A3D3 seminar: Is machine learning good or bad for astrophysics?
TLDRIn the March 2024 A3 D3 seminar, David Hogg, a professor of physics and data science, discusses the role of machine learning in astrophysics. He highlights the potential biases and limitations of machine learning models, emphasizing the need for trust and interpretability in their application. Hogg explores the use of machine learning for emulators, label transfer, and causal inference, while cautioning against over-reliance on these tools without proper validation and understanding of their underlying mechanisms.
Takeaways
- π David Hogg, a professor of physics and data science, discusses the application of machine learning in astrophysics and its potential trustworthiness.
- π€ Machine learning methods are seen as untrustworthy partners due to their potential to introduce biases or systematic errors in scientific research.
- π Despite their limitations, untrusted systems like machine learning can be used effectively if structured correctly, allowing for trustworthy scientific outcomes.
- π Hogg highlights that machine learning isn't new to astronomy and has been used in remote sensing with tools like CCDs and infrared detectors.
- π§ The talk emphasizes that while machine learning has not yet led to significant astrophysics discoveries, it has the potential to do so in the future.
- π A key issue in machine learning for science is the assumption that new data will be similar to training data, which is often violated in scientific contexts.
- π Outlier detection is an area where machine learning excels and has historically led to important astronomical discoveries, such as quasars.
- π οΈ Machine learning is valuable in the engineering aspects of scientific projects, including software improvement and data analysis pipeline management.
- π‘ The philosophy of machine learning differs from traditional scientific inquiry as it focuses on data-to-data transformation without needing to understand the underlying model's workings.
- π Interpretability in machine learning has been a goal, but it has not been fully achieved, leaving a gap in understanding what complex models are actually doing.
- π₯ Adversarial attacks on machine learning models reveal their internal workings and the potential for these models to produce incorrect outputs with minor perturbations.
Q & A
What is David's main research interest in the field of observational cosmology?
-David's main research interest in observational cosmology is using galaxies to infer the physical properties of the Universe.
What are the implications of machine learning being untrustworthy partners in scientific research?
-The implications of machine learning being untrustworthy partners include the potential for bad biases or systematic errors when used in certain areas of research.
How does David feel about the application of machine learning in astronomy projects?
-David acknowledges that while machine learning has been successfully applied in astronomy projects, there is no major result in astrophysics that can be solely attributed to machine learning.
What is an example of a machine learning success in another scientific field that David mentions?
-David mentions the success of AlphaFold in predicting protein structures from their sequences as an example of a machine learning achievement in bioinformatics.
What is David's definition of machine learning?
-David defines machine learning as a method whose capability improves substantially with more data, meaning faster than the square root of n, and includes learning the model structure or representation of data.
What are the two fundamental tenets of machine learning according to David?
-The two fundamental tenets of machine learning, according to David, are that the model's only goal is to connect data to data, and the model is good if it does a good job of relating data to data, usually in held-out training data.
What is the main concern David raises about using machine learning for emulators in scientific research?
-David's main concern about using machine learning for emulators is that if the emulator produces surprising results, it may be difficult to verify these results due to the computational expense of simulations, potentially leading to confirmation bias.
What is the issue with using biased estimators in scientific research?
-Using biased estimators in scientific research can lead to incorrect conclusions about the relationships between variables, as they may not accurately represent the true underlying patterns in the data.
How does David suggest improving the trustworthiness of machine learning in scientific applications?
-David suggests that improving communication about the reliability of machine learning outputs, understanding the subjective needs of users, and potentially structuring machine learning methods to resemble physical laws could enhance their trustworthiness in scientific applications.
What is David's stance on the relationship between prediction and understanding in physics?
-David believes that while predictions are important in physics, the biggest breakthroughs often involve changes in the latent structure of models and a deeper understanding of the underlying principles, rather than just improving predictions.
Outlines
π€ Introduction and Speaker Credentials
The seminar begins with an introduction to the speaker, David, a professor of physics and data science at New York University. He is a leading figure in applying machine learning techniques to astronomy and has made significant contributions to observational cosmology, including research on galaxies, stars, and exoplanets. The speaker discusses the potential and pitfalls of machine learning in astrophysics, emphasizing the need for caution due to the lack of interpretability and trustworthiness in machine learning models.
π§ Machine Learning's Role in Science
David discusses the impact of machine learning on various scientific fields, including astronomy. He highlights the limitations of machine learning, such as its inability to provide a true understanding of phenomena like protein folding. He also touches on the importance of engineering in scientific endeavors, including the development of software and data analysis pipelines. David emphasizes that while machine learning is powerful, it should be used with an understanding of its limitations and potential biases.
π Astrophysics and Machine Learning
The speaker delves into the application of machine learning in astrophysics, particularly in outlier detection and dimensionality reduction. He expresses optimism about the potential of machine learning to identify unusual astronomical phenomena. However, he also raises concerns about the reliability of machine learning models for emulating complex simulations, given their potential to produce biased results that may not accurately represent the physical universe.
π€ Trust and Interpretability in Machine Learning
David explores the philosophical differences between machine learning and traditional scientific methods. He argues that machine learning's focus on data-to-data transformation, rather than understanding underlying phenomena, is a departure from the scientific norm. The speaker also addresses the challenge of interpretability in machine learning, noting that despite efforts to understand complex models, there is still a lack of clarity about how they make predictions.
π Case Study: Stellar Spectral Analysis
The speaker presents a case study of using machine learning to analyze stellar spectra and infer properties such as mass, age, and composition. He explains how machine learning can accurately model and predict these properties, but also warns of the potential biases in the estimates. David emphasizes the importance of understanding the limitations of machine learning regressions in scientific contexts, where unbiased estimates are crucial for accurate analysis.
π‘ Positive Outlook on Machine Learning in Astrophysics
Despite the concerns raised, David maintains a positive outlook on the use of machine learning in astrophysics. He highlights the value of machine learning in instrument calibration and causal inference, where its flexibility can help make more conservative and reliable scientific claims. The speaker concludes by emphasizing the need for clear communication about the capabilities and limitations of machine learning in scientific applications.
π Final Thoughts and Open Questions
In the concluding part of the talk, David reiterates the importance of trust in machine learning emulators and the need for the scientific community to address this issue. He discusses ongoing work aimed at improving the trustworthiness of machine learning models in astrophysics, including adversarial training and sanity checks. The speaker leaves the audience with open questions about the future of machine learning in the natural sciences and its potential as a sandbox for broader discussions on trust in AI.
π’ Q&A Session
The seminar concludes with a Q&A session where the audience engages with the speaker on various topics. Questions range from the specifics of machine learning biases in age estimation to the broader implications of machine learning's role in scientific understanding. The speaker emphasizes the importance of understanding the subjective nature of data analysis and the need for clear communication with end-users of machine learning outputs.
Mindmap
Keywords
π‘Astrophysics
π‘Machine Learning
π‘Big Data
π‘Interpretability
π‘Adversarial Attacks
π‘Emulation
π‘Causal Inference
π‘Outlier Detection
π‘Label Transfer
π‘Data-to-Data Transformation
Highlights
David Hogg, a professor of physics and data science, discusses the application of machine learning in astrophysics and its potential biases and errors.
Machine learning methods in astrophysics can't be trusted as they may produce bad biases or systematic errors.
Despite their untrustworthiness, machine learning systems can be used effectively in scientific ways when structured correctly.
Astronomy is built on a history of remote sensing with technologies like CCDs and infrared detectors, which are used without fully understanding their properties.
Machine learning isn't new to astronomy and has been used for precise measurements, similar to how infrared detectors are utilized.
The talk focuses on whether machine learning is beneficial or detrimental to astrophysics, highlighting both positive and negative aspects.
Machine learning has not led to significant results in astrophysics that could not have been achieved without it, but it has components in astrophysics projects.
An example of machine learning's success is AlphaFold, which predicts protein structures but does not explain how proteins fold, showing the limitations of machine learning in scientific discovery.
Machine learning is used in astrophysics for outlier detection, which has historically led to important discoveries like quasars.
The philosophy of machine learning differs from traditional scientific methods, focusing on data-to-data transformation without needing to understand the internal workings.
Machine learning methods are optimized for commercial applications, and their usefulness in scientific contexts is not always guaranteed.
Adversarial attacks on machine learning models reveal that they are not doing what humans think they are doing, which is concerning for scientific applications.
The use of machine learning emulators in place of expensive simulations can lead to confirmation bias and a higher bar for verifying surprising results.
Machine learning regressions generally return posterior estimates, which are biased, rather than likelihood estimates, leading to potential issues in scientific analysis.
Causal inference in astrophysics, such as determining whether observed variations are due to the star or the instrument, benefits from flexible machine learning models.
The key challenge in using machine learning for science is establishing trust in emulators and understanding their limitations and potential biases.
Effective communication about the capabilities and limitations of machine learning outputs is crucial for their appropriate use in scientific research.
The discussion emphasizes the need for a better language to communicate about machine learning in science and the importance of understanding the subjective needs of users.
Transcripts
Browse More Related Video

Computer Scientist Explains Machine Learning in 5 Levels of Difficulty | WIRED

What is logarithm? | Math, Statistics for data science, machine learning

Calculus II: Partial Derivatives & Integrals β Subject 4 of Machine Learning Foundations

what is computational chemistry?!
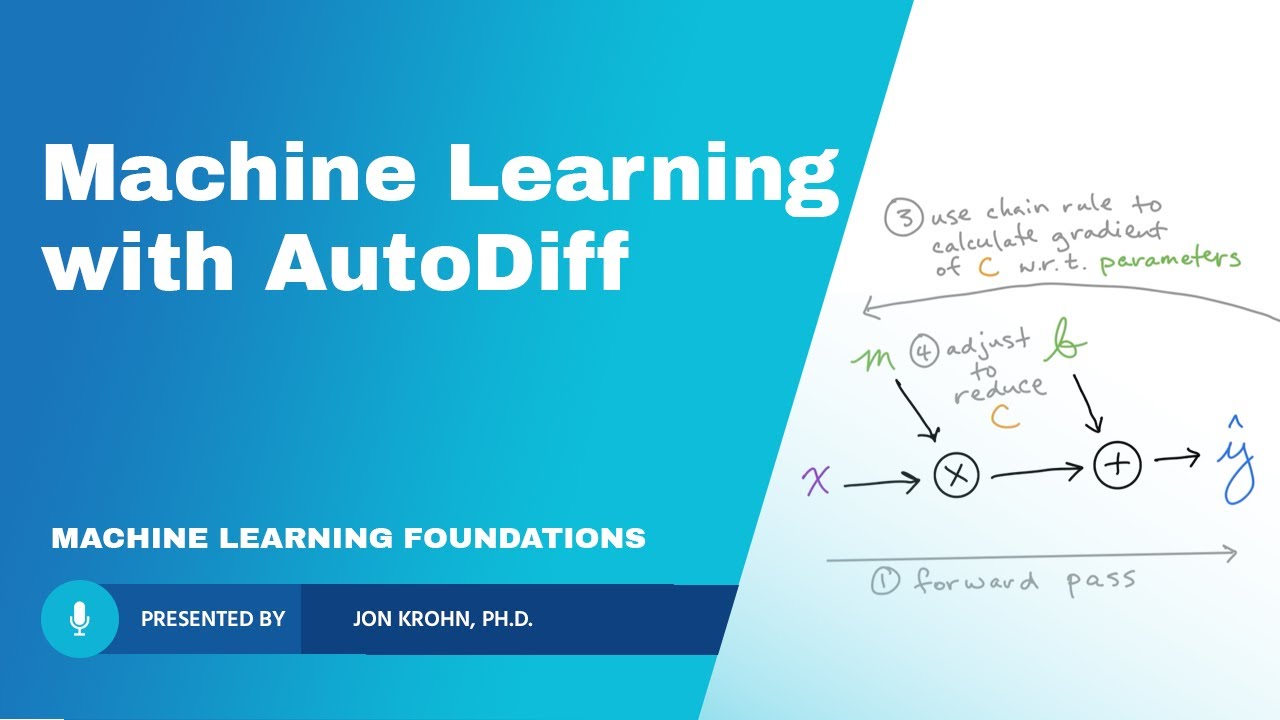
Machine Learning from First Principles, with PyTorch AutoDiff β Topic 66 of ML Foundations
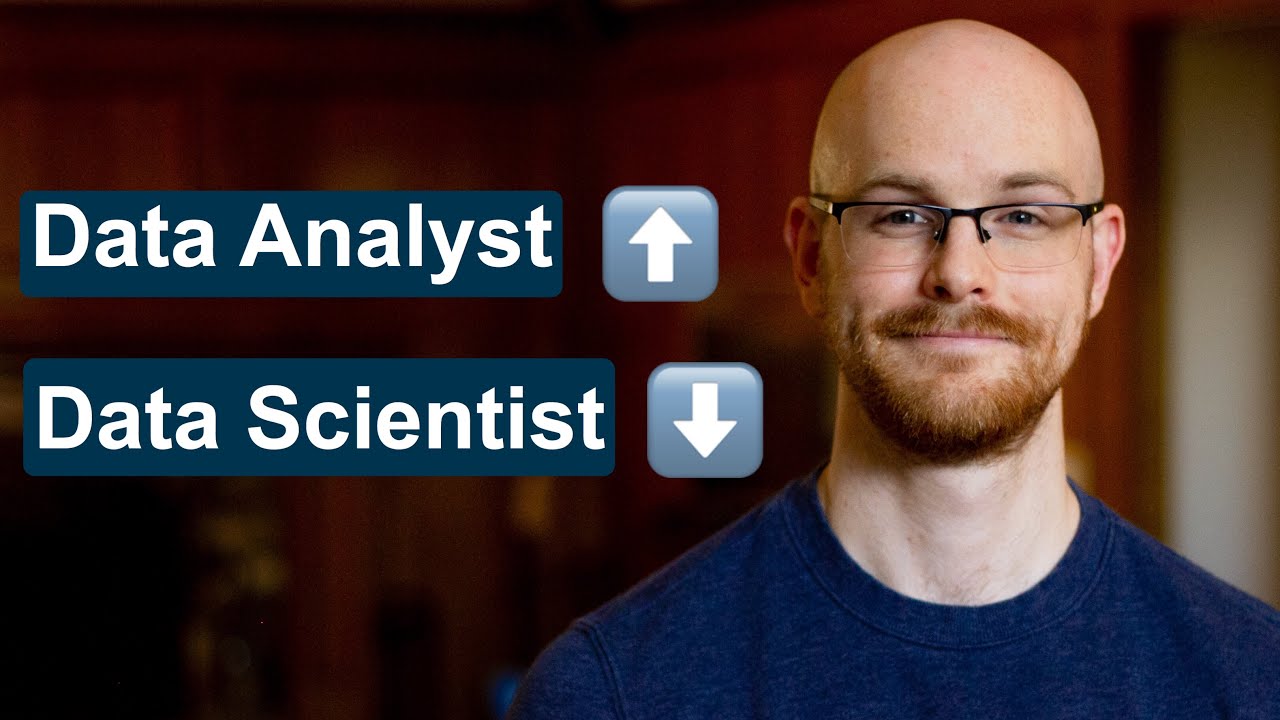
Data Scientist vs Data Analyst | Which Is Right For You?
5.0 / 5 (0 votes)
Thanks for rating: