Why AI art struggles with hands
TLDRThe video explores why AI art models struggle to generate realistic hands compared to other body parts. It traces this back to three key factors - the limited hand photo data they train on, the complexity of hand poses and motions, and the low tolerance viewers have for inaccuracies in rendered hands. The narrator contrasts how artists simplify forms to tackle complexity while AIs directly interpolate available data. Though improving, models still fail to capture the precise rules governing hands, unlike faces. More training data and human ranking of results may overcome this, guiding AIs to meet people's higher standards for hands.
Takeaways
- ๐ AI struggles with drawing realistic hands compared to other body parts
- ๐ Humans learn to draw hands through pattern recognition from seeing many examples
- ๐ค AI learns differently - by seeing labeled images without real world interaction
- ๐ There's less quality hand image data for AI art to train on compared to faces
- ๐ Hands can contort in many more positions than predictable parts like faces
- ๐ต The variety of hand poses means the AI has trouble learning clear rules
- ๐จ Artists simplify subjects like hands into basic shapes before adding detail
- ๐ AI focuses on textures and lighting details but misses structural rules
- ๐ง Engineers are working on getting more data and feedback to improve AI hands
- ๐ Despite issues with hands, AI art keeps advancing in quality and capabilities
Q & A
Why does the narrator say that AI art is basically bad at art?
-The narrator says this because AI models struggle to create anatomically accurate depictions of human hands. This shows that while AI can create art, it lacks fundamental artistic skills like accurately representing real world objects.
What are the three main reasons the narrator gives for why AI struggles with drawing hands?
-The three main reasons are: 1) Lack of quality hand image data 2) The complexity and variability of hand positions/gestures 3) The low margin of error - hands need to look right or they look very wrong
How does the way AI learns differ from the way humans learn to draw?
-Humans simplify complex forms like hands into basic shapes while learning. AI just tries to replicate pixel patterns without understanding anatomy. Humans also get real world experience rotating objects in their hands.
What solutions are being worked on to help AI get better at drawing hands?
-Two main solutions - getting more computing power to train models on vastly more images, and incorporating human feedback to fine tune the models towards what people find convincing.
Why does the narrator say AI art models are spending time on things people don't notice?
-Because the models are decent at generating things like natural scenery that people still appreciate, even if they have flaws people don't notice. The models focus less on tricky things like hands.
What is the difference between AI art models and ChatGPT?
-ChatGPT used human feedback to rate which sentences it generated were good or bad during training. This kind of feedback could allow AI art to better learn what people find convincing.
Why can AI generate decent apples but struggle with hands?
-Apples just need to look apple-like, but hands require very specific anatomical accuracy that the models lack exposure to and understanding of.
How are AI art model datasets similar and different to a museum?
-Similar in that the model sees images and descriptions - but very limited. Different in that humans can touch and rotate real objects for a more complex perspective.
Why do hands require a higher standard than things like shirts or apples in AI art?
-Because inaccurate hands look very obviously wrong and break the illusion, while slight errors in patterns like shirts or apple details are less noticeable.
Will AI art models likely improve at depicting hands over time?
-Yes, newer models like Midjourney v5 have already shown some progress. With more data, computing power, and potentially human feedback, hands should steadily improve.
Outlines
๐ฎ Why AI struggles to draw hands
Paragraph 1 explains why AI art struggles to draw realistic hands compared to other body parts. It contrasts how humans learn to draw hands through pattern recognition from real-world exposure versus how AI models learn from limited museum-like datasets. The key reasons identified are insufficient hand image data, lack of detail on hand pose/gestures, and low tolerance for errors in depicting hands.
๐ค Factors behind the AI hand struggle
Paragraph 2 elaborates on 3 main factors making hands difficult for AI art: 1) Smaller datasets of hand images compared to faces, lacking details on hand structure/gestures, 2) Hands are highly complex and versatile unlike predictable faces, and 3) There is little room for error in depicting hands realistically unlike abstract backgrounds.
Mindmap
Keywords
๐กpattern recognition
๐กsimplify
๐กannotation
๐กbias
๐กfeedback
๐กretrain
๐กcompute
๐กquality
๐กengineering
๐กconvincing
Highlights
First significant research finding
Introduction of new theoretical model
Analysis reveals important implications
Proposed methods enable new applications
Results show potential for practical impact
Transcripts
Browse More Related Video
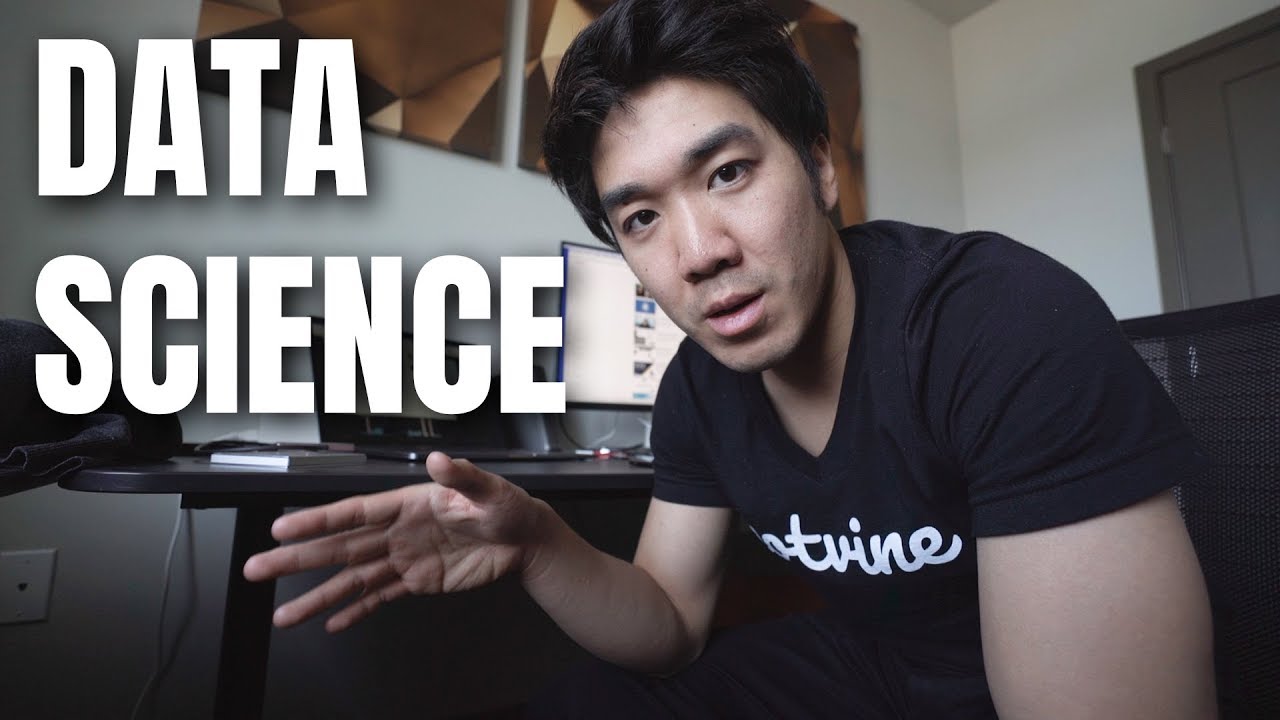
What REALLY is Data Science? Told by a Data Scientist
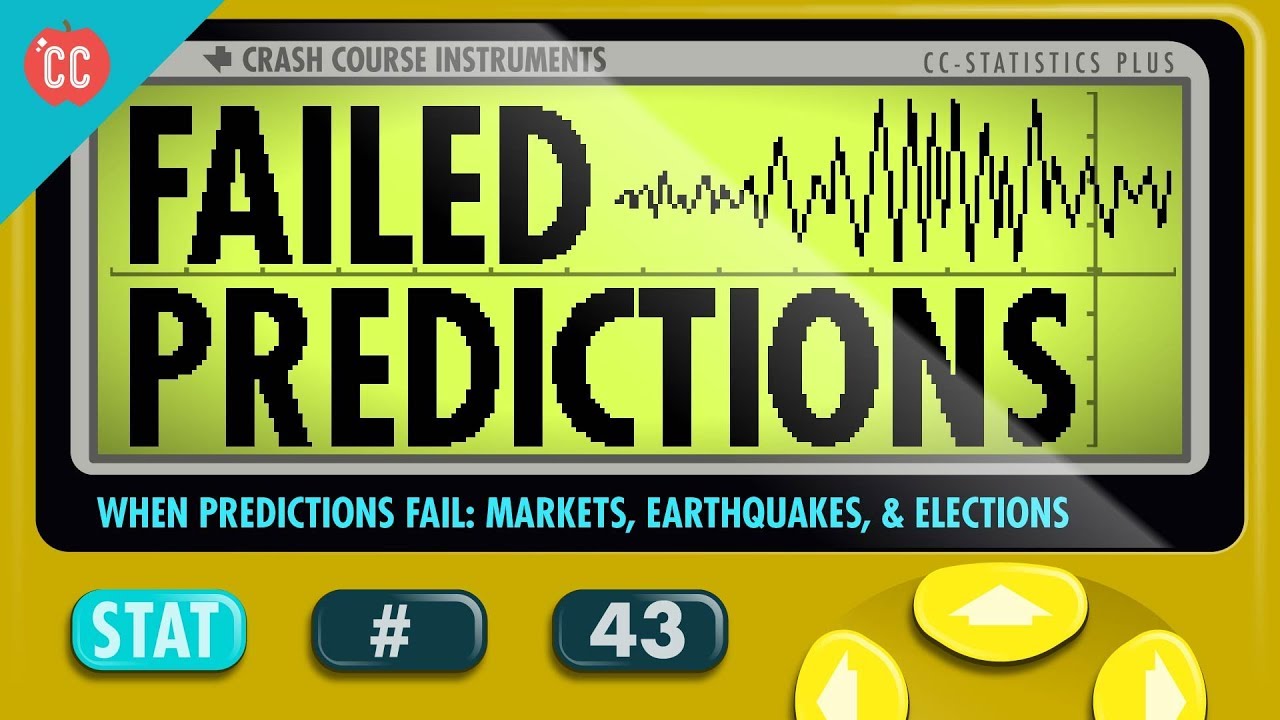
When Predictions Fail: Crash Course Statistics #43
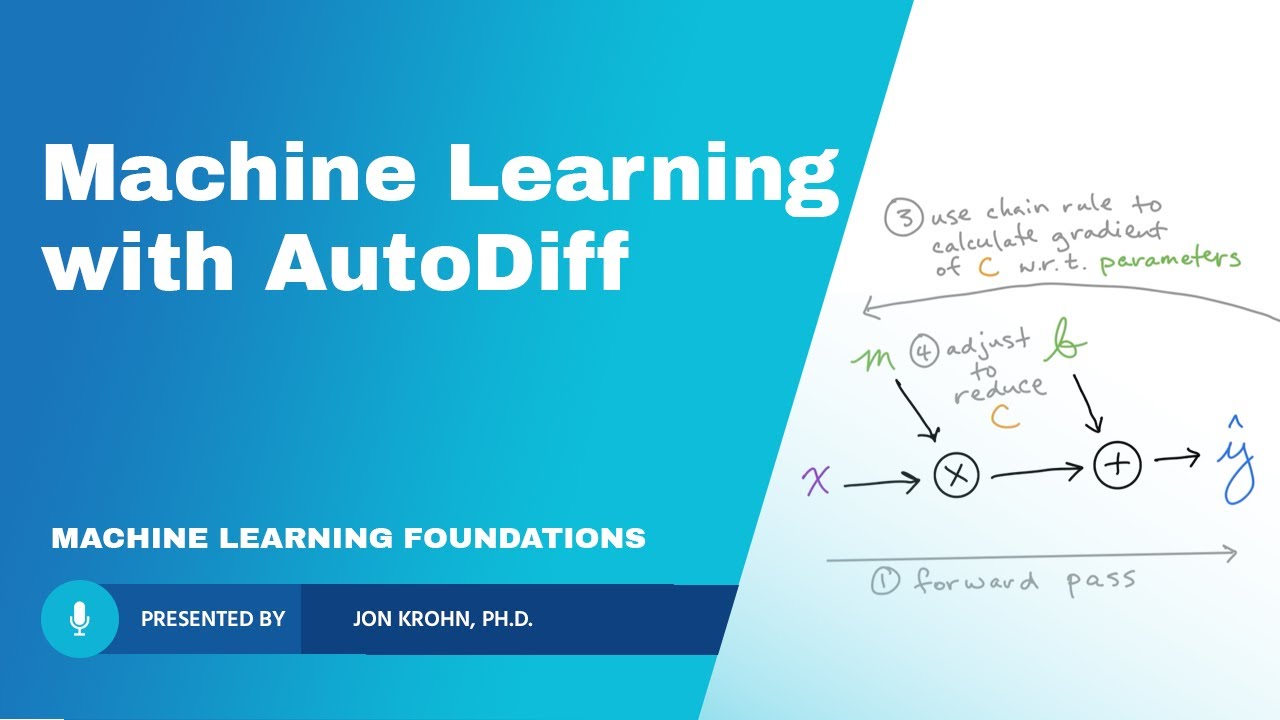
Machine Learning from First Principles, with PyTorch AutoDiff โ Topic 66 of ML Foundations
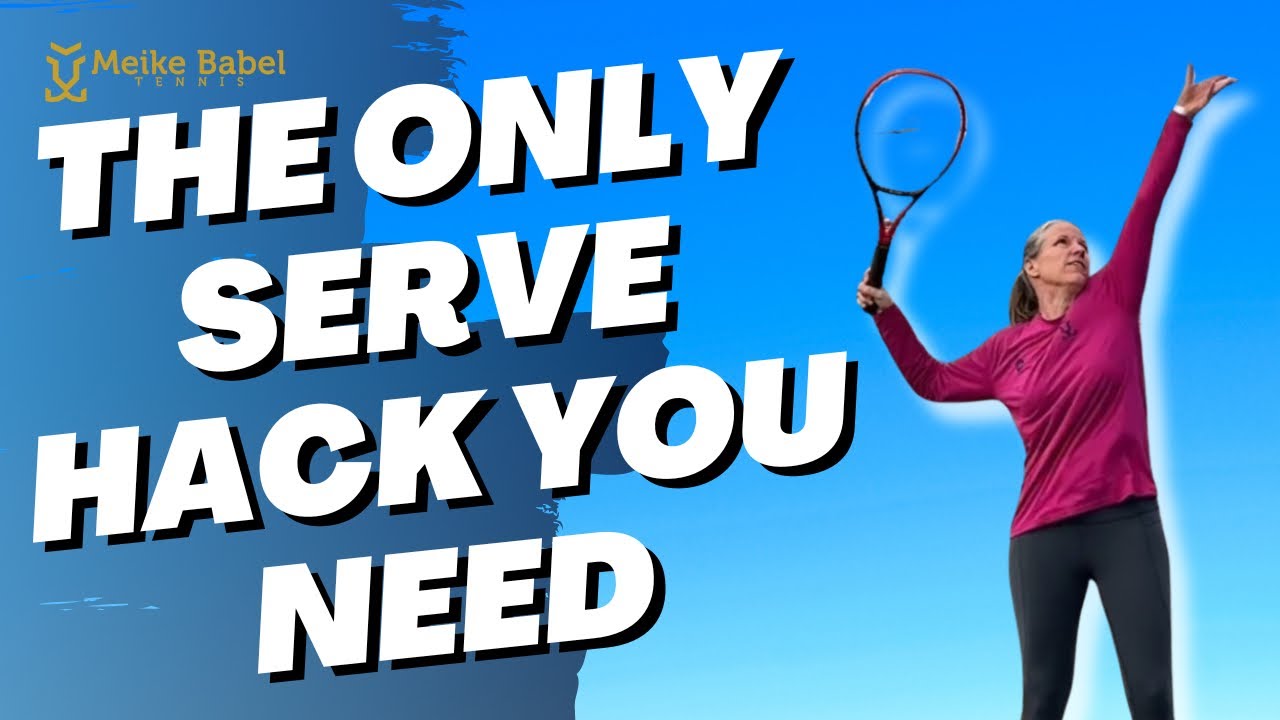
The Best Hack for a Better Tennis Serve for Tennis Players at ANY LEVEL
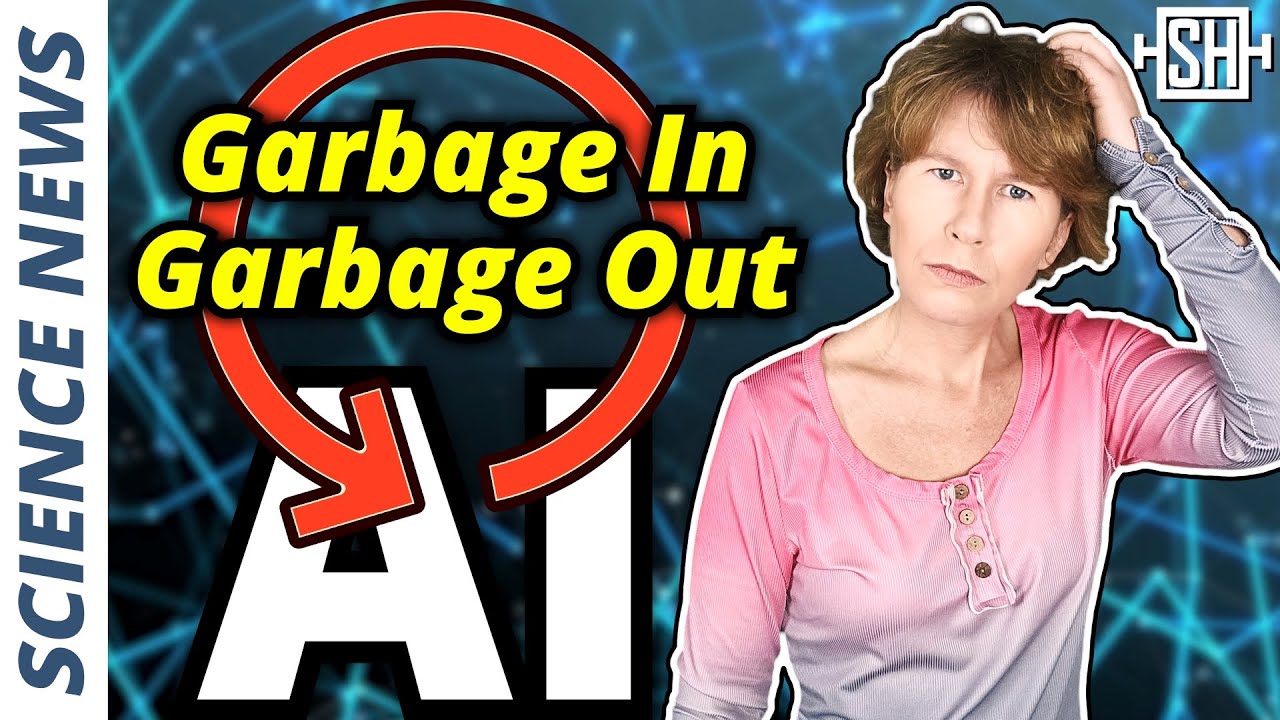
Scientists warn of AI collapse

Recursion x NVIDIA event at JPM2024 โ Fireside Chat with Jensen Huang & Martin Chavez
5.0 / 5 (0 votes)
Thanks for rating: