NLM Science, Technology, and Society Lecture - Confronting Race, Gender, & Ability Bias in Tech
TLDRThe transcript from the Fourth Annual NLM Lecture, hosted by the Office of Strategic Initiatives, delves into the societal and ethical implications of artificial intelligence (A.I.), particularly focusing on bias in A.I. systems. Guest lecturer Meredith Broussard, an associate professor at New York University and author, discusses the misconceptions about A.I., emphasizing that it is not magic and does not operate independently of human biases. She highlights the importance of considering the social context and historical data that train A.I. systems, which often reflect past discrimination and inequality. Broussard shares her personal experience with breast cancer and how it shaped her understanding of A.I. in medicine. She stresses the need for collaboration across disciplines to audit and improve A.I. systems, advocating for a more nuanced and contextual approach to A.I. development and use, especially in high-stakes areas like healthcare and elections. The lecture underscores the critical role of human involvement in creating equitable and trustworthy A.I. technologies.
Takeaways
- π The importance of understanding societal and ethical implications in research, particularly in the development of Artificial Intelligence (AI) technologies.
- π§βπ« Introducing Meredith Broussard, an expert in AI ethics, investigative reporting, and author, who provides insights into bias in AI and its impact on society.
- π« Debunking the myth of AI as magic or Hollywood fantasy, emphasizing that AI is a complex reality based on mathematical patterns and data.
- π Discussing the issue of algorithmic bias, particularly how past societal problems like discrimination and inequality are reflected in the data used to train AI systems.
- π¦ Highlighting real-world examples of AI bias, such as in mortgage-approval algorithms and facial recognition technology, and their societal consequences.
- π€ The need for careful consideration of potential biases when developing AI technologies to avoid perpetuating harmful stereotypes and discrimination.
- π Addressing the challenges of racial disparities in medical diagnosis and AI-read scans, and questioning the use of race as a biological determinant in medical algorithms.
- π The complexity of changing AI systems and pipelines once discriminatory assumptions are embedded, emphasizing the resource-intensive nature of such efforts.
- π€ Encouraging a critical perspective on AI, considering it better suited for low-stakes mundane tasks rather than high-stakes or general-purpose applications.
- π€ The value of interdisciplinary collaboration, including humanists, social scientists, and technologists, to audit algorithms and ensure ethical AI development.
- π The necessity for algorithmic accountability, with recommendations for resources and methodologies to evaluate AI systems in context and their impact on society.
Q & A
What is the main focus of the Fourth Annual NLM Lecture?
-The main focus of the Fourth Annual NLM Lecture is to raise awareness around societal and ethical implications in the conduct of research, particularly concerning the development of artificial intelligence technologies.
Who is the guest lecturer for the event?
-The guest lecturer is Meredith Broussard, an Associate Professor at New York University's Carter Journalism Institute and author of 'More Than a Glitch' and 'Artificial Unintelligence.'
What is the significance of discussing bias in AI?
-Discussing bias in AI is significant because it helps to develop strategies to mitigate bias, which is crucial for creating trustworthy AI systems that do not perpetuate or exacerbate existing social inequalities and discrimination.
What are some of the key documents guiding the development of trustworthy AI?
-Key documents include the Blueprint for an AI Bill of Rights from the White House Office of Science and Technology Policy and the AI Risk Management Framework published by the National Institute of Standards and Technology (NIST).
How does the National Library of Medicine (NLM) contribute to the advancement of equitable health outcomes?
-The NLM contributes by housing and making accessible valuable data resources, leading in health data standards development, and driving data science through its extramural and intramural research programs.
What is the role of the audience in the lecture?
-The audience is expected to engage in important conversations about AI ethics and bias, and to participate in a question-and-answer portion following the lecture.
Why is it important to challenge the idea that AI systems are unbiased?
-It is important to challenge this idea because AI systems can inadvertently perpetuate historical patterns of discrimination, racism, sexism, and structural inequality present in the data they are trained on, leading to biased outcomes.
What is the significance of the term 'Techno Chauvinism' in the context of AI?
-Techno Chauvinism refers to the belief that AI or computational solutions are inherently objective, unbiased, or superior. Challenging this perspective is important to recognize the potential for bias and the need for careful consideration when applying AI.
How can algorithmic bias manifest in real-world systems?
-Algorithmic bias can manifest in systems like mortgage-approval algorithms, which have been found to deny borrowers of color more frequently than white counterparts, or in facial recognition systems that are less accurate for women and people with darker skin tones.
What is the role of the Algorithm Accountability Act?
-The Algorithm Accountability Act, although not explicitly detailed in the transcript, generally aims to ensure that automated decision-making processes within AI systems are fair and transparent, without discrimination or bias.
How does the speaker suggest we approach the development of AI systems to ensure they are used for social good?
-The speaker suggests a multidisciplinary approach involving collaboration between humanists, social scientists, and technologists. This includes algorithmic auditing, benchmarking, and considering the social implications and potential biases before implementing AI systems.
Outlines
π Introduction to the NLM Lecture and Guest Speaker
The Fourth Annual NLM Lecture is organized to raise awareness about societal and ethical implications in research. The guest lecturer, Meredith Broussard, an Associate Professor at NYU and author, is introduced. Her expertise lies in AI ethics, investigative reporting, and using data analysis for social good. The lecture aims to discuss strategies to mitigate bias in AI technologies and is set against the backdrop of increasing discussions around trustworthy AI and recent guiding documents from the U.S. government.
π§ Understanding AI and Algorithmic Bias
Meredith Broussard emphasizes that AI is not magic and dispels Hollywood's portrayal of AI. She discusses the importance of understanding AI's limitations and potential biases. Using the concept of 'Technochauvinism,' she explains that AI systems can default to discrimination because they learn from historical data, which contains patterns of past discrimination. The Markup's investigation into mortgage-approval algorithms is highlighted as an example of this bias.
πΌοΈ Addressing Bias in Automated Systems
The paragraph discusses the need to adjust algorithms to ensure fairness, particularly in lending practices. It also touches on the issues with facial recognition systems, which often exhibit bias based on gender and skin color. The importance of diverse training data is stressed, and the use of AI in high-stakes contexts, such as policing, is questioned. The potential regulation of such technologies is suggested to prevent misuse and discrimination.
π€ Personal Encounters with AI in Medicine
Meredith shares her personal experience with breast cancer during the pandemic and her subsequent exploration of AI's role in medical diagnosis. She recounts her attempt to use an open-source AI to analyze her own medical scans, which led to a series of challenges and misconceptions about AI capabilities in medical imaging and diagnosis.
π Misconceptions and Realities of AI in Cancer Detection
The speaker clarifies misconceptions about AI in cancer detection, explaining that AI typically identifies areas of concern in scans and assigns a score rather than providing a definitive diagnosis. She also discusses the complexities of racial disparities in medical statistics and the limitations of AI in accounting for these factors.
π The Role of Race in Medical and AI Systems
Meredith Broussard addresses the issue of race in medical algorithms and AI systems, noting that race is a social construct rather than a biological reality. She provides examples where race has been incorrectly used in medical calculations, such as kidney disease diagnosis and concussion assessments, and the recent changes made to remove race as a variable in these algorithms.
π« The Problematic Embedding of Race in AI
The paragraph discusses the issues with large language models (LLMs) and their propagation of race-based medicine. It highlights the biases in ML systems and the need for more thoughtful and less discriminatory AI applications in healthcare and other fields.
π€ Reflecting on AI's Role and Development
Broussard expresses skepticism about the timeline for improving AI, suggesting that many easy problems have already been solved by computers and what remains are complex sociotechnical problems. She references the AI Democracy Project by ProPublica, which benchmarked leading AI models for their potential to generate misinformation, especially in the context of U.S. elections.
π€ The Importance of Collaboration in AI
The speaker advocates for a collaborative approach to AI development, involving humanists, social scientists, and biomedical researchers. She emphasizes the need for algorithmic auditing and benchmarking to ensure AI technologies are accessible and unbiased. Broussard suggests that AI is better suited for low-stakes, mundane tasks rather than high-stakes decisions.
π Resources for Further Learning
Meredith provides a list of resources for further learning about racial disparities in medicine and AI, including books, social media accounts, and organizations focused on algorithmic accountability and responsible AI practices.
π€ Audience Q&A and Final Thoughts
The session concludes with a Q&A where the audience asks about the specifics of harm in the context of election information, the role of algorithmic auditing, and the importance of considering social determinants of health when evaluating AI systems. The speaker emphasizes the need for granular, context-specific evaluations of AI and the importance of consulting with affected communities in technology design.
Mindmap
Keywords
π‘Algorithmic Bias
π‘Artificial Intelligence (AI)
π‘Data Analysis
π‘Health Disparities
π‘Machine Learning
π‘Misinformation
π‘Racial Disparities
π‘Social Constructs
π‘Technochauvinism
π‘Transparency
π‘Algorithmic Accountability
Highlights
The lecture emphasizes the societal and ethical implications of research in artificial intelligence (AI), aiming to spark important conversations within the biomedical research community.
Meredith Broussard, the guest lecturer, is an associate professor at New York University and author of 'More Than a Glitch' and 'Artificial Unintelligence', discussing AI ethics and its impact on society.
AI is not magic and does not operate on Hollywood's vision, contrary to public misconceptions; it's a complex and beautiful field of study based on math and data.
AI systems can inadvertently discriminate by default due to historical patterns of discrimination reflected in the training data.
The Markup's investigation revealed mortgage-approval algorithms were biased, denying borrowers of color at a higher rate than white counterparts.
Facial recognition systems were found to have accuracy disparities based on gender and skin tone, with the worst performance on women with dark skin.
The use of facial recognition in high-risk contexts, such as policing, needs careful consideration due to its potential for misidentification and discrimination.
Broussard shares her personal experience with breast cancer and her investigation into AI's role in medical diagnosis, uncovering her own misconceptions about AI in medicine.
AI in cancer detection identifies areas of concern in scans and assigns scores, but these scores are not definitive predictions of cancer.
There are racial disparities in medical diagnosis and AI-read scans, with some models showing different accuracies based on race.
Race is a social construct, not a biological reality, yet it is sometimes erroneously embedded in medical algorithms and AI systems.
The kidney disease diagnosis algorithm previously included a race correction, which has now been removed due to its discriminatory nature.
Large language models (LLMs) can propagate harmful, race-based medicine due to their inability to parse for content, treating popularity as a proxy for quality.
Broussard questions the expectation that AI will eventually overcome its biases, given the persistence of social problems throughout history.
The AI Democracy Project by Proof Labs assessed leading AI models for their potential to generate misinformation in the context of U.S. elections.
A four-dimensional approach to evaluating AI performance includes assessing for inaccuracy, harm, incompleteness, and bias.
Broussard emphasizes the importance of looking for human problems within AI systems and the need for collaboration between technologists, humanists, and social scientists.
AI is better suited for low-stakes, mundane tasks rather than high-stakes or general-purpose use, where the risks of bias and inaccuracy are higher.
Transcripts
Browse More Related Video
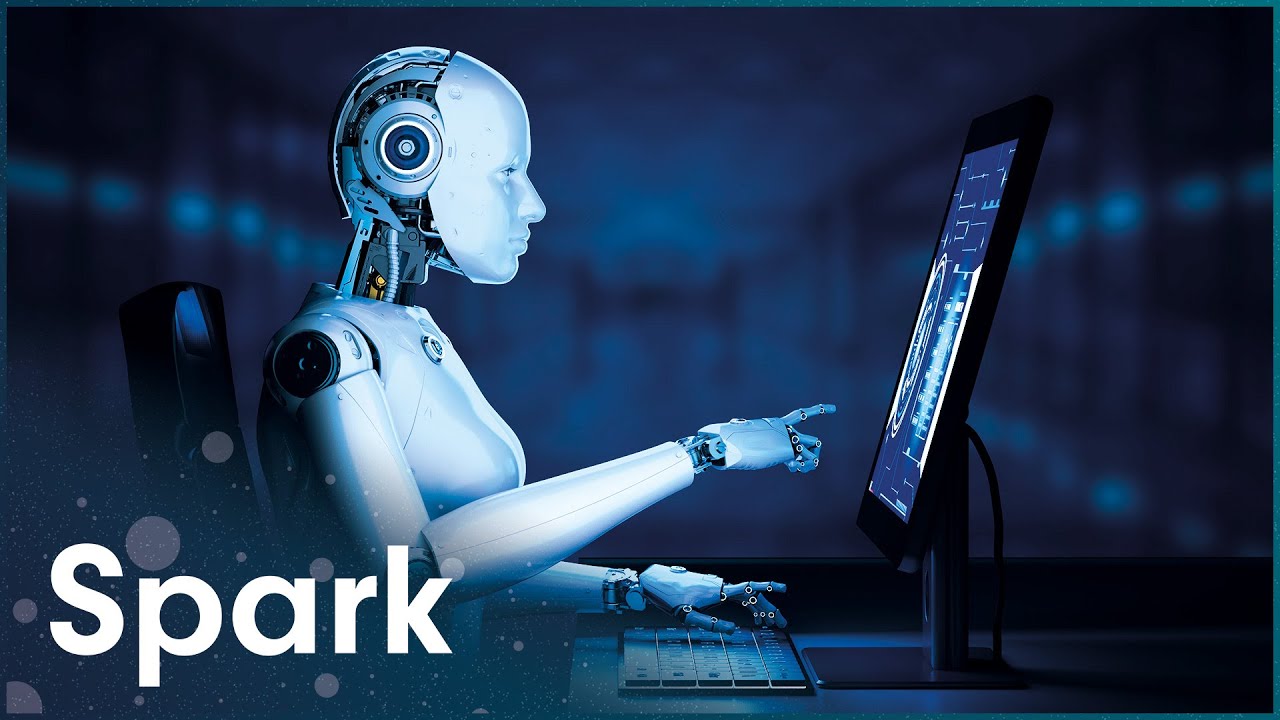
How AI Is Changing The Future Of The Human Race | Spark
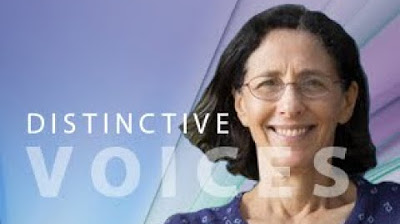
The Future of Artificial Intelligence
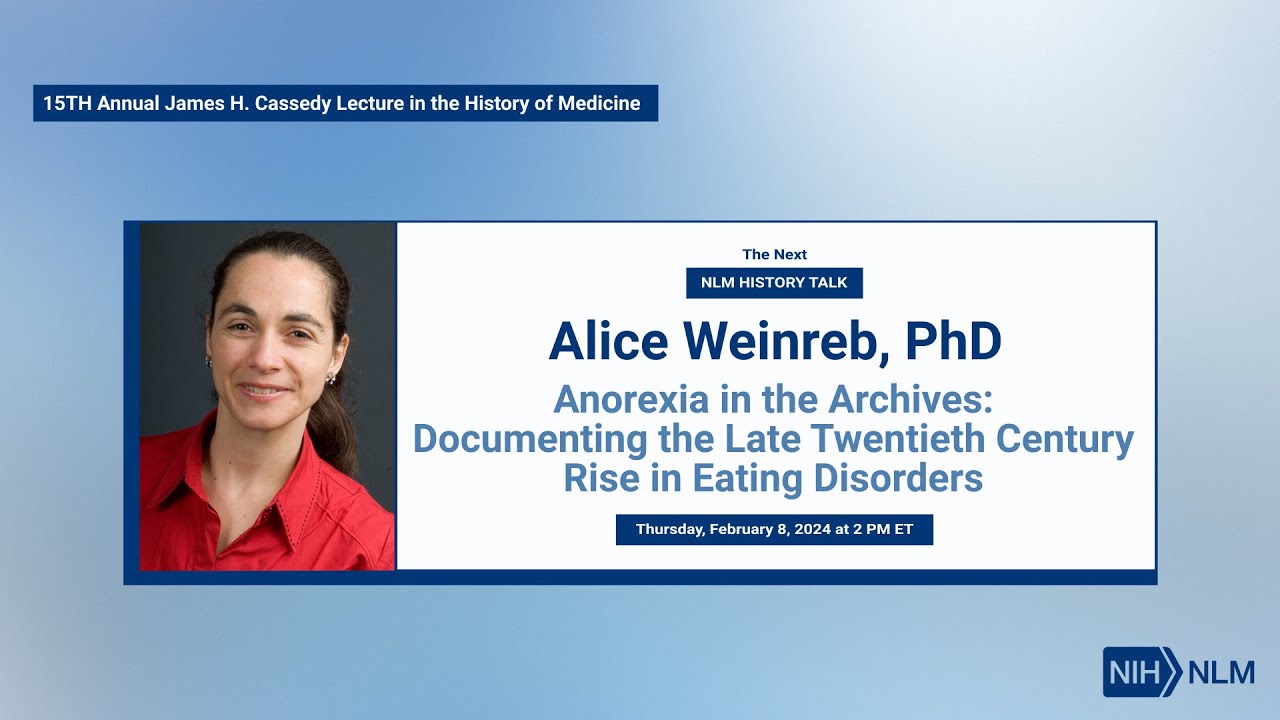
Anorexia in the Archives: Documenting the Late Twentieth Century Rise in Eating Disorders
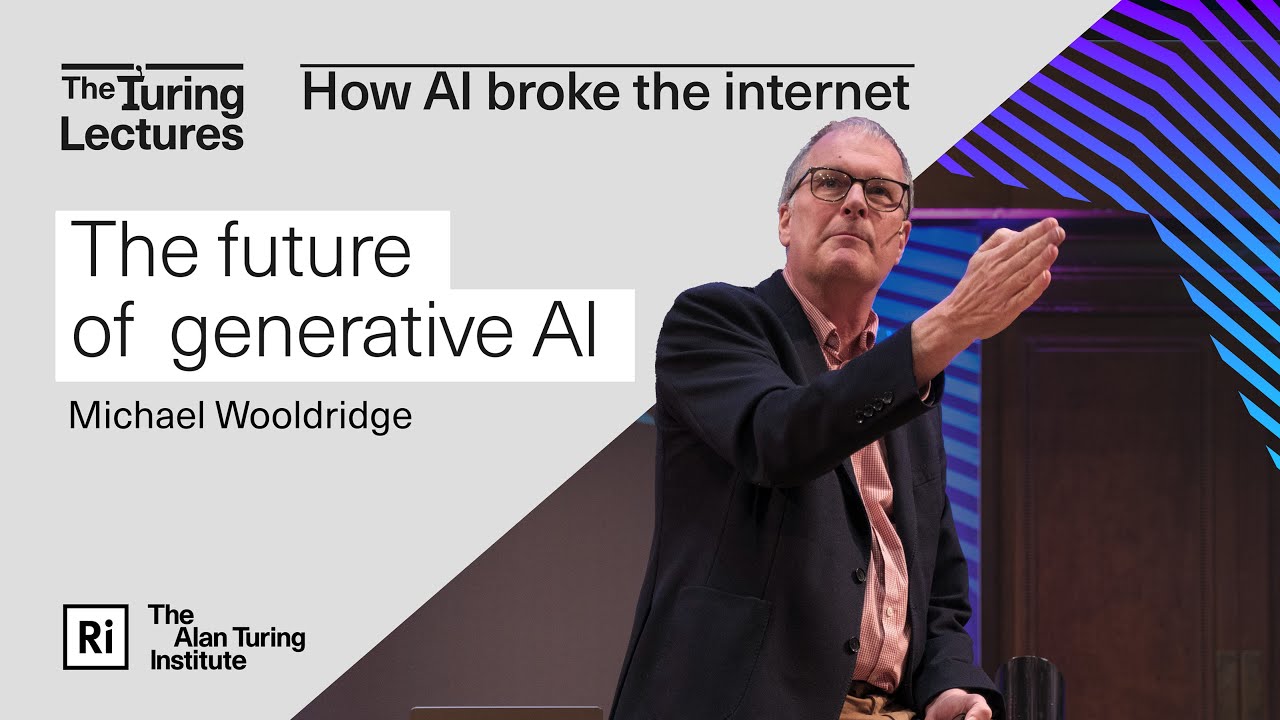
The Turing Lectures: The future of generative AI

Mel Robbins: This One Hack Will Unlock Your Happier Life | E108
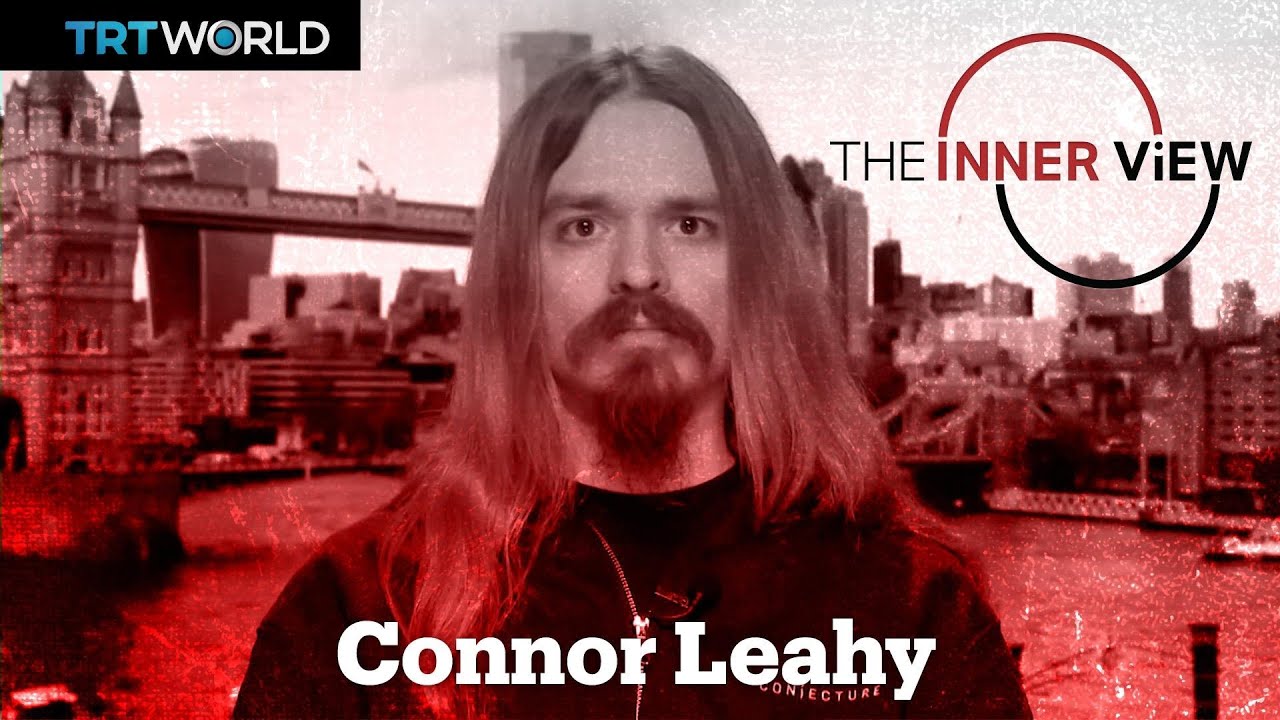
Why this top AI guru thinks we might be in extinction level trouble | The InnerView
5.0 / 5 (0 votes)
Thanks for rating: