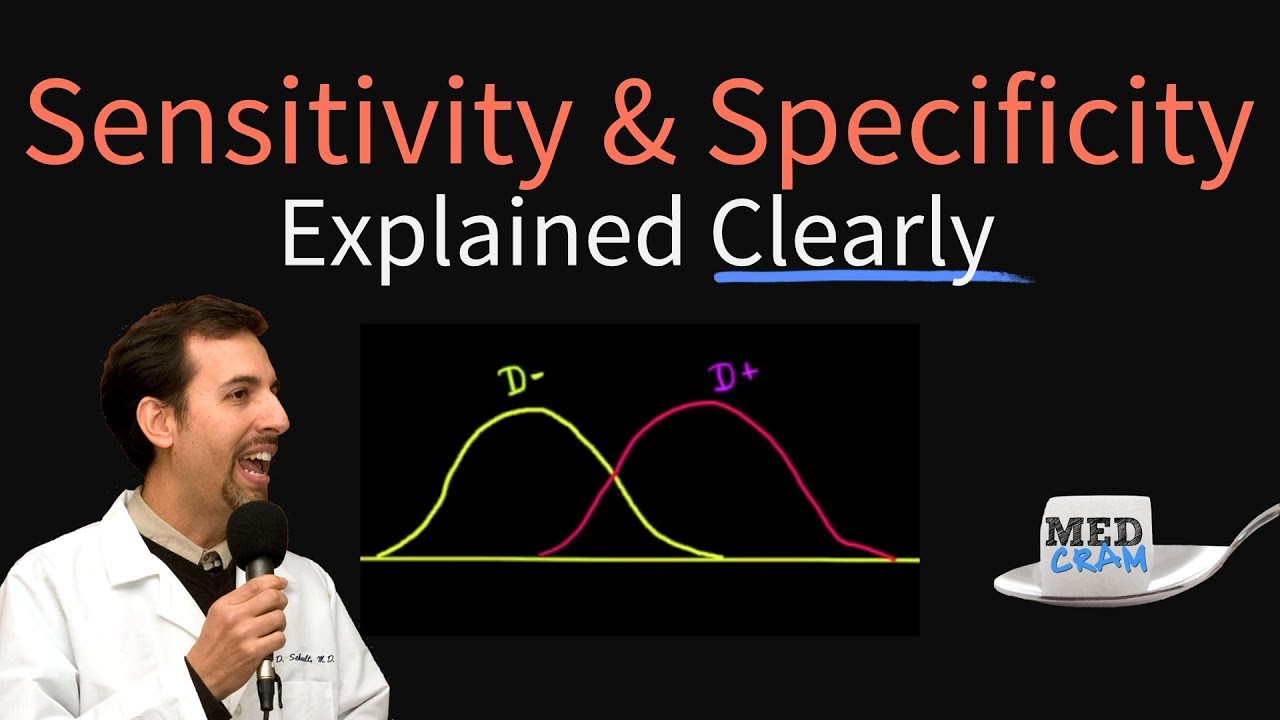
Sensitivity and Specificity Explained Clearly (Biostatistics)
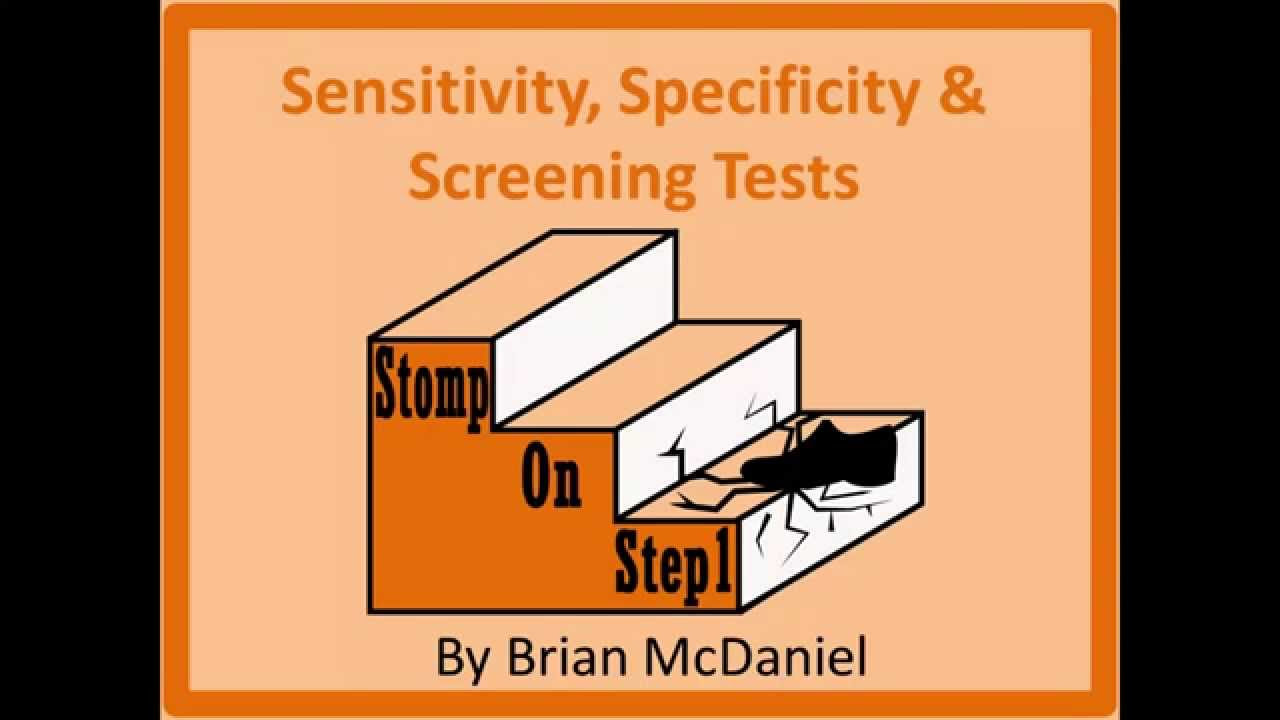
Sensitivity, Specificity, Screening Tests & Confirmatory Tests
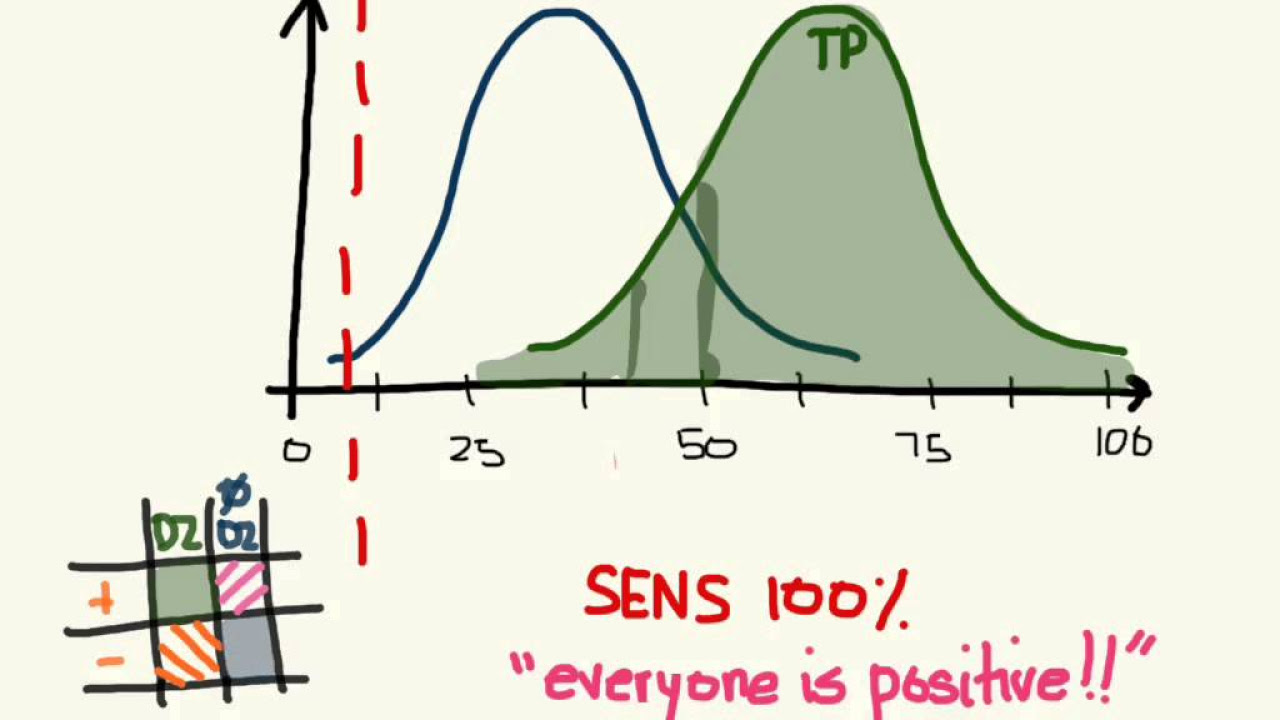
The tradeoff between sensitivity and specificity
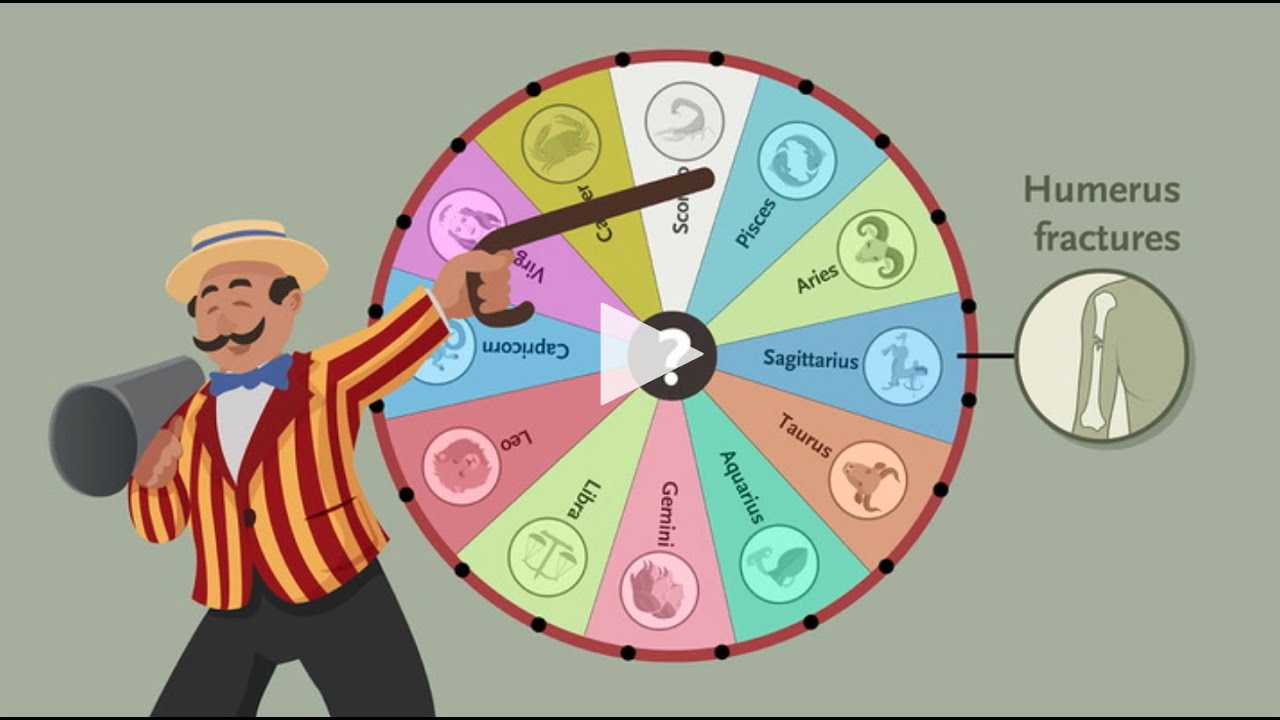
The Problem of Multiple Comparisons | NEJM Evidence
5.0 / 5 (0 votes)
Thanks for rating: