The Math Behind Basketball's Wildest Moves | Rajiv Maheswaran | TED Talks
TLDRThis talk explores the science of tracking human movement, highlighting its applications in sports. By capturing and analyzing data, machines can now understand complex movements like basketball's pick-and-roll, providing insights coaches and players can use to improve performance. The speaker illustrates how this technology can predict shot probabilities and rebound chances, potentially transforming strategies and outcomes in sports. The discussion extends to envisioning future applications, such as enhancing urban planning and personal milestones, emphasizing the broader impact of spatiotemporal pattern recognition.
Takeaways
- π The science of moving dots refers to tracking and understanding human movement.
- π We can capture detailed movement data through sensors, videos, and apps.
- π Sports, especially basketball, provide a rich source of movement data.
- π€ Machine learning helps analyze complex movements that humans can't process easily.
- π The machine can now recognize advanced basketball plays like pick-and-rolls.
- ποΈ Machines can see the game from a coach's perspective, identifying important patterns.
- π― The key to success in modern basketball is mastering the pick-and-roll play.
- π Machine learning can quantify shot quality and player performance in basketball.
- π Almost all NBA championship contenders use this technology for strategic insights.
- π The principles of tracking movement in sports can apply to everyday life and urban planning.
Q & A
What is the main subject of fascination for the speaker and their colleagues?
-The main subject of fascination for the speaker and their colleagues is the science of moving dots, which represents the movement of people in various environments like homes, offices, and cities.
How is the movement of people being captured and tracked?
-The movement of people is being captured and tracked through various means such as sensors, videos, and apps, which allow for detailed monitoring of movements.
Why is sports one of the best sources of data for studying movement?
-Sports is one of the best sources of data for studying movement because stadiums and players are instrumented to track movements every fraction of a second, providing a wealth of detailed data.
What does the speaker refer to as 'moving dots' in the context of sports?
-In the context of sports, 'moving dots' refers to athletes whose movements are tracked and turned into data points for analysis.
What is the challenge for basketball coaches in analyzing game footage?
-The challenge for basketball coaches is that they would have to watch every second of every game, remember it, and process it, which is not feasible for a human but can be done by a machine.
How has machine learning been applied to help understand complex basketball events?
-Machine learning has been applied by teaching the machine to recognize and differentiate between various basketball events, such as passes, shots, rebounds, and complex plays like pick-and-rolls and down screens, through examples and feature identification.
What is the significance of the pick-and-roll in modern basketball?
-The pick-and-roll is significant in modern basketball as it is perhaps the most important play, with knowing how to run it and defend it being a key to winning and losing most games.
How does the machine identify the variations of a pick-and-roll with high accuracy?
-The machine identifies the variations of a pick-and-roll with high accuracy by using spatiotemporal features such as relative and absolute location, distance, timing, and velocities.
What is the advantage of using machine analysis in basketball over traditional coaching methods?
-The advantage of using machine analysis is that it can process and analyze vast amounts of data with high precision and recall, providing insights that can change strategies and help teams win important games.
How does the machine's understanding of basketball shots contribute to evaluating players?
-The machine's understanding of basketball shots allows for the evaluation of both the quality of the shot and the quality of the shooter, providing a more nuanced assessment of a player's abilities.
What broader implications does the speaker suggest for the science of moving dots?
-The speaker suggests that the science of moving dots could have broader implications beyond sports, such as improving the way we use buildings, plan cities, and potentially even identifying significant moments in everyday life.
Outlines
π Exploring Movement Patterns
The speaker and their colleagues are intrigued by the science of tracking human movement through various environments. By utilizing advanced data collection methods such as sensors, videos, and apps, they capture detailed movement data. This data is particularly rich in sports, where tracking athletes' movements can provide insights that were previously unattainable. However, processing and understanding this data requires sophisticated machine learning techniques to recognize patterns and events in sports like basketball, which coaches can then use to enhance their strategies.
π Teaching Machines to Understand Sports
The process of teaching a machine to recognize complex basketball events involves breaking down movements into simpler components. Starting with basic actions like passes and shots, the machine learns more complex events like pick-and-rolls. This involves understanding intricate details such as timing, distances, and player positions. By using machine learning, the system can identify key features that differentiate various plays, allowing it to see the game with the analytical eye of a coach and provide valuable insights that human observers might miss.
π€ Machine Learning and Pick-and-Rolls
Describing a pick-and-roll to a machine is challenging due to its nuanced nature, involving four players and various possible outcomes. Traditional algorithmic descriptions fall short, but machine learning can surpass human explanations by learning from examples. By feeding the machine numerous instances of pick-and-rolls and non-pick-and-rolls, it identifies key features that distinguish them. This spatiotemporal pattern recognition is essential for the machine to understand and replicate the insights of a professional coach, improving the analysis of basketball plays.
π Advanced Shot Analysis
The machine not only recognizes plays but also evaluates shot quality. By analyzing every shot's context, including player positions and movements, it predicts the likelihood of the shot being successful. This dual analysis separates shot quality from shooter ability, providing a more nuanced understanding of performance. For instance, it can differentiate between a player who takes difficult shots and one who makes bad shots. This advanced analysis aids teams in making informed decisions about player evaluations and strategies.
π Real-World Impact of Machine Insights
The application of machine learning extends beyond theoretical insights to practical impacts on the game. A recreated example from an NBA finals game illustrates how the machine's understanding of shot probabilities and rebound chances provides deeper insights into game-changing moments. This technology, while initially applied to professional sports, has broader implications for understanding movement in various contexts, from urban planning to personal milestones. The speaker envisions a future where spatiotemporal pattern recognition enhances how we interact with and understand our environments.
Mindmap
Keywords
π‘Moving Dots
π‘Sensors
π‘Machine Learning
π‘Pick-and-Roll
π‘Spatiotemporal Pattern Recognition
π‘Shot Quality
π‘Machine Vision
π‘Coaching Insight
π‘Data Capture
π‘Insight
Highlights
The science of moving dots involves tracking human movement in various settings, from homes to offices, and across cities and the world.
Capturing movement through sensors, videos, and apps allows for detailed tracking of human activity.
Sports, particularly basketball, provide an excellent source of data on movement, with instruments tracking players' movements in high detail.
The challenge of processing and understanding large amounts of raw data from sports, such as basketball, is addressed by machine learning.
Machines can process data at a level of detail that human eyes cannot, providing insights into complex events like basketball plays.
Machine learning enables the differentiation between complex events like basketball's pick-and-roll plays, which were previously difficult to identify.
The pick-and-roll is a prime example of a basketball play that is crucial for understanding and winning games, showcasing the importance of detailed analysis.
Machine learning's ability to understand and analyze complex basketball plays has been validated by its adoption by professional teams, including NBA champions.
Machines can now predict the quality of a basketball shot, considering factors like the player's position, the angle to the basket, and the presence of defenders.
Machine learning has improved the understanding of player performance, distinguishing between good and bad shots based on spatiotemporal features.
The technology can provide insights into critical moments of games, such as the shot that tied the game in the NBA finals, with a high level of accuracy.
The science of moving dots has practical applications beyond sports, potentially improving urban planning and the design of buildings.
Machine learning can identify and analyze human movement in various contexts, not just sports, offering a broader range of applications.
The potential of machine learning to predict and understand complex human movements could lead to significant advancements in how we live and move.
The presentation highlighted the transformative power of machine learning in analyzing and understanding human movement, with implications for various fields.
The ability to track and analyze human movement in detail has the potential to revolutionize how we perceive and interact with our environment.
The science of moving dots is a testament to the power of machine learning in extracting meaning from vast amounts of data, with far-reaching implications for society.
Transcripts
Browse More Related Video
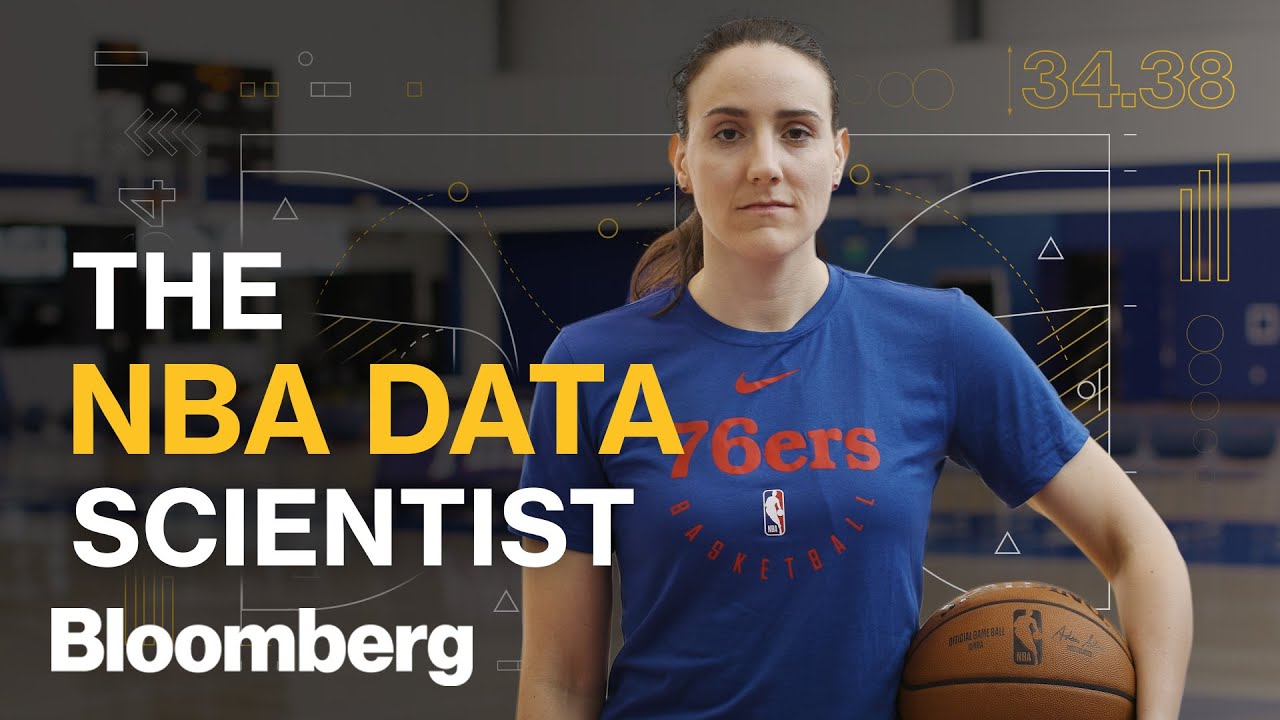
The NBA Data Scientist
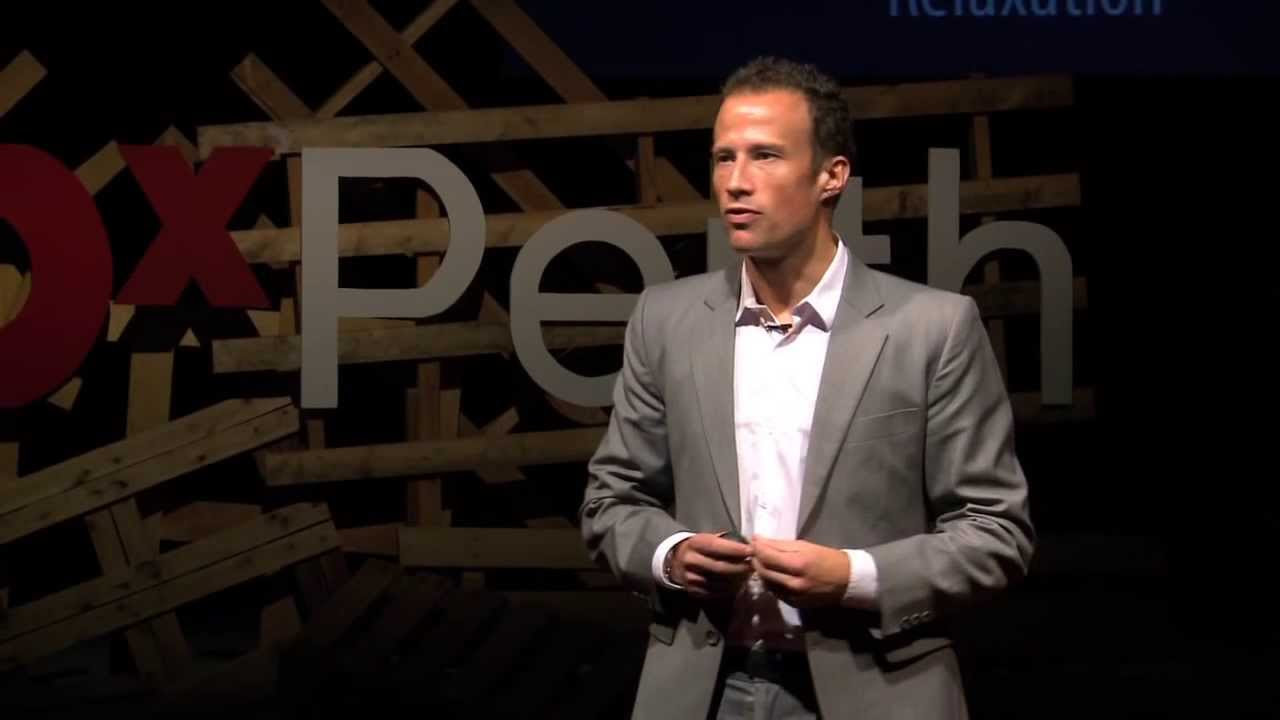
Sport psychology - inside the mind of champion athletes: Martin Hagger at TEDxPerth
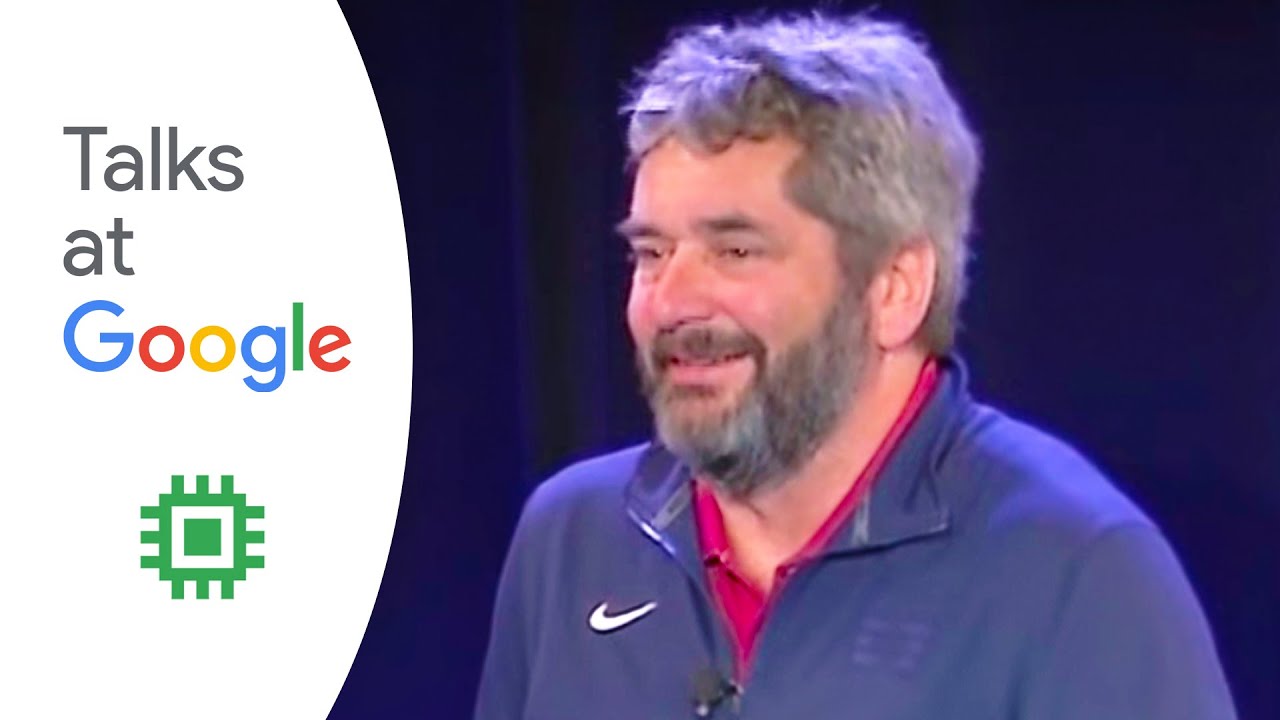
Sabermetrics 101: Baseball Analytics | Andy Andres | Talks at Google
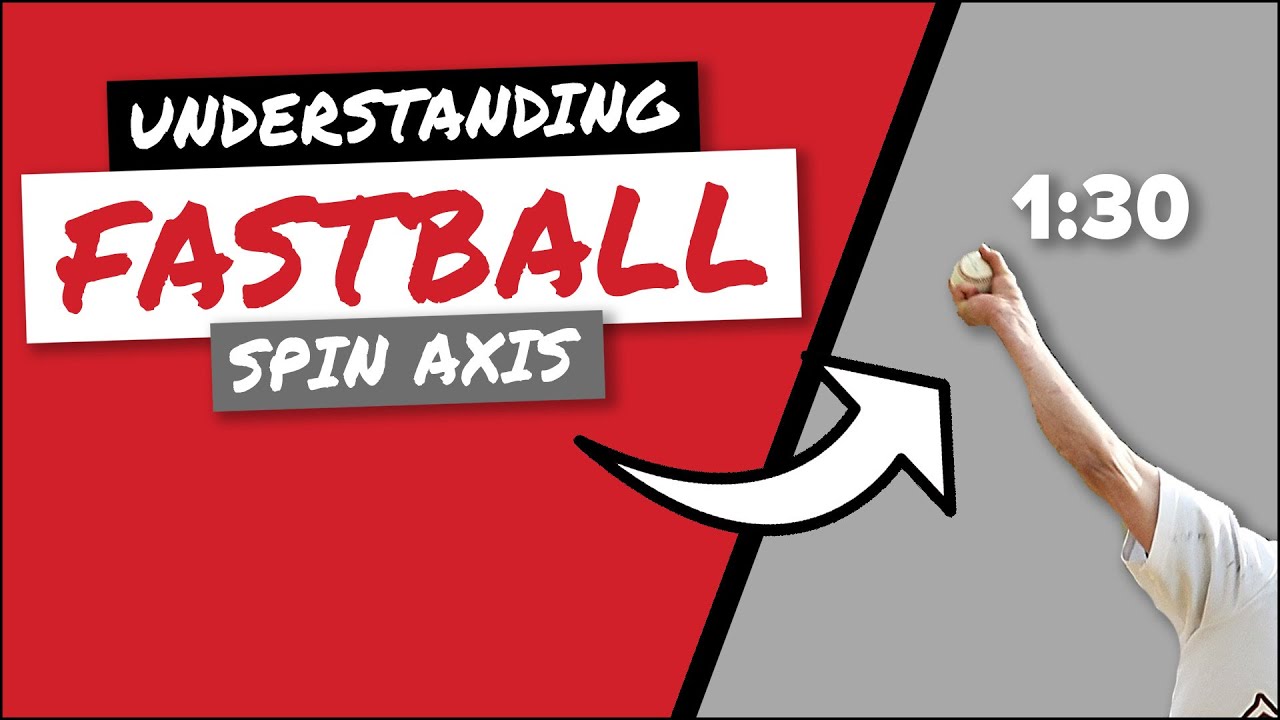
UNDERSTANDING FASTBALL SPIN AXIS

What is Calculus Used For? | Jeff Heys | TEDxBozeman
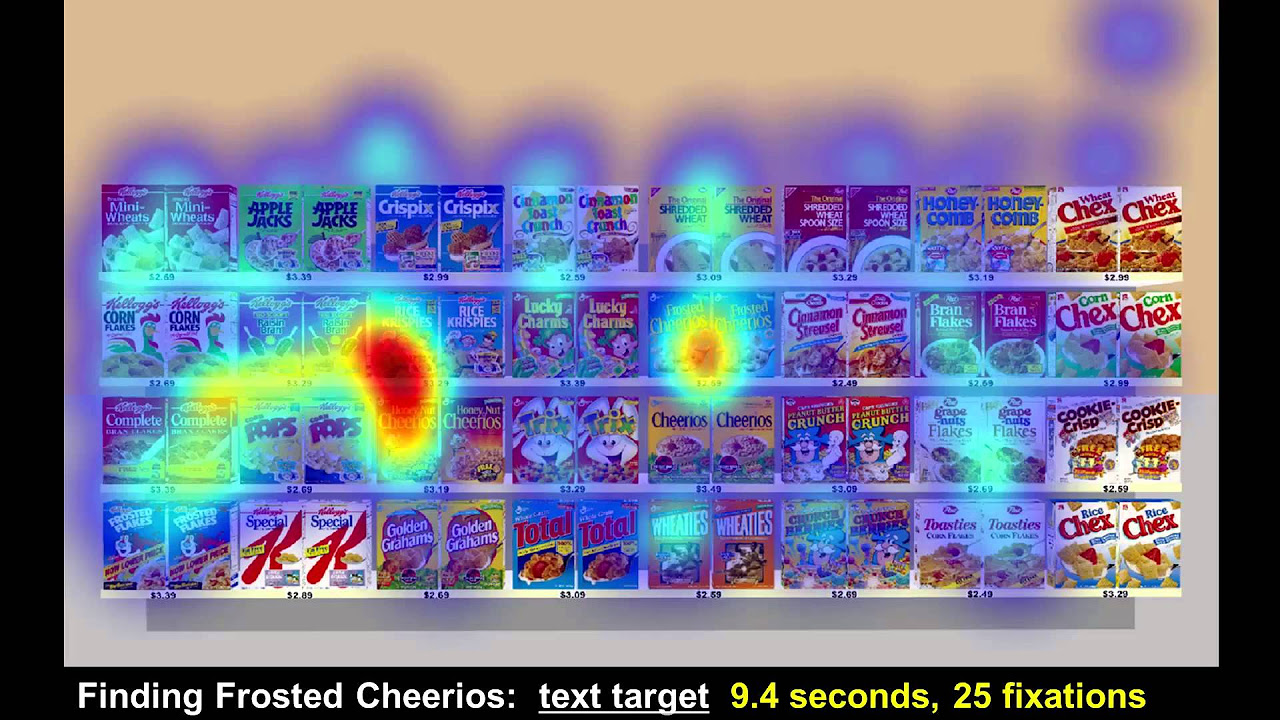
How stores track your shopping behavior | Ray Burke | TEDxIndianapolis
5.0 / 5 (0 votes)
Thanks for rating: